文献来源:, Zhihua Zhou a, ,
文章摘要:Heating load predictions serve as one of the fundamental tasks in heating operation management. Many studies have used data-driven methods to build prediction models, and the quantity and quality of training data are key factors affecting the model performance. However, for some special cases, such as new heating substation and the end period of heating with different load characteristics, sufficient and high-quality data cannot be provided for model training, resulting in low accuracy of the model. In this paper, TimeGAN is applied in the heating field for the first time to augment the data and improve the prediction accuracy of the model. Results show that the prediction error reduces by 50% and CV-RMSE can reach 0.0405 after using TimeGAN in the early period of heating, and the accuracy is highest when the synthetic data are three times of original data. For the mid and end period of heating, the prediction errors can also be reduced by 3%–8% compared with training on original data, and the data amount reaches 15,000–30000, the performance of the model reaches the best
/TimeGAN_cjy.pdf
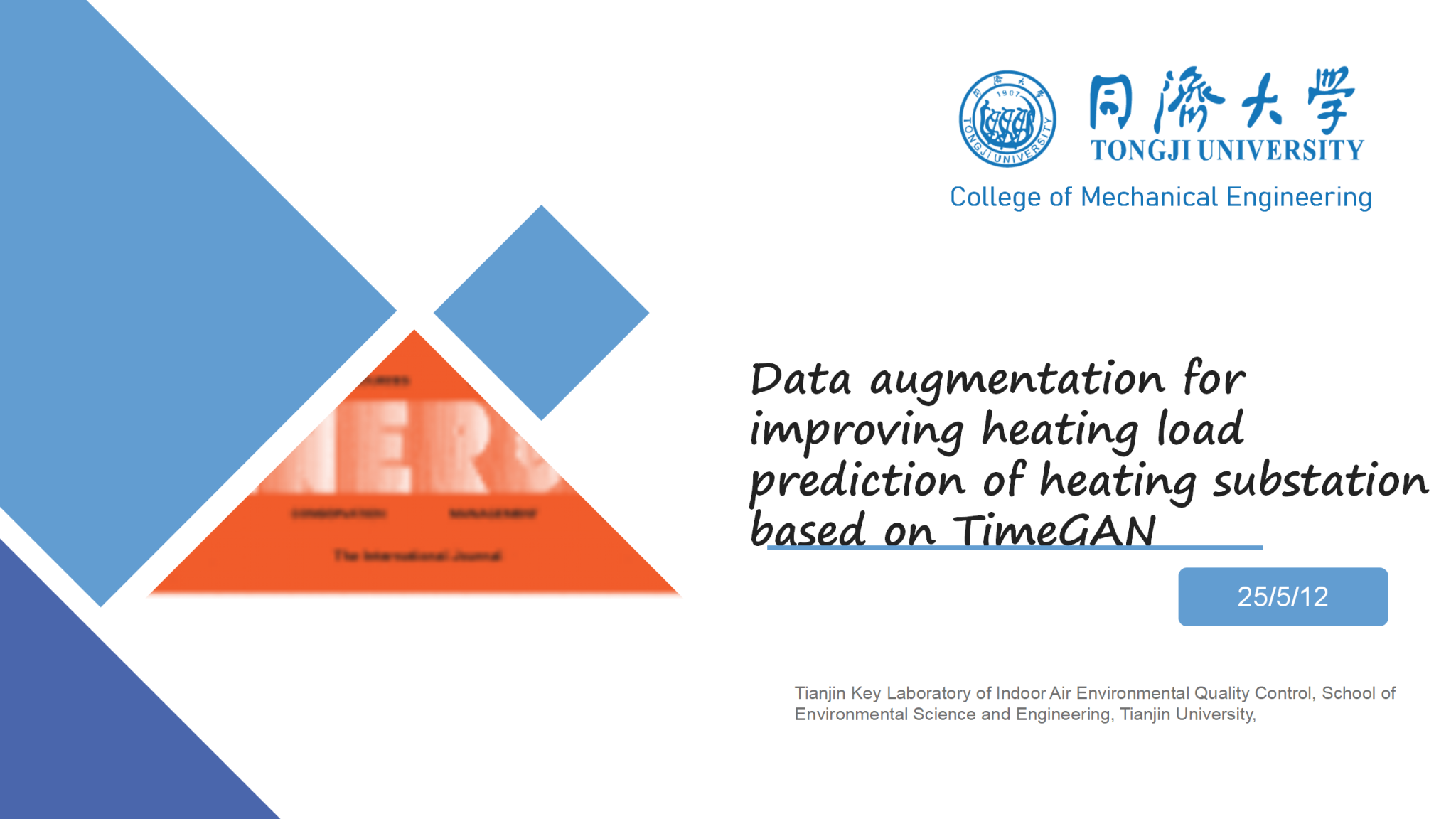
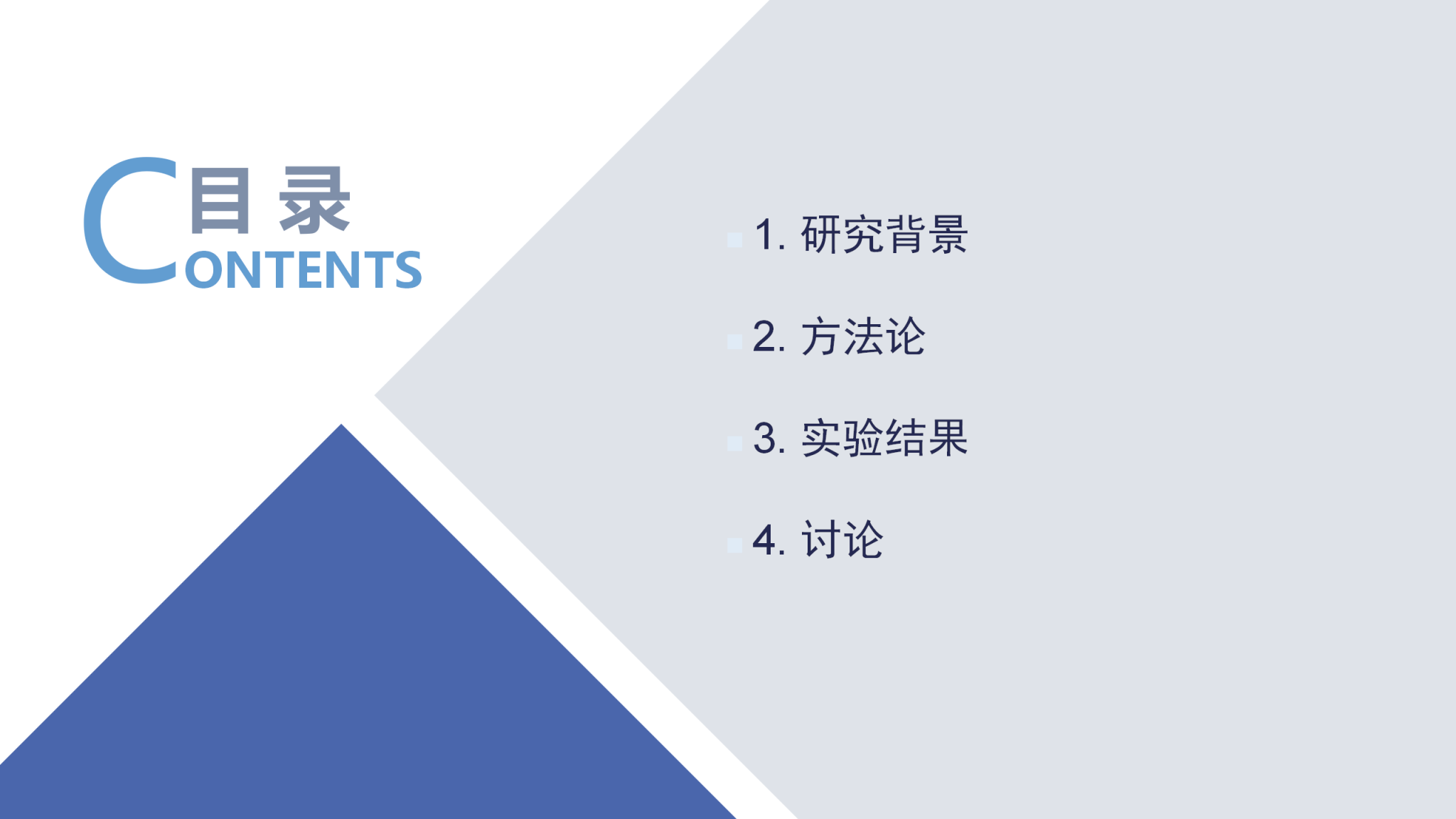
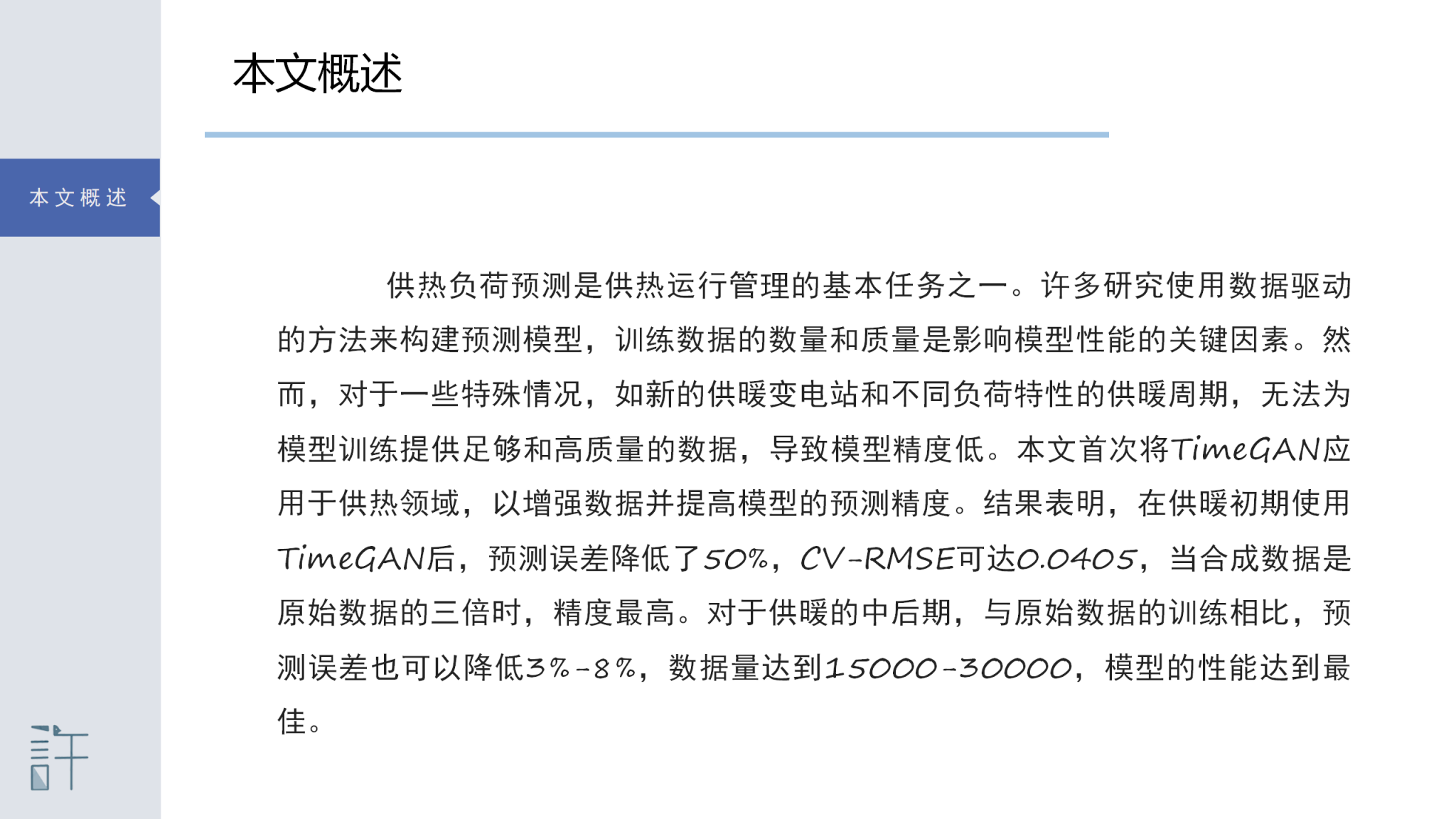
