文献来源: Yu-Hao Huang, Chang Xu, Yueying Wu, Wu-Jun Li, Jiang Bian
文章摘要:Time series generation models are crucial for applications like data augmentation and privacy preservation. Most existing time series generation models are typically designed to generate data from one specified domain. While leveraging data from other domain for better generalization is proved to work in other application areas, this approach remains challenging for time series modeling due to the large divergence in patterns among different real world time series categories. In this paper, we propose a multi-domain time series diffusion model with domain prompts, named TimeDP. In TimeDP, we utilize a time series semantic prototype module which defines time series prototypes to represent time series basis, each prototype vector serving as "word" representing some elementary time series feature. A prototype assignment module is applied to extract the extract domain specific prototype weights, for learning domain prompts as generation condition. During sampling, we extract "domain prompt" with few-shot samples from the target domain and use the domain prompts as condition to generate time series samples. Experiments demonstrate that our method outperforms baselines to provide the state-of-the-art in-domain generation quality and strong unseen domain generation capability.
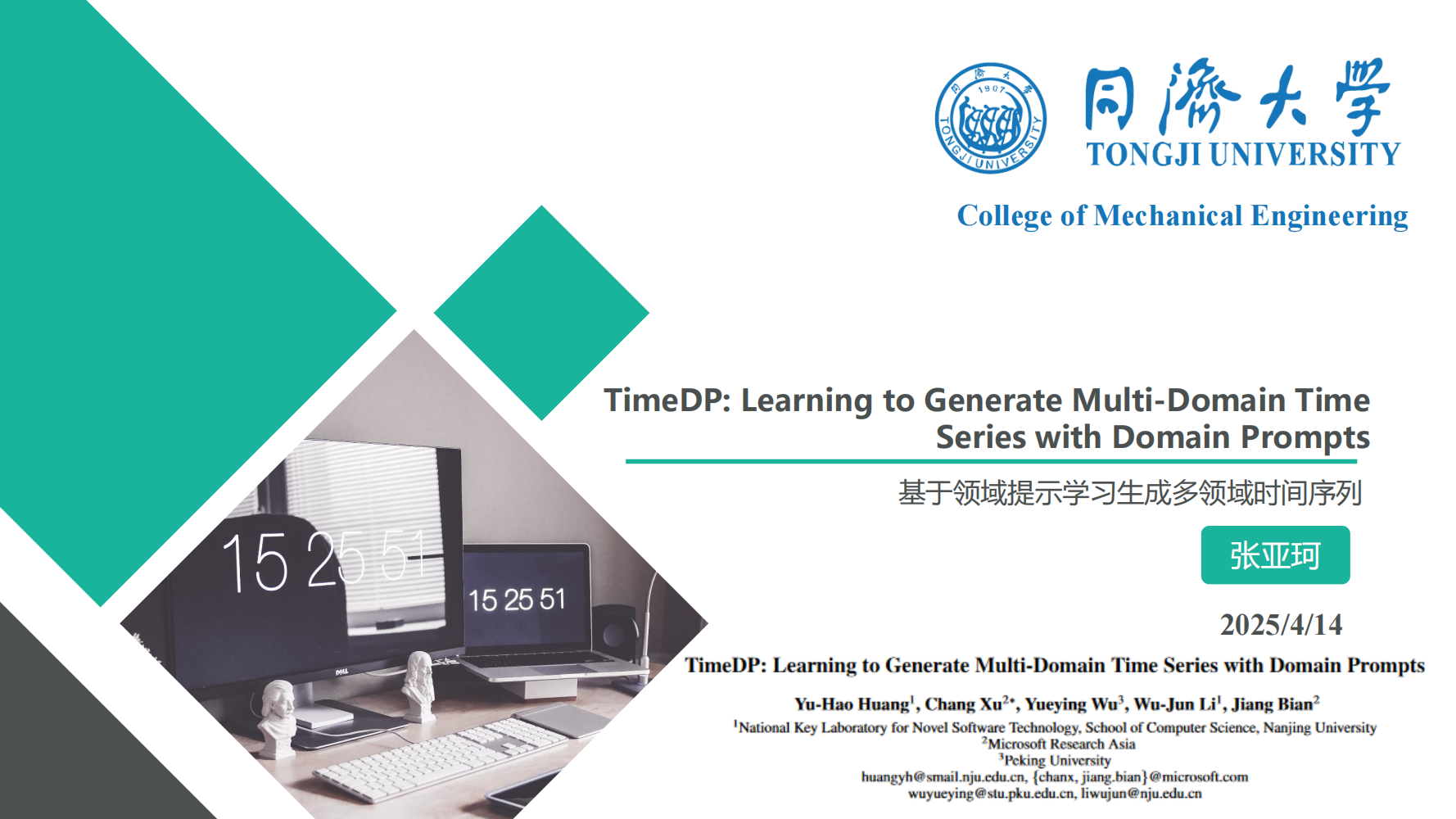
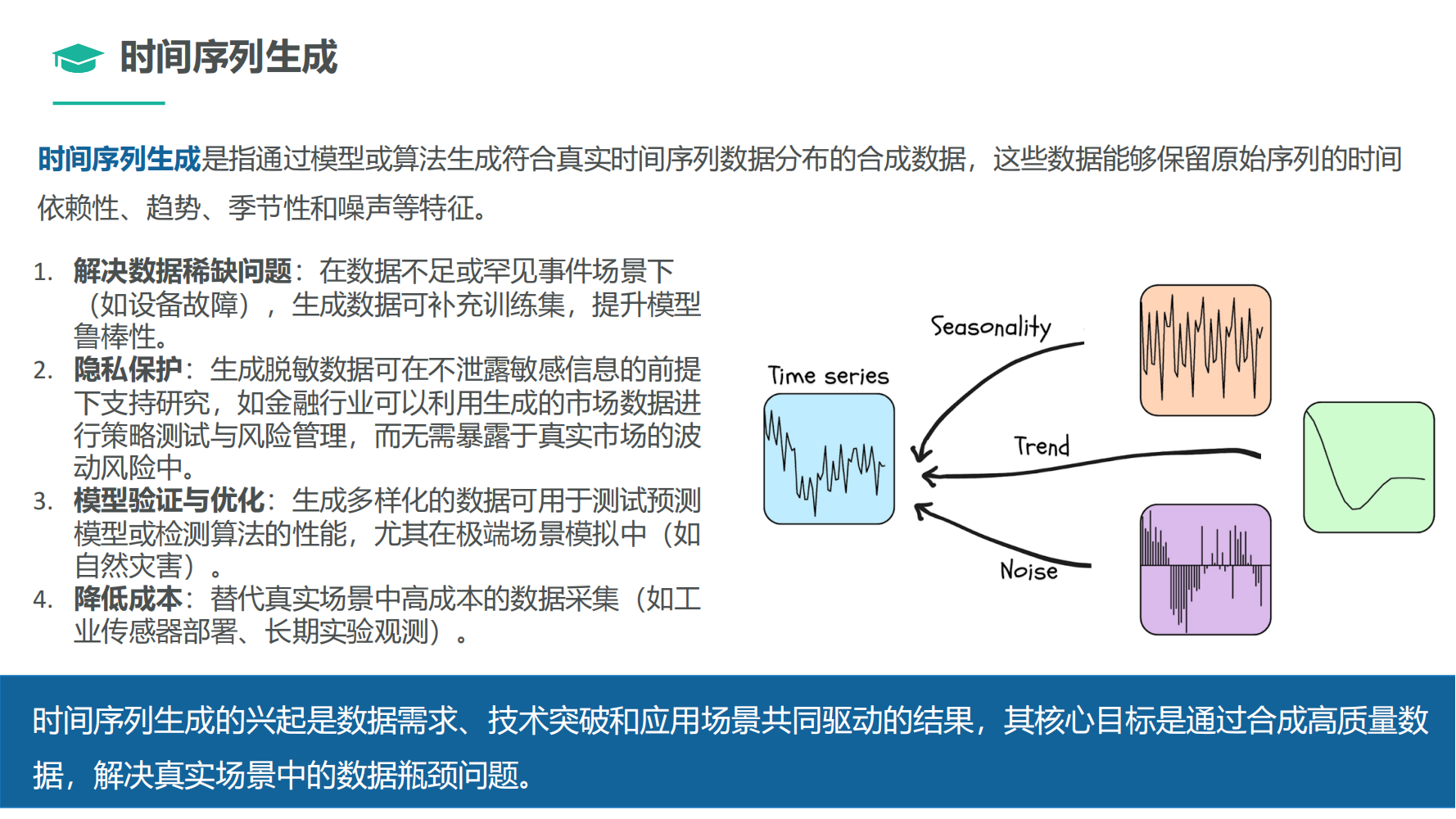
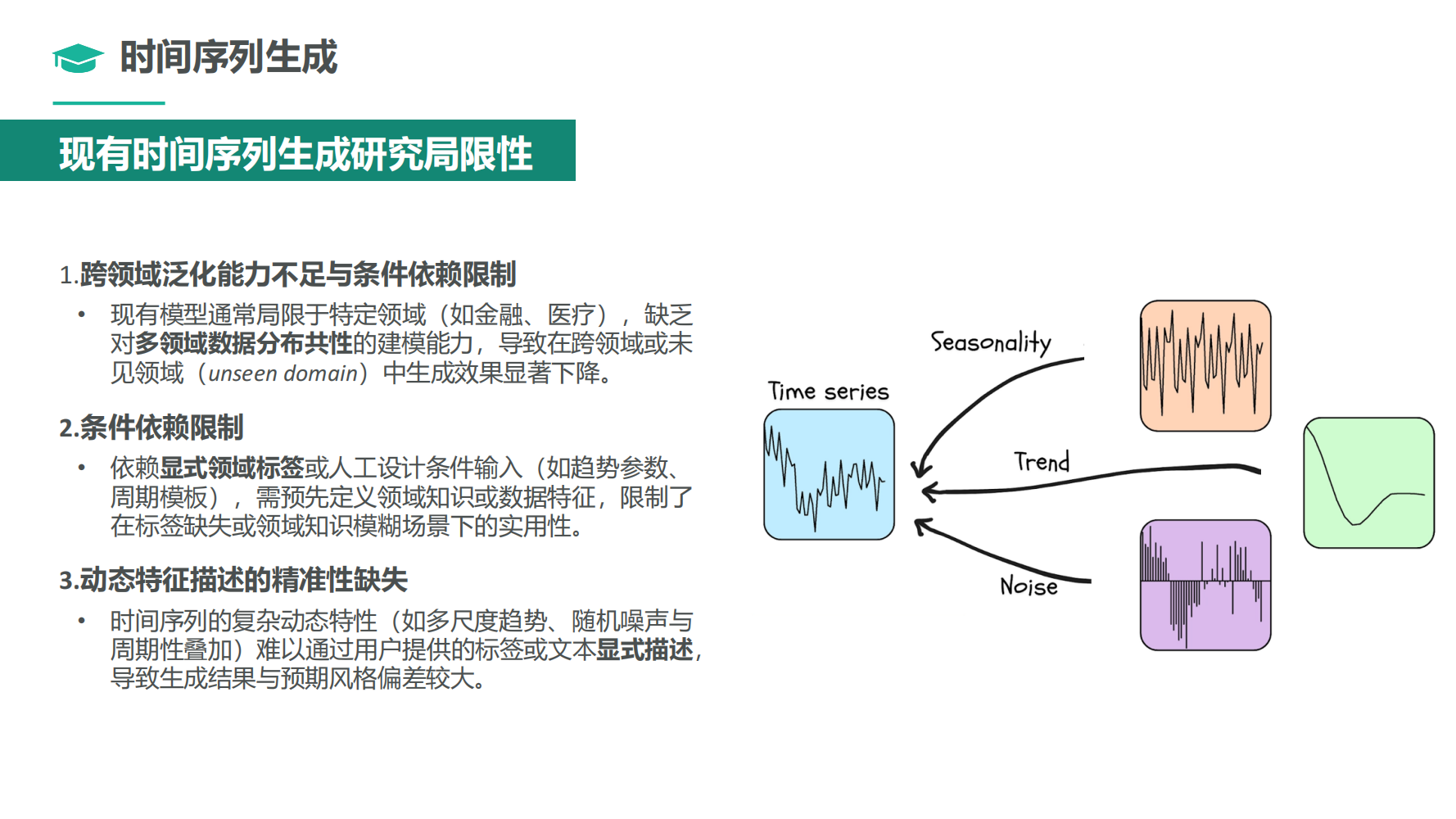