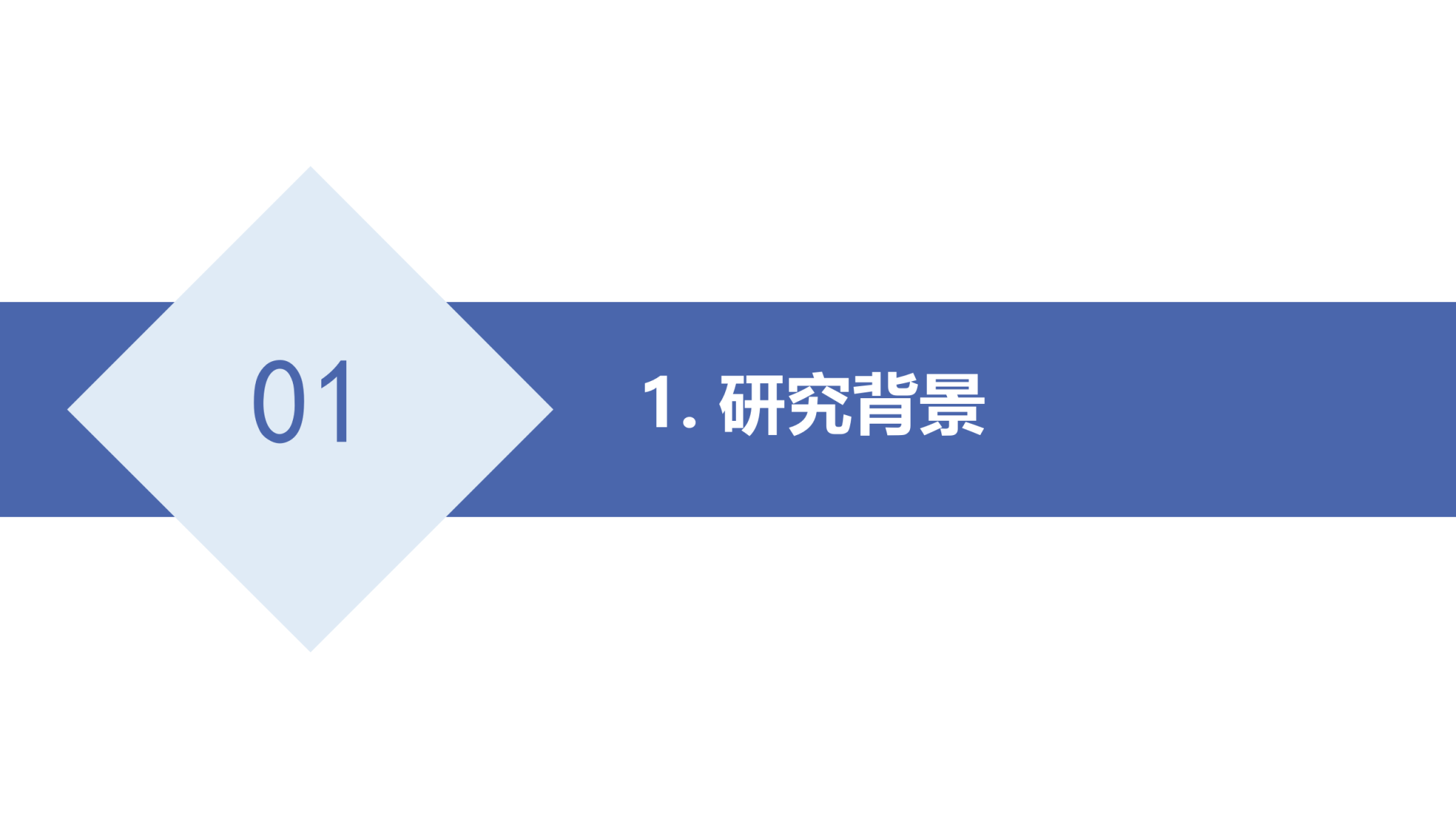
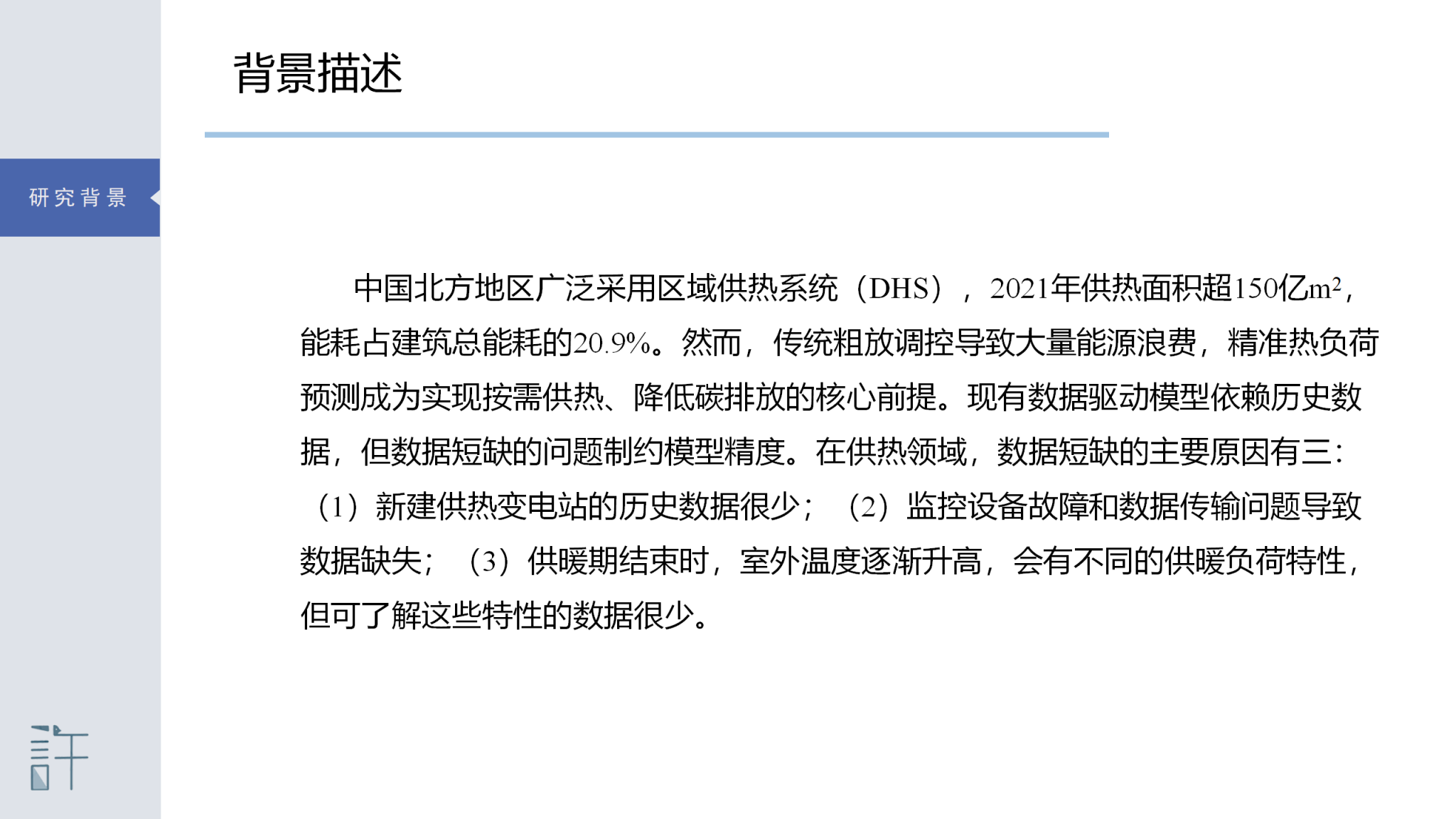
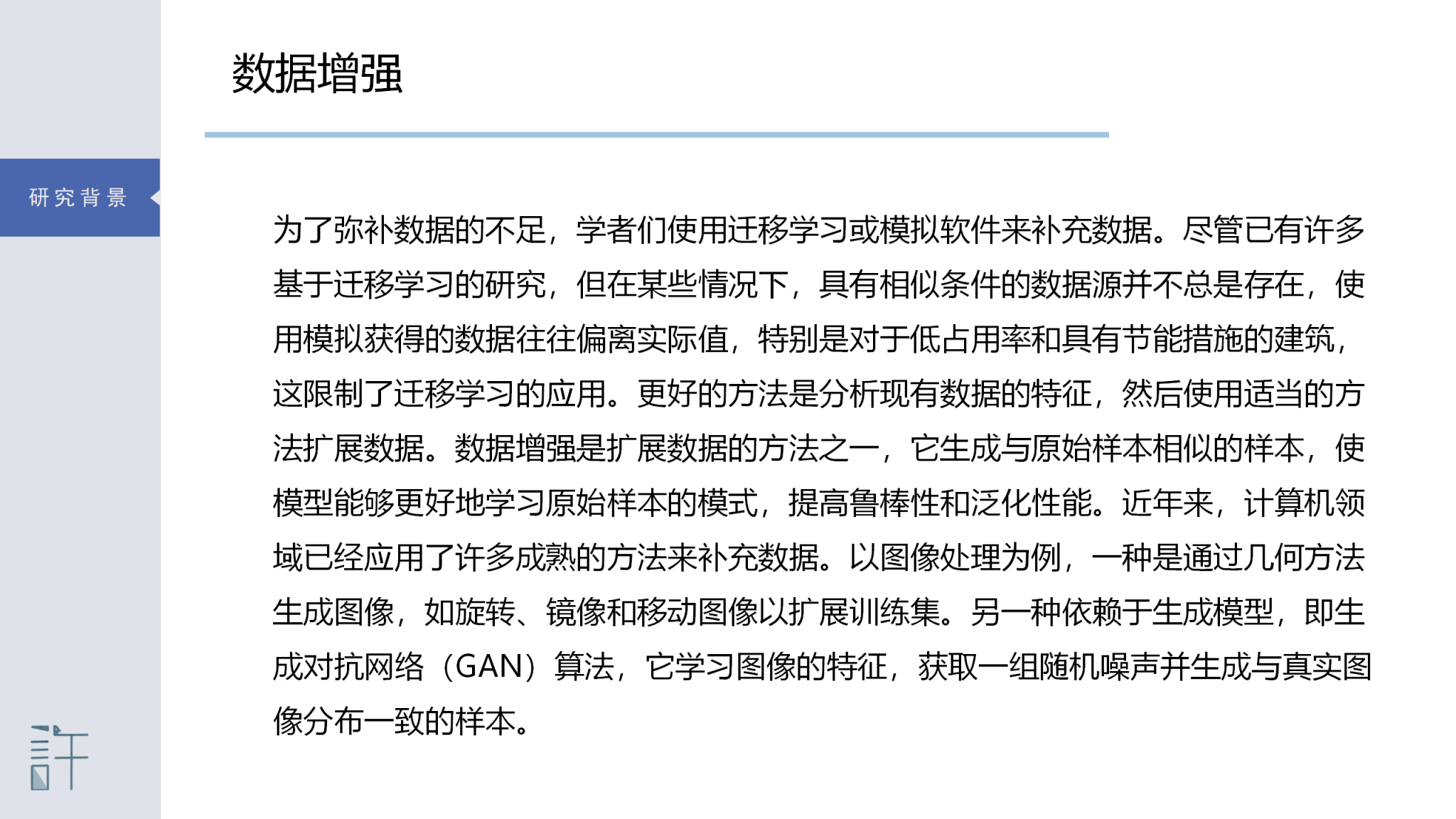
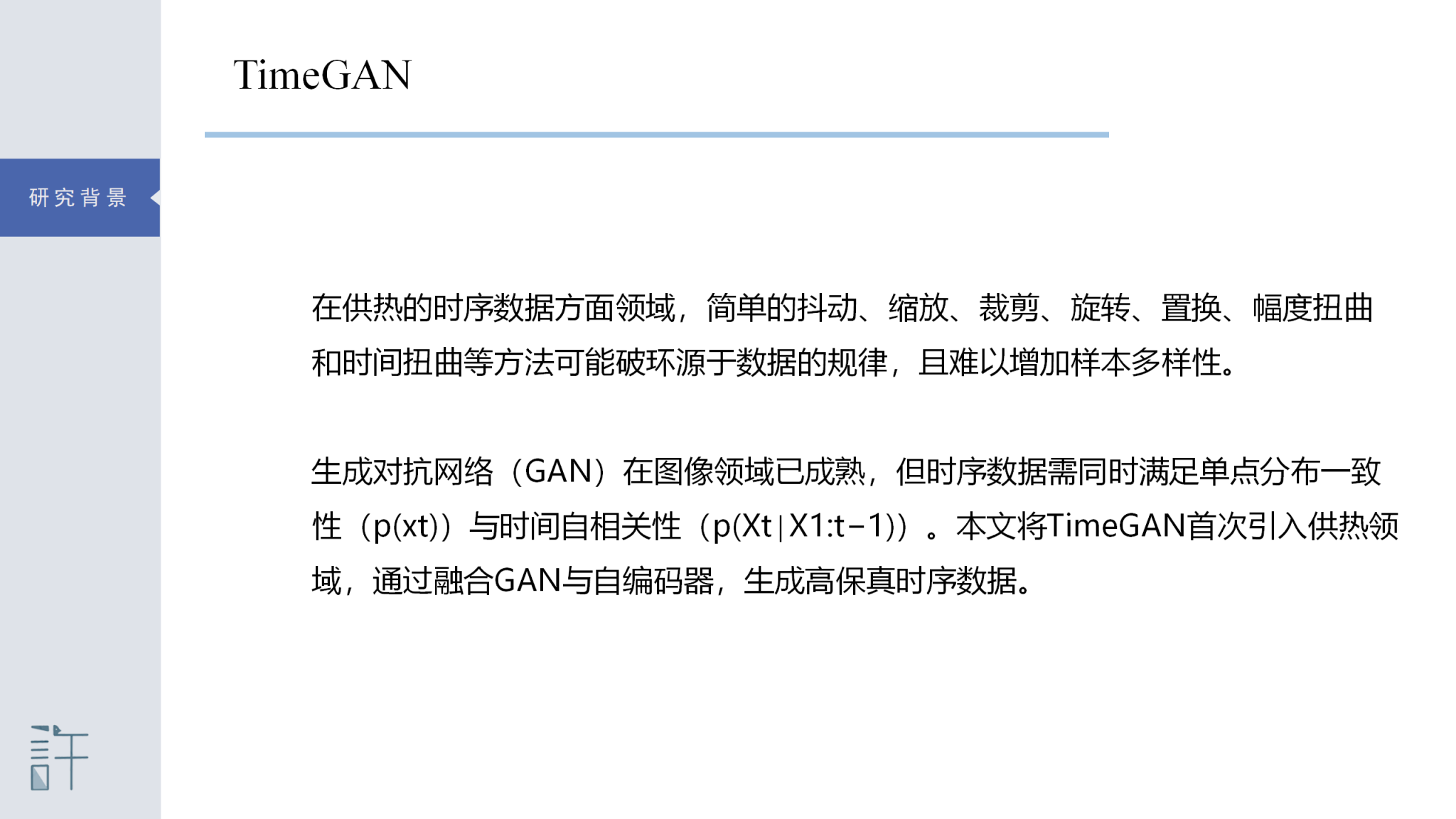
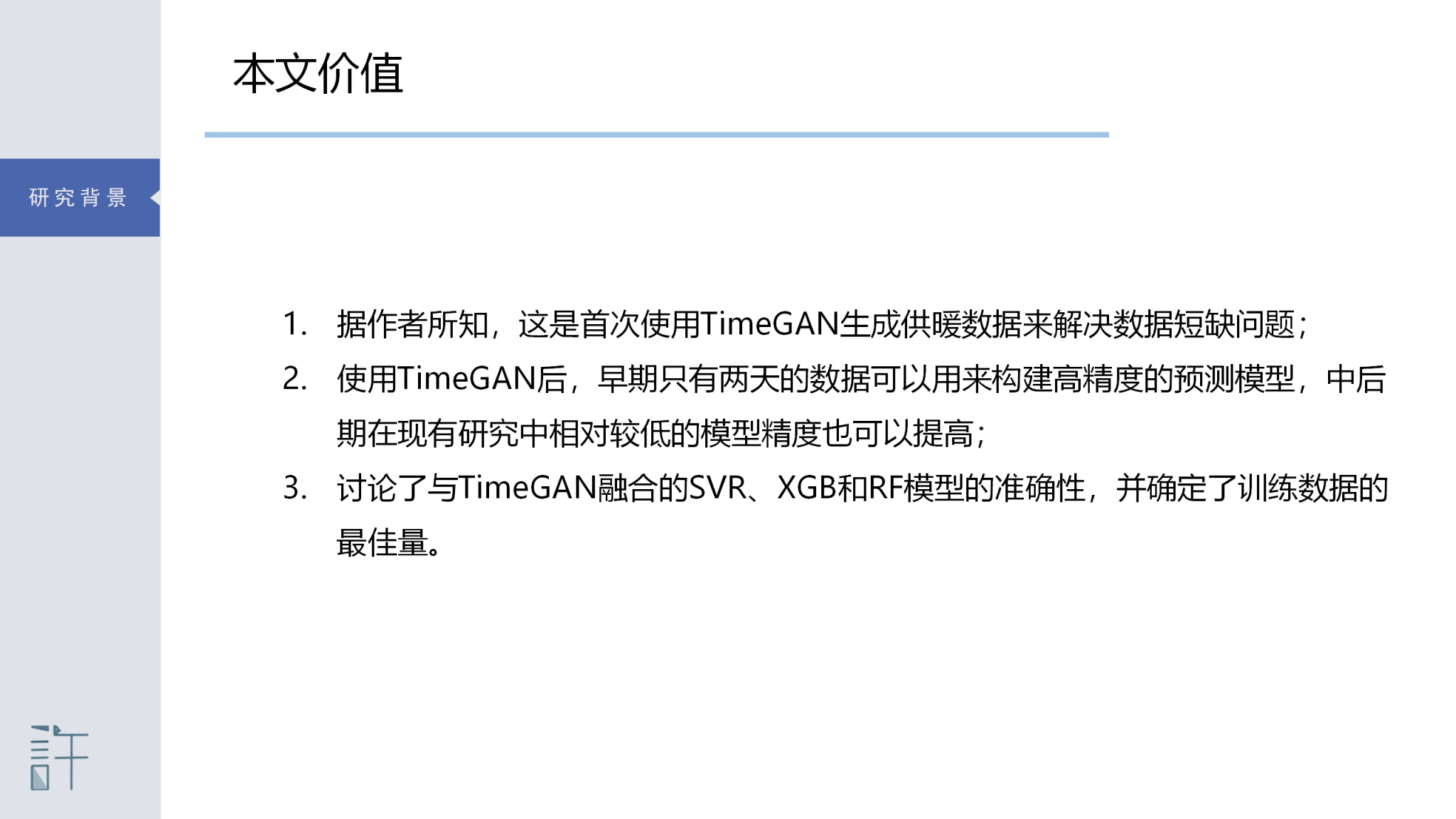
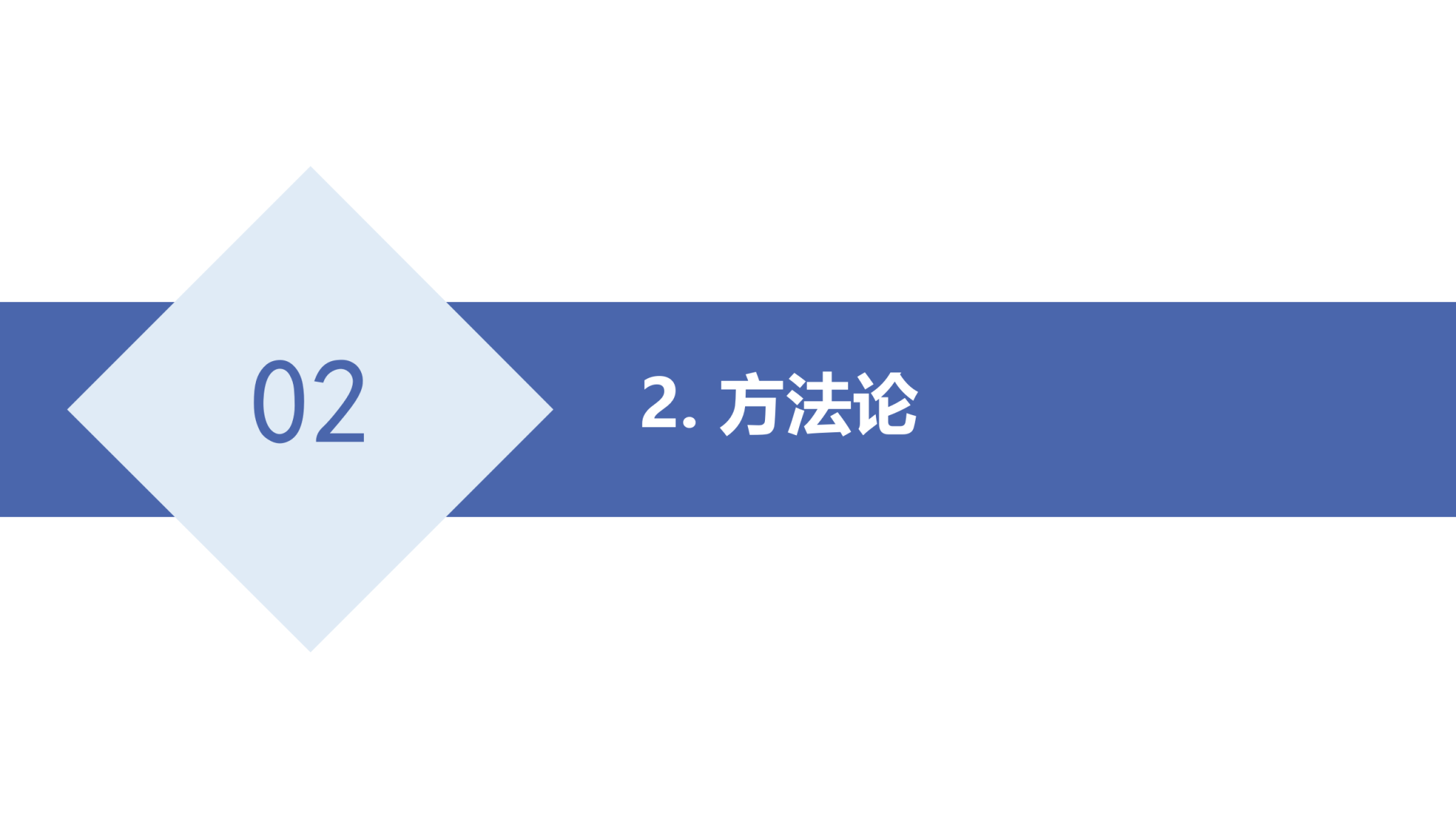
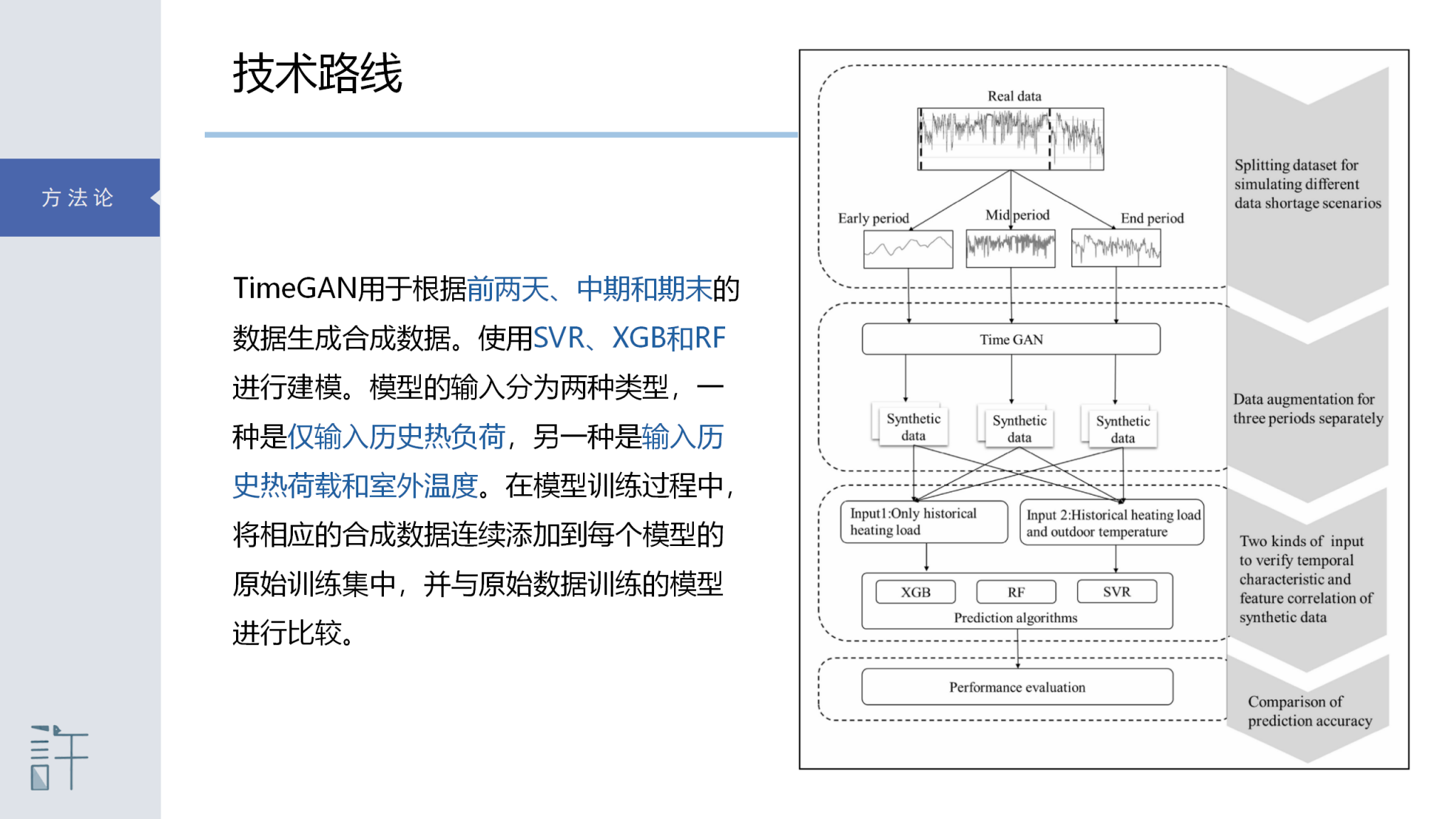
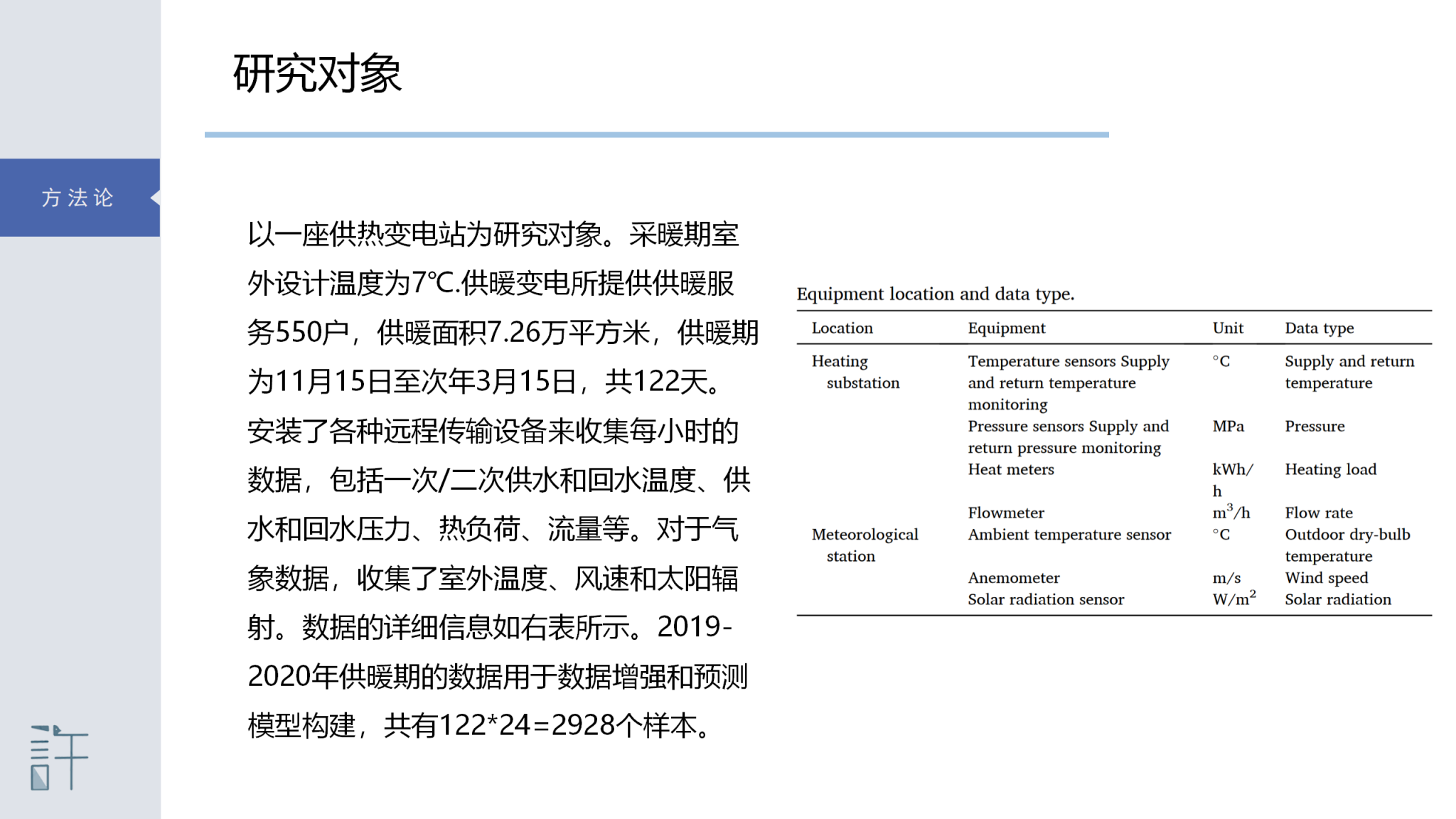
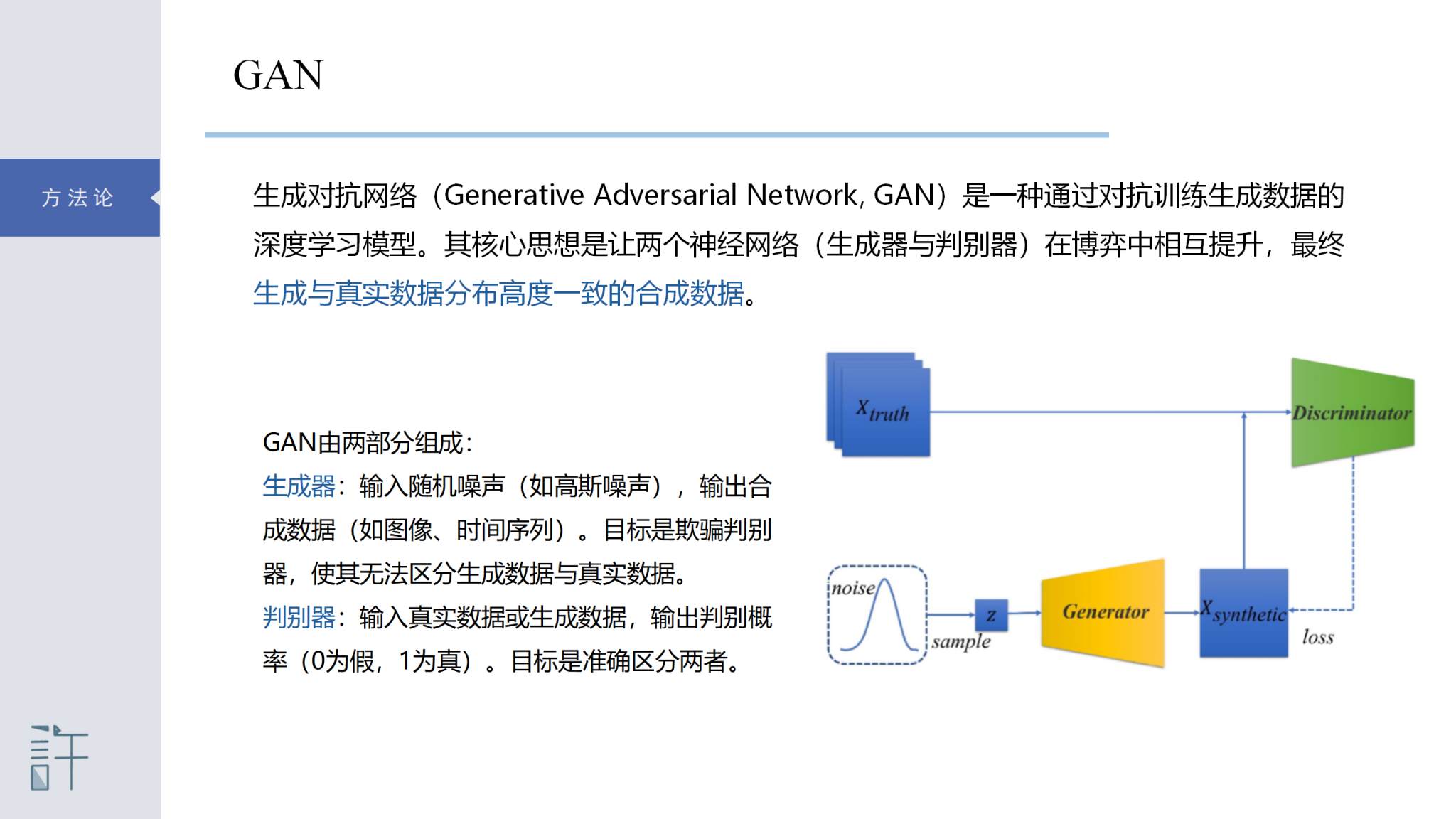
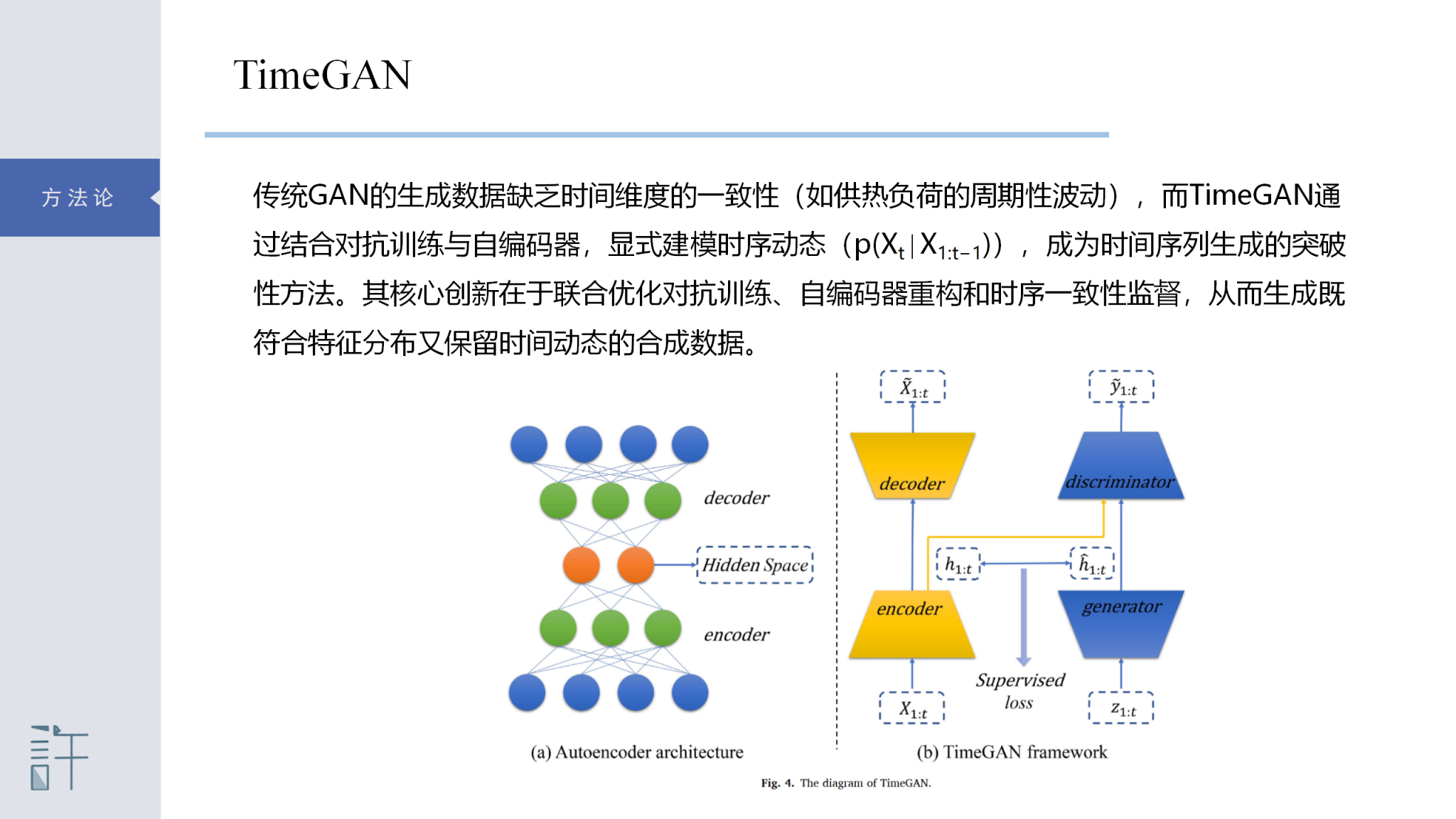
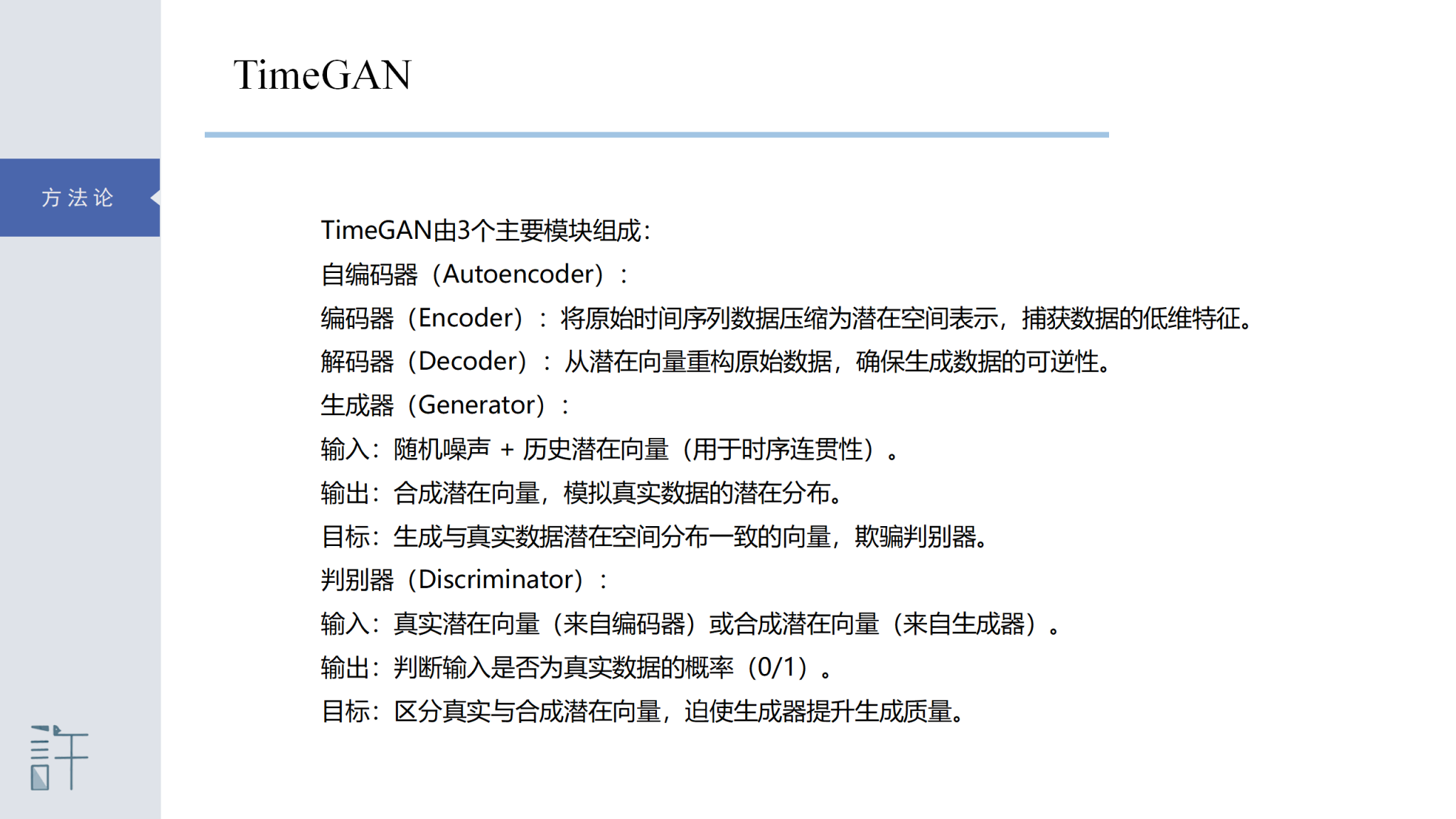
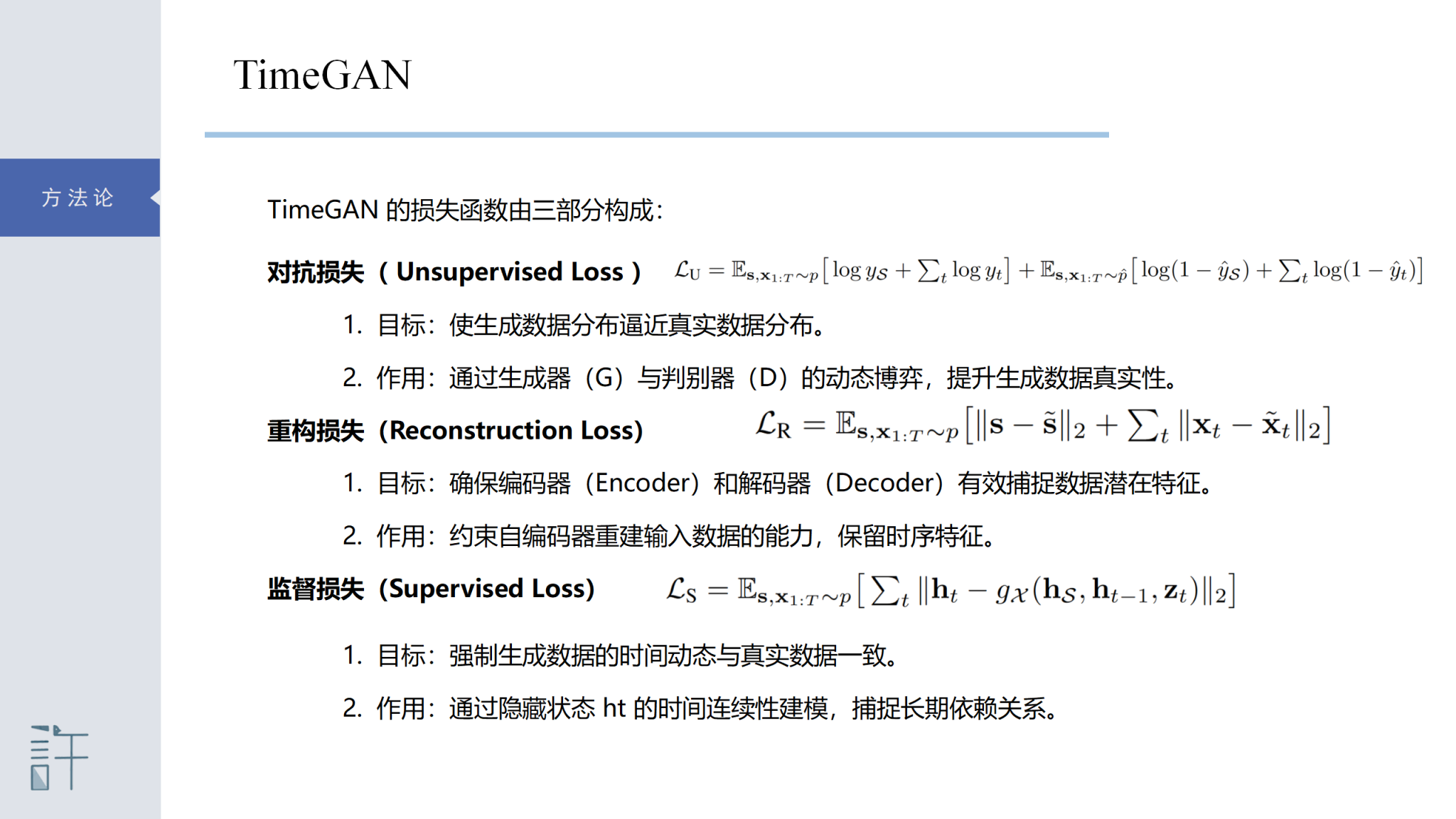
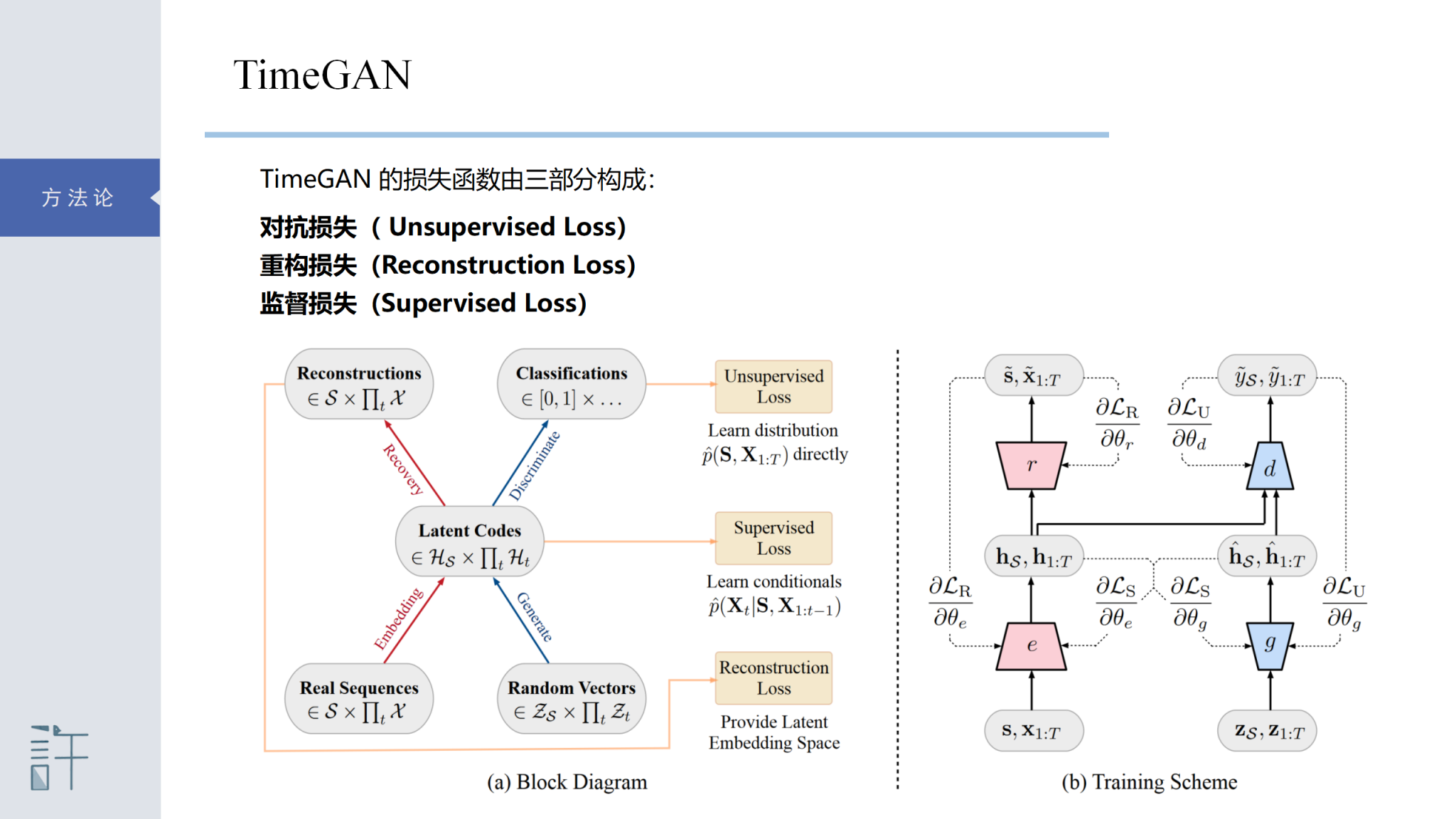
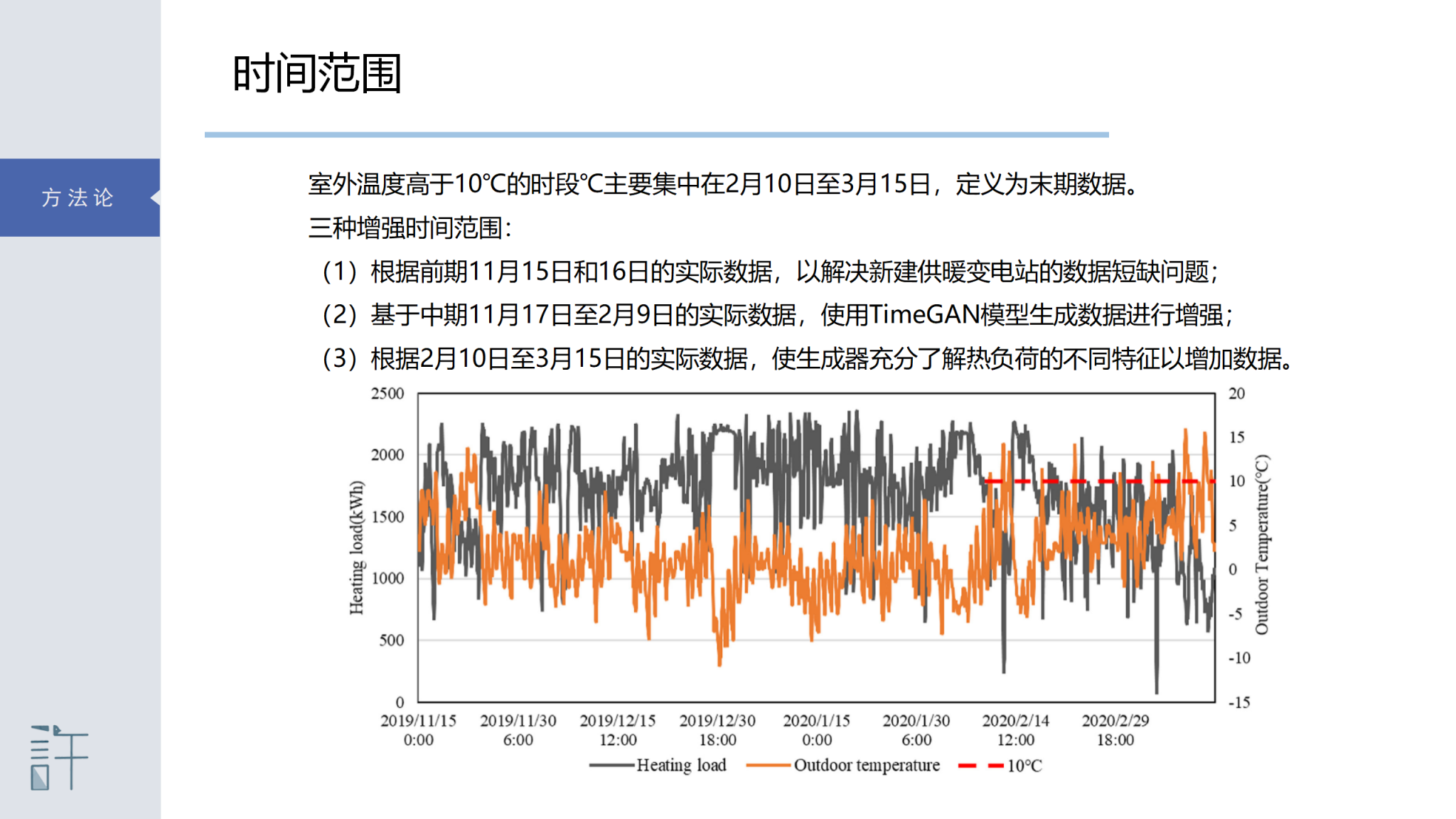
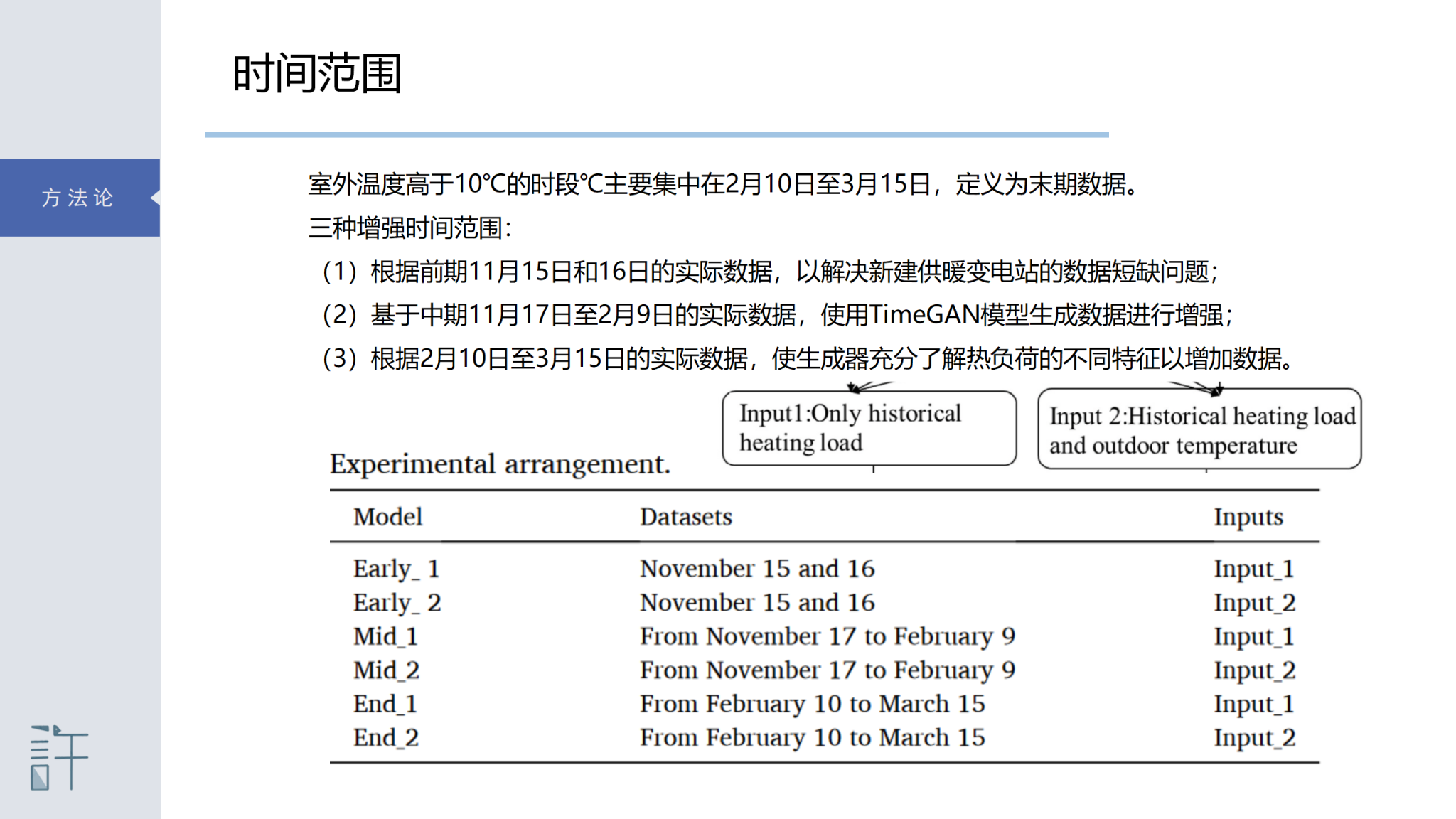
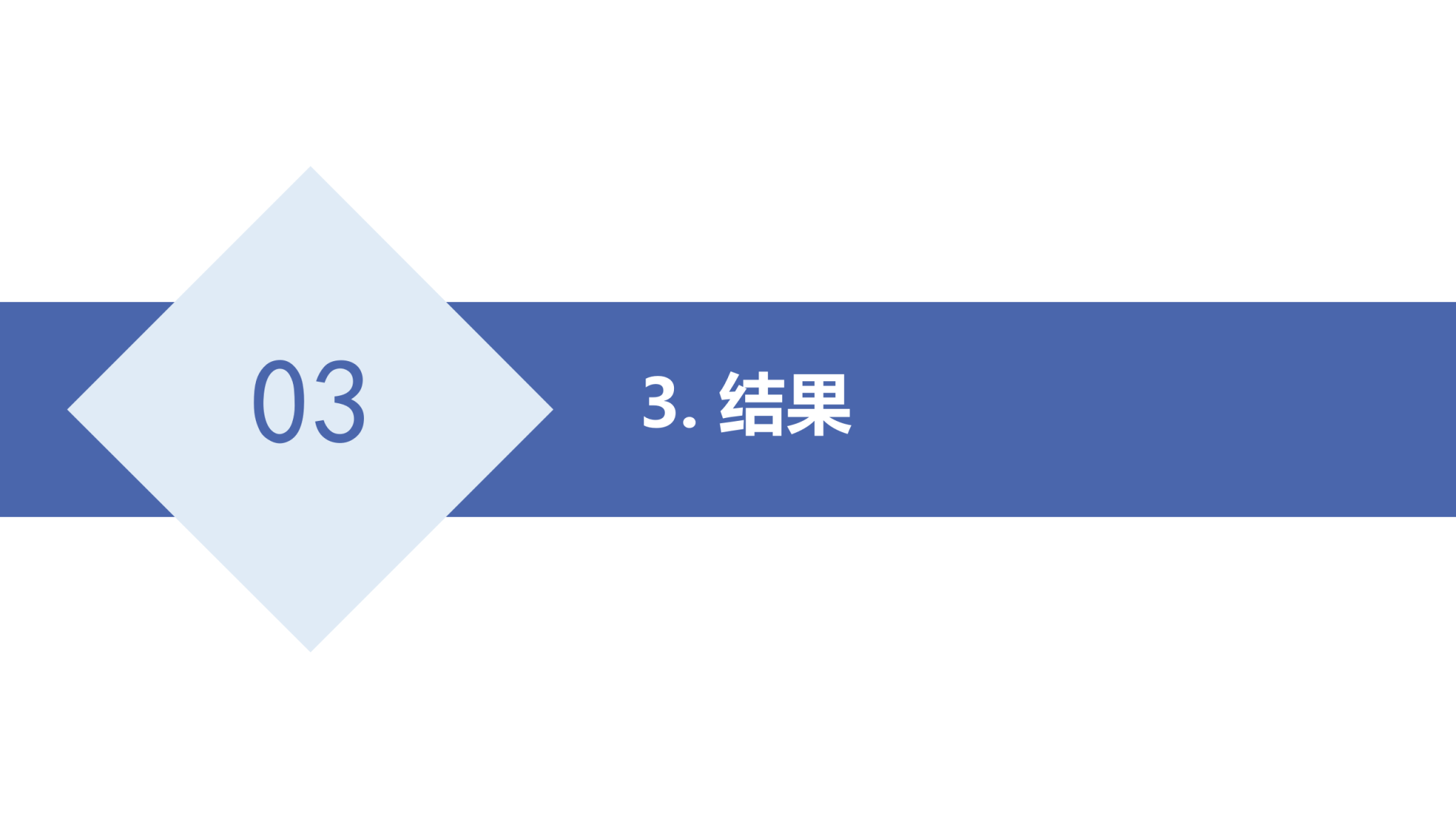
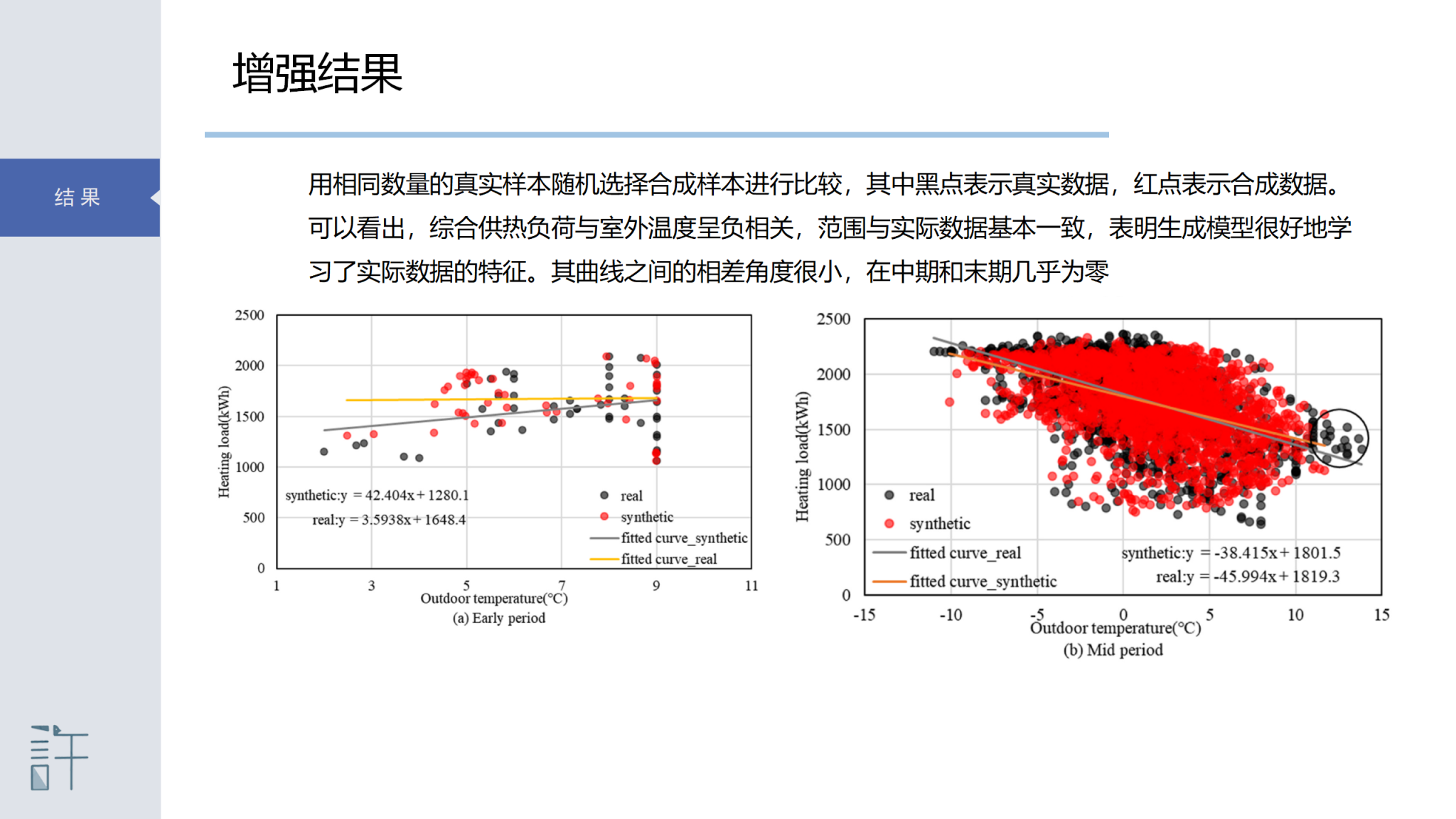
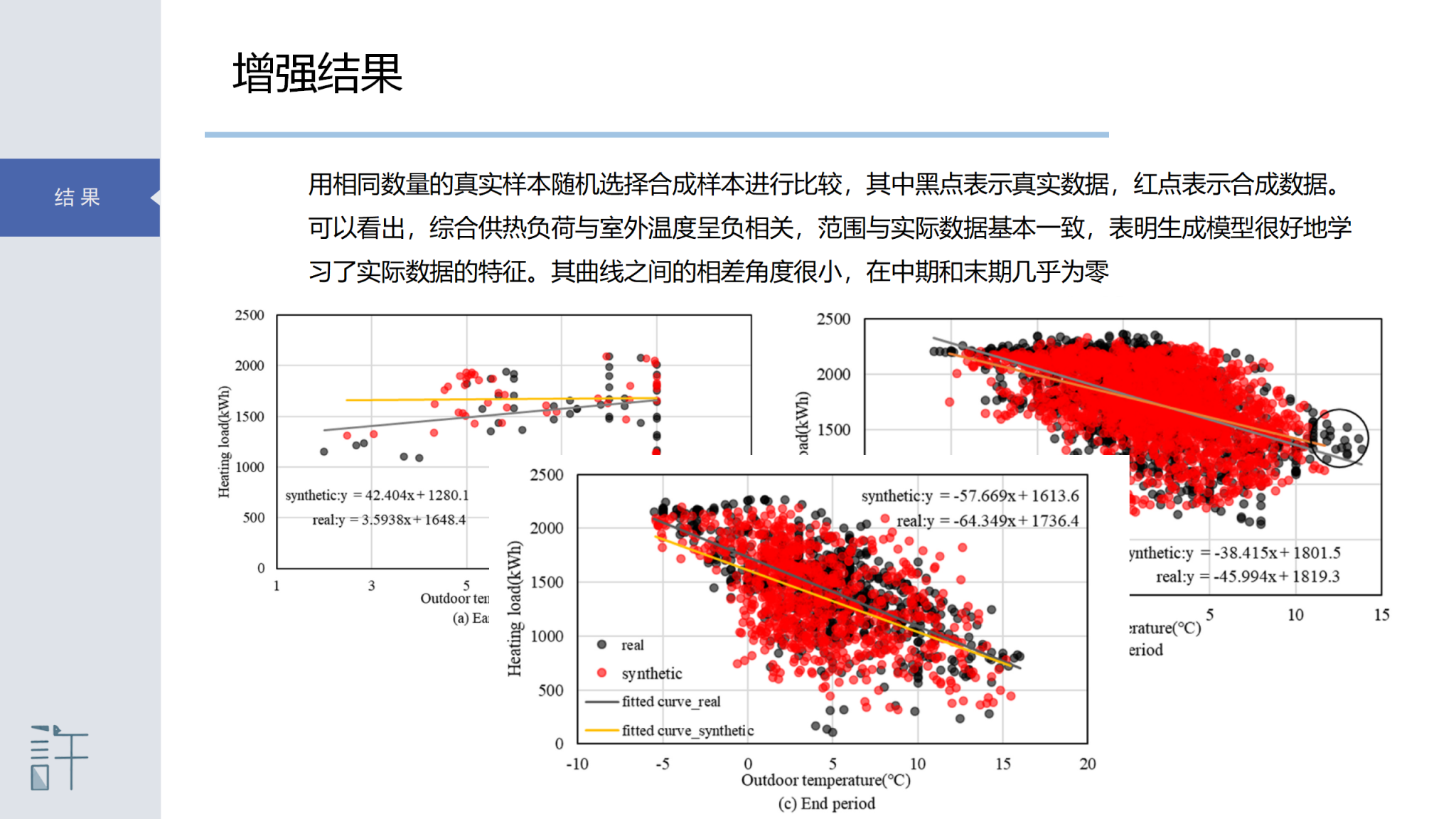
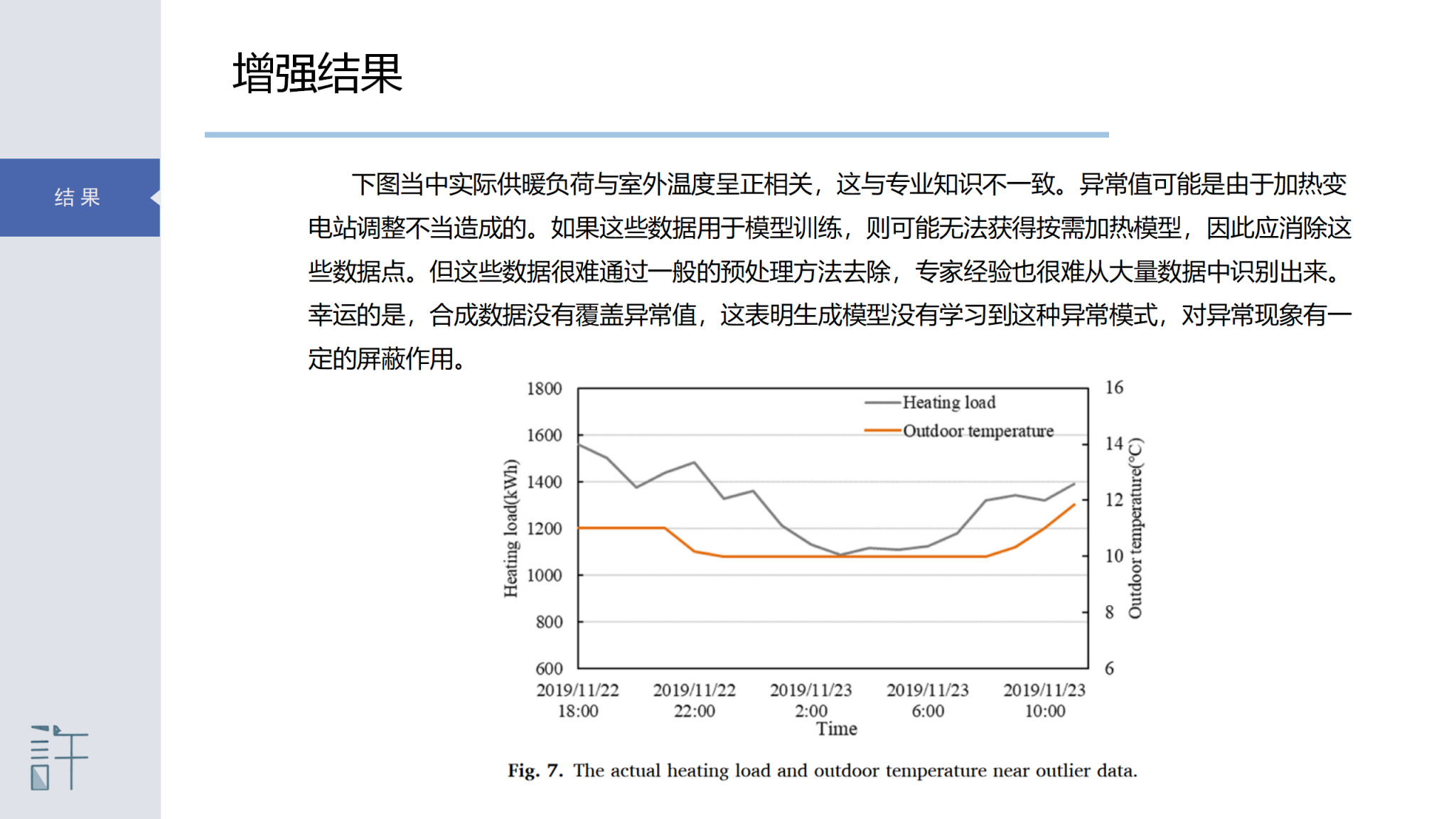
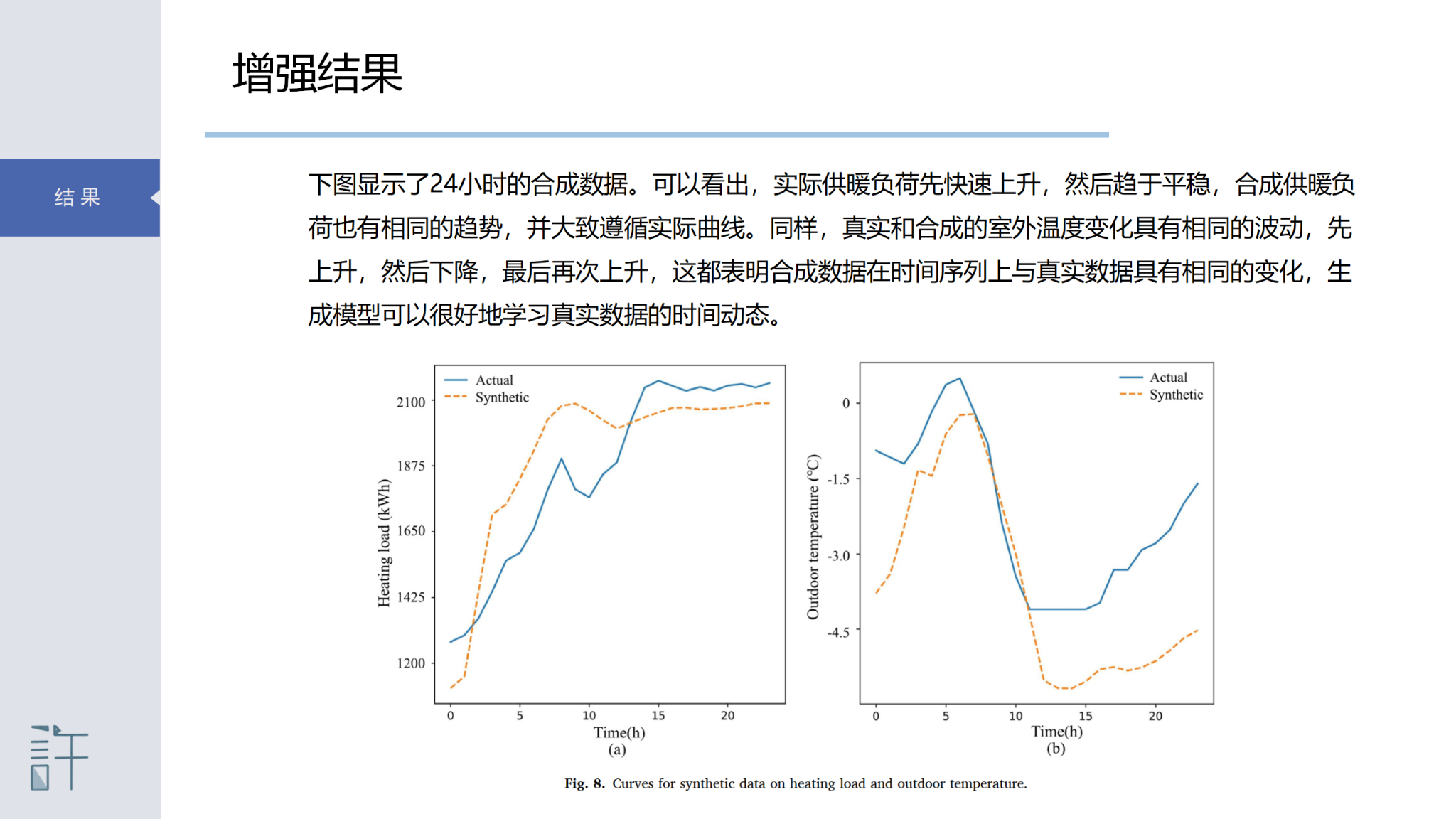
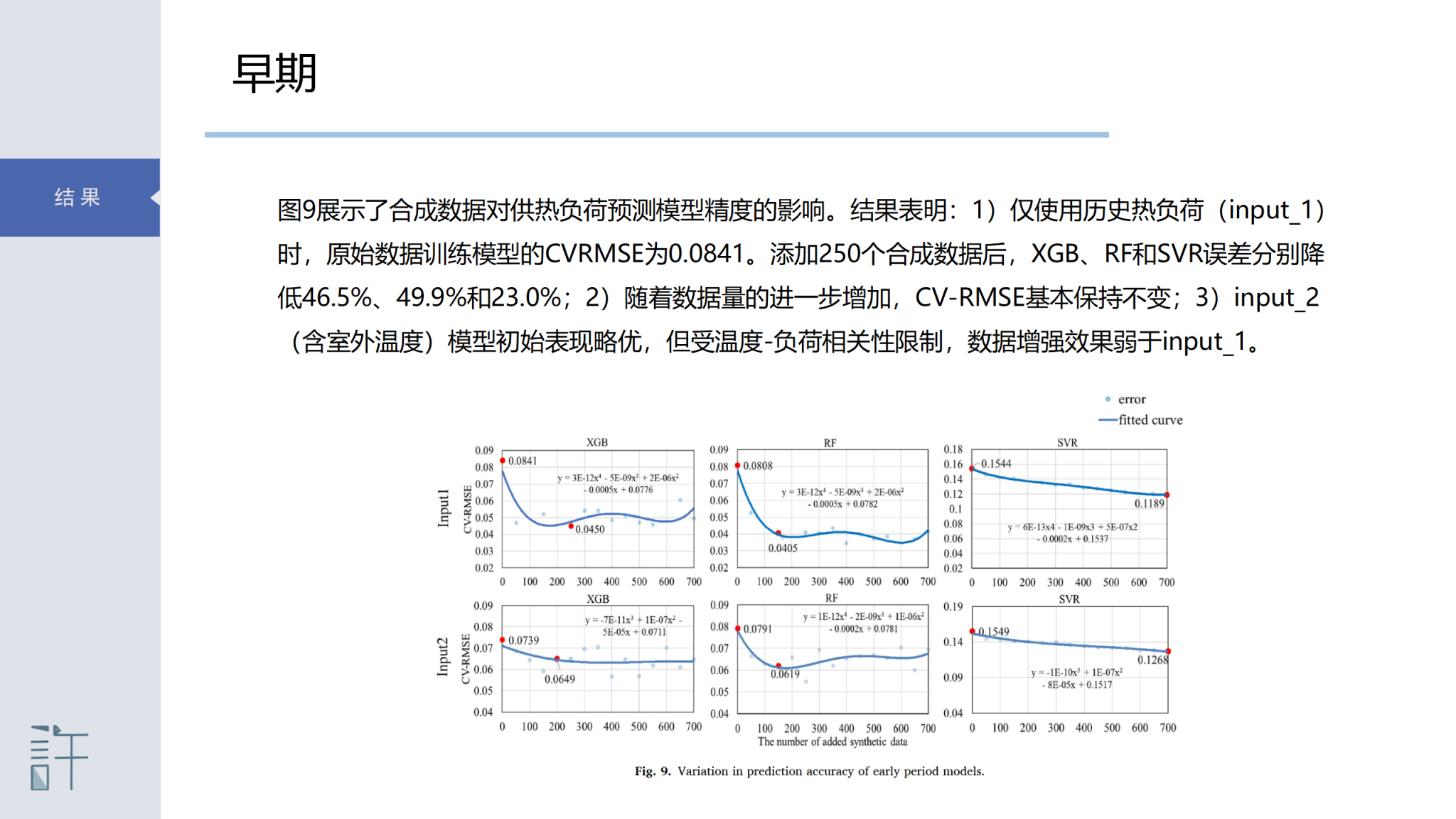
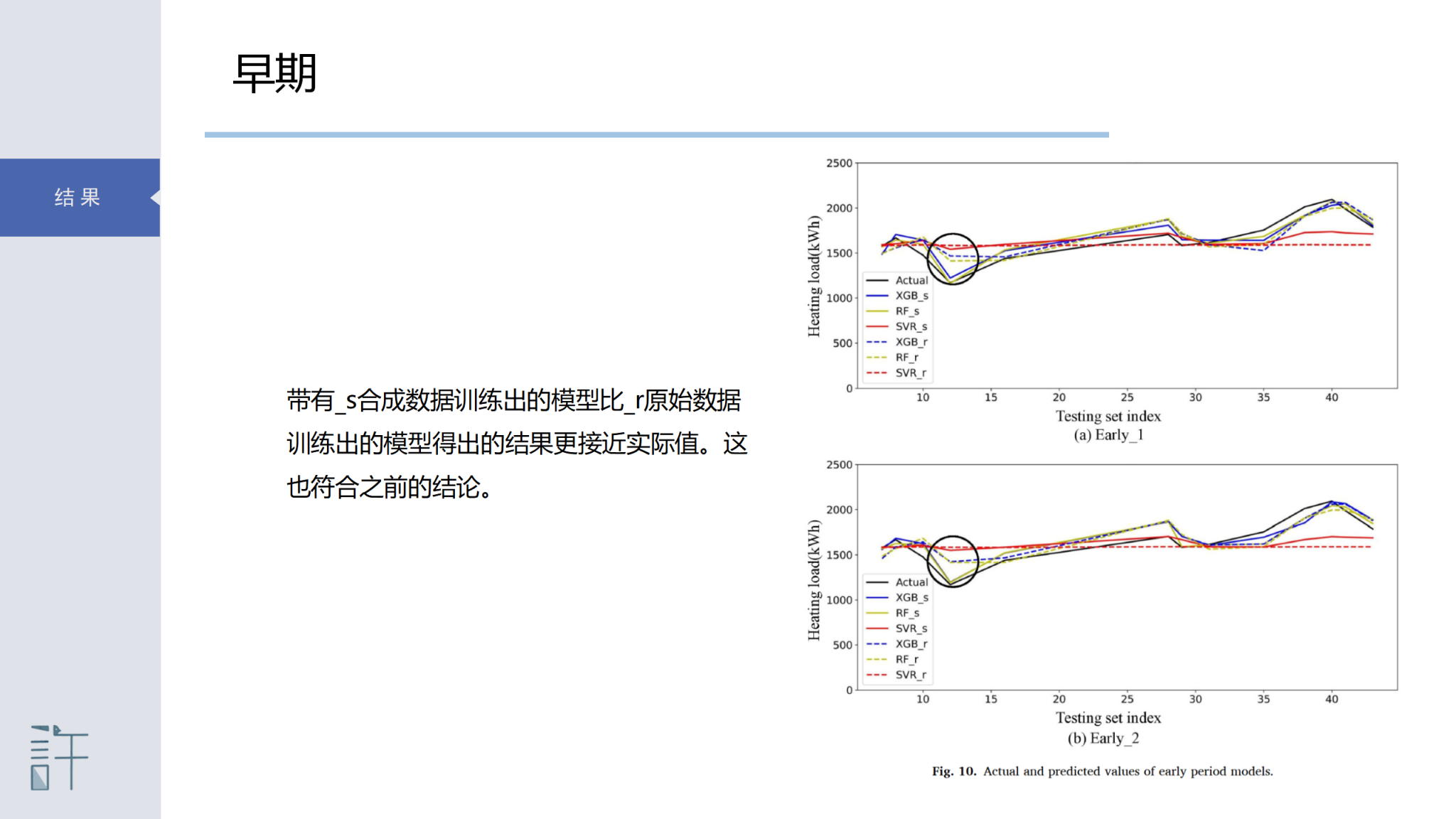
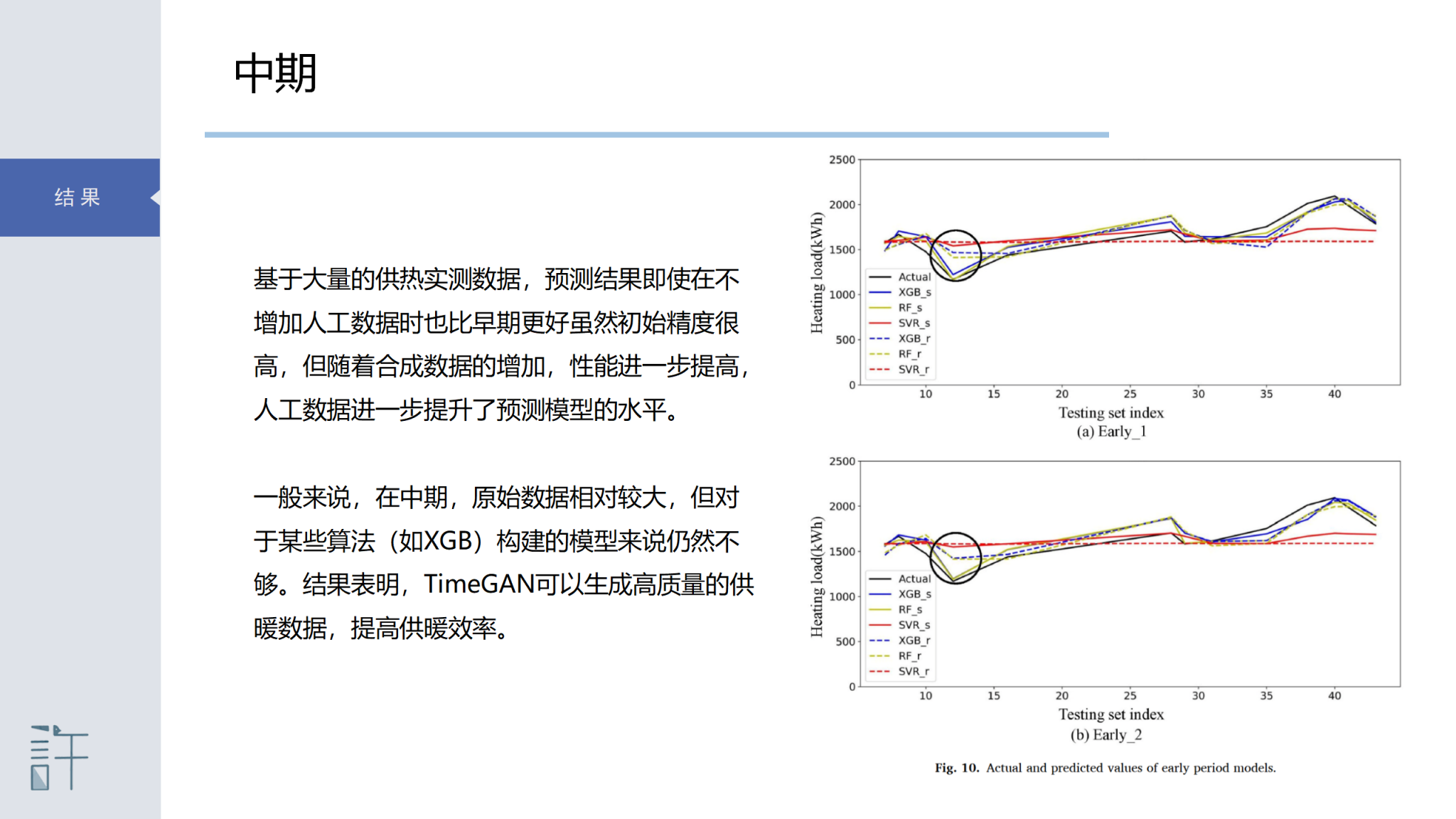
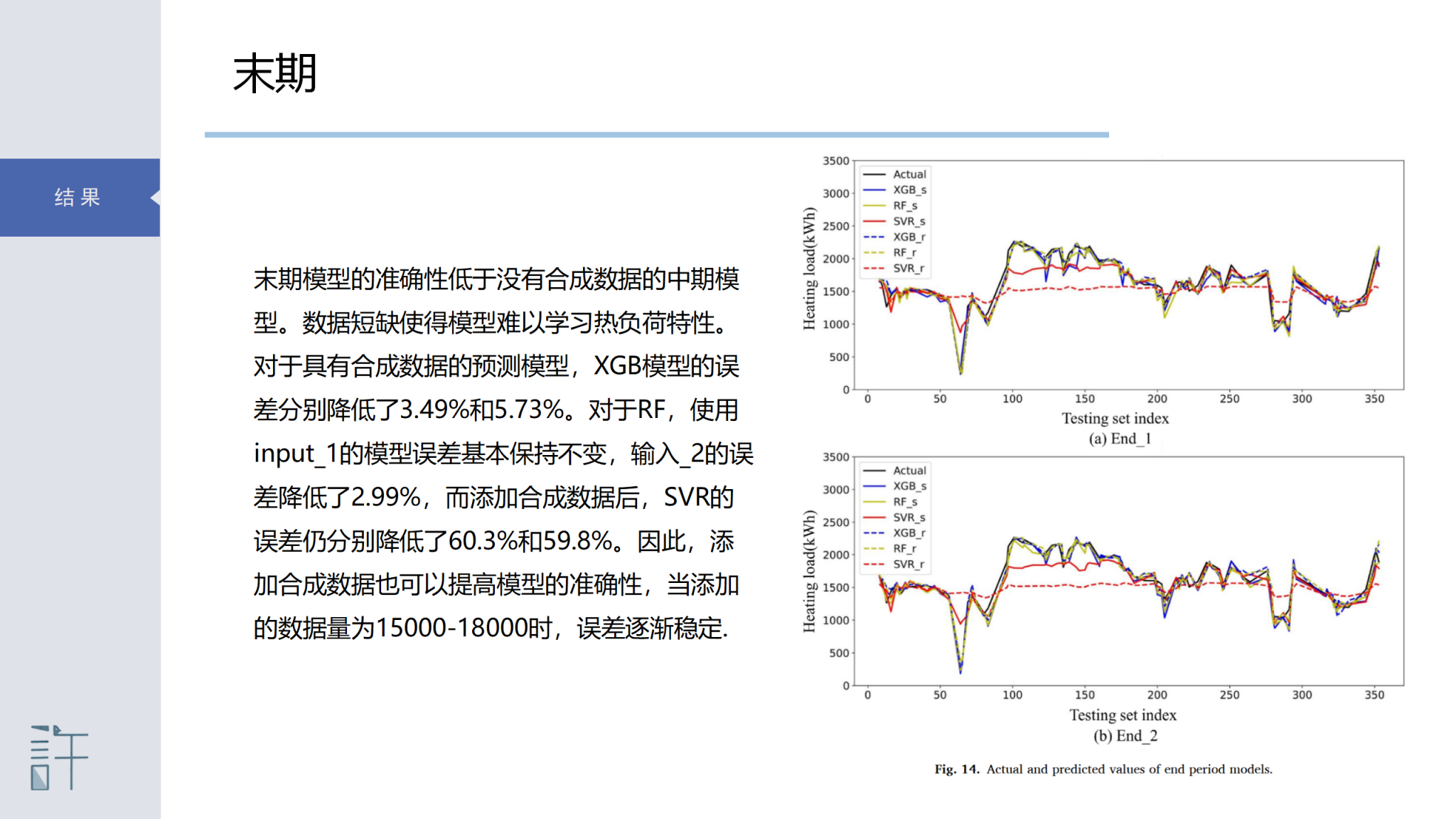
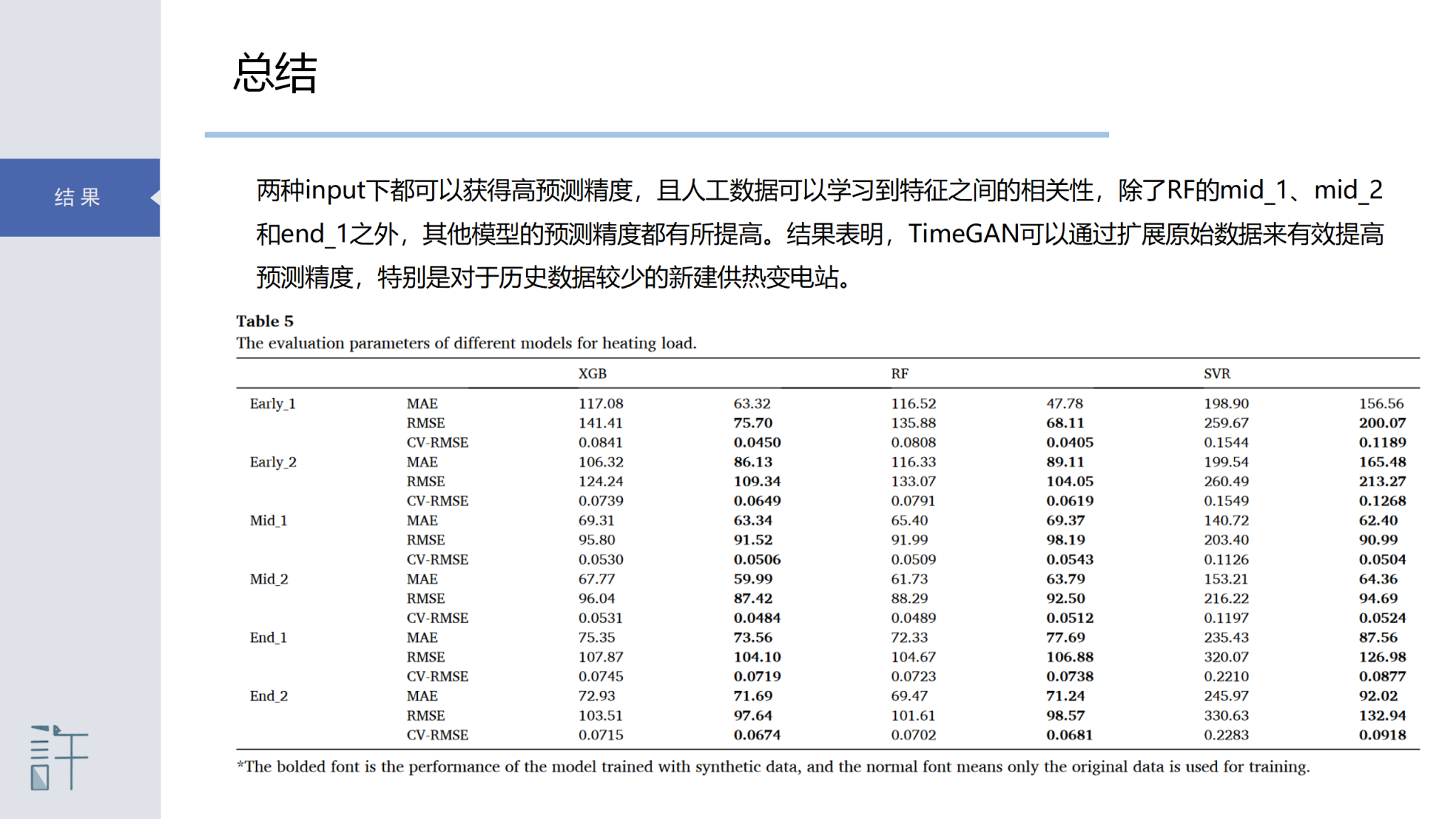
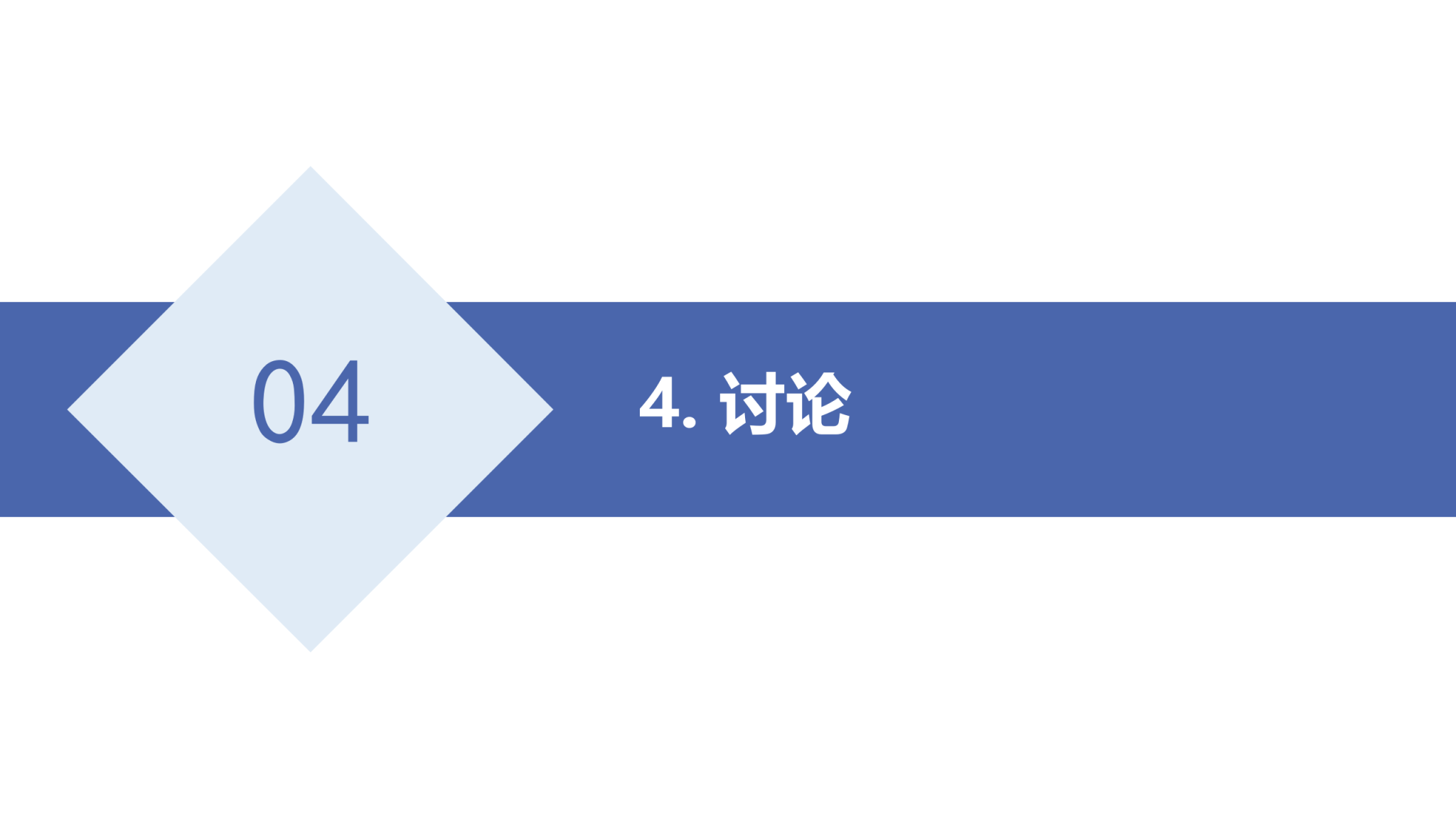
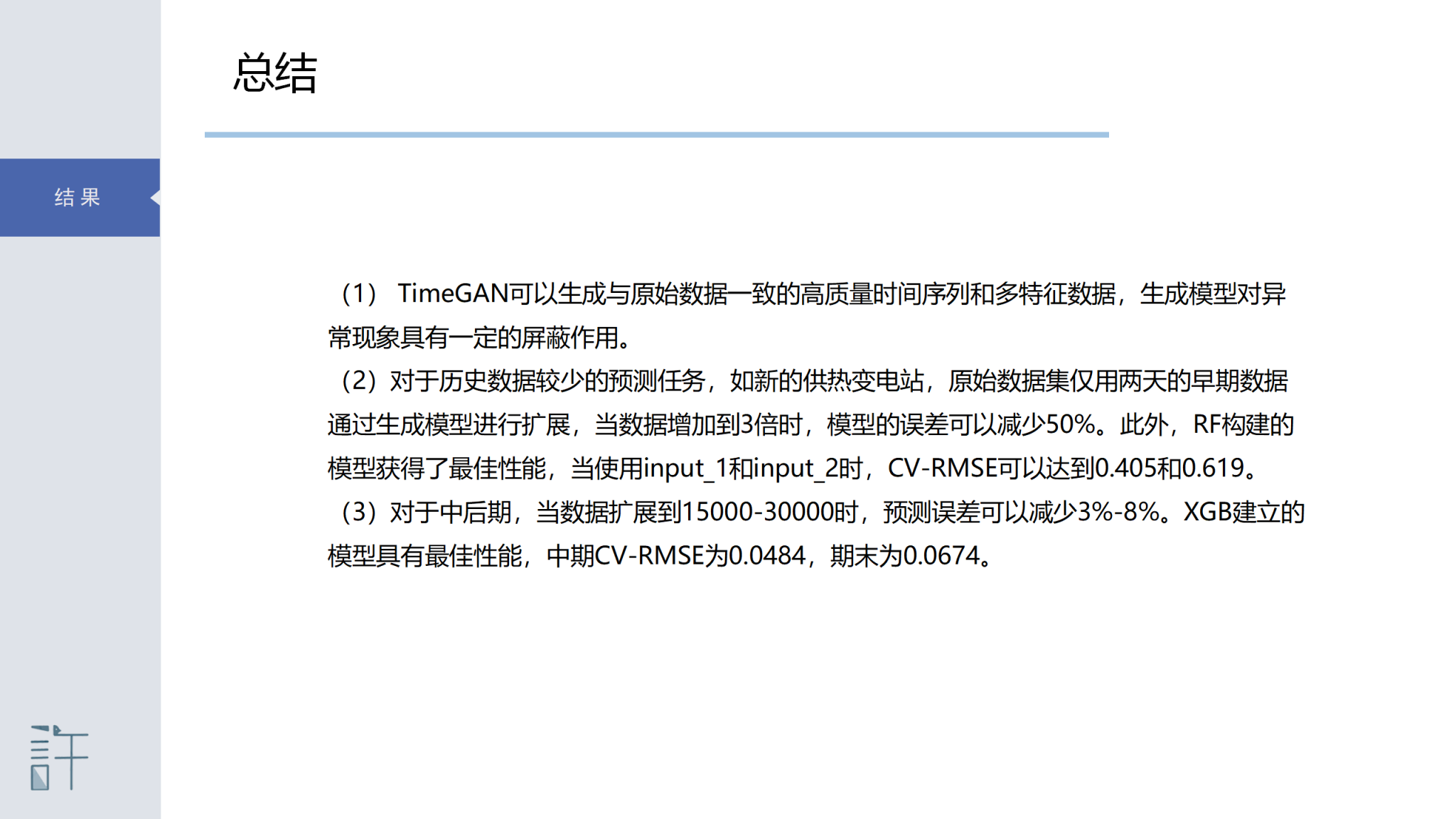
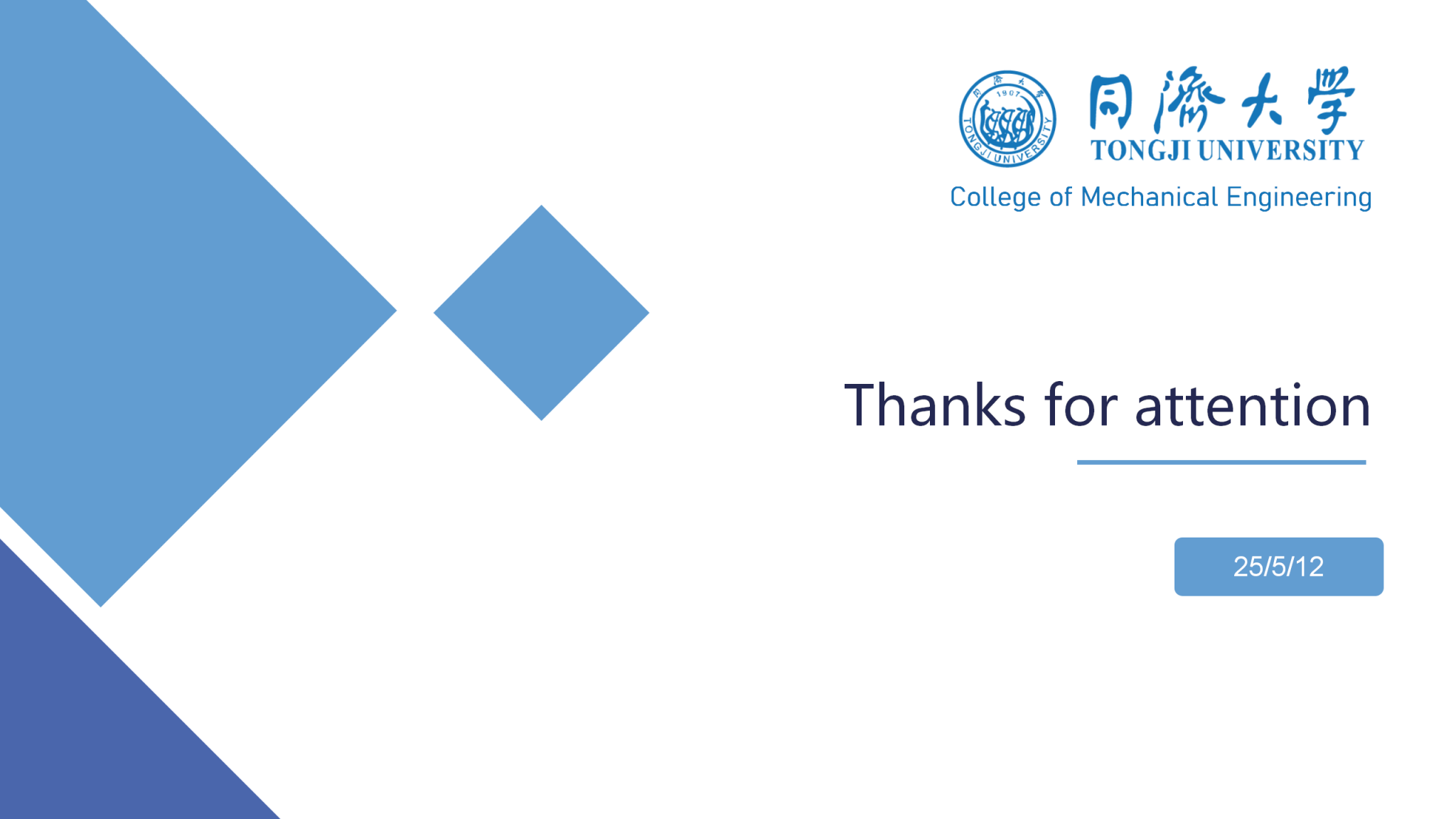
声明:本网站内容版权归许鹏教授课题组所有。未经本课题组授权不得转载、摘编或利用其它方式使用上述作品。
视频链接:陈俊宇_基于TimeGAN的数据增强以改进供热变电站的供热负荷预测_哔哩哔哩_bilibili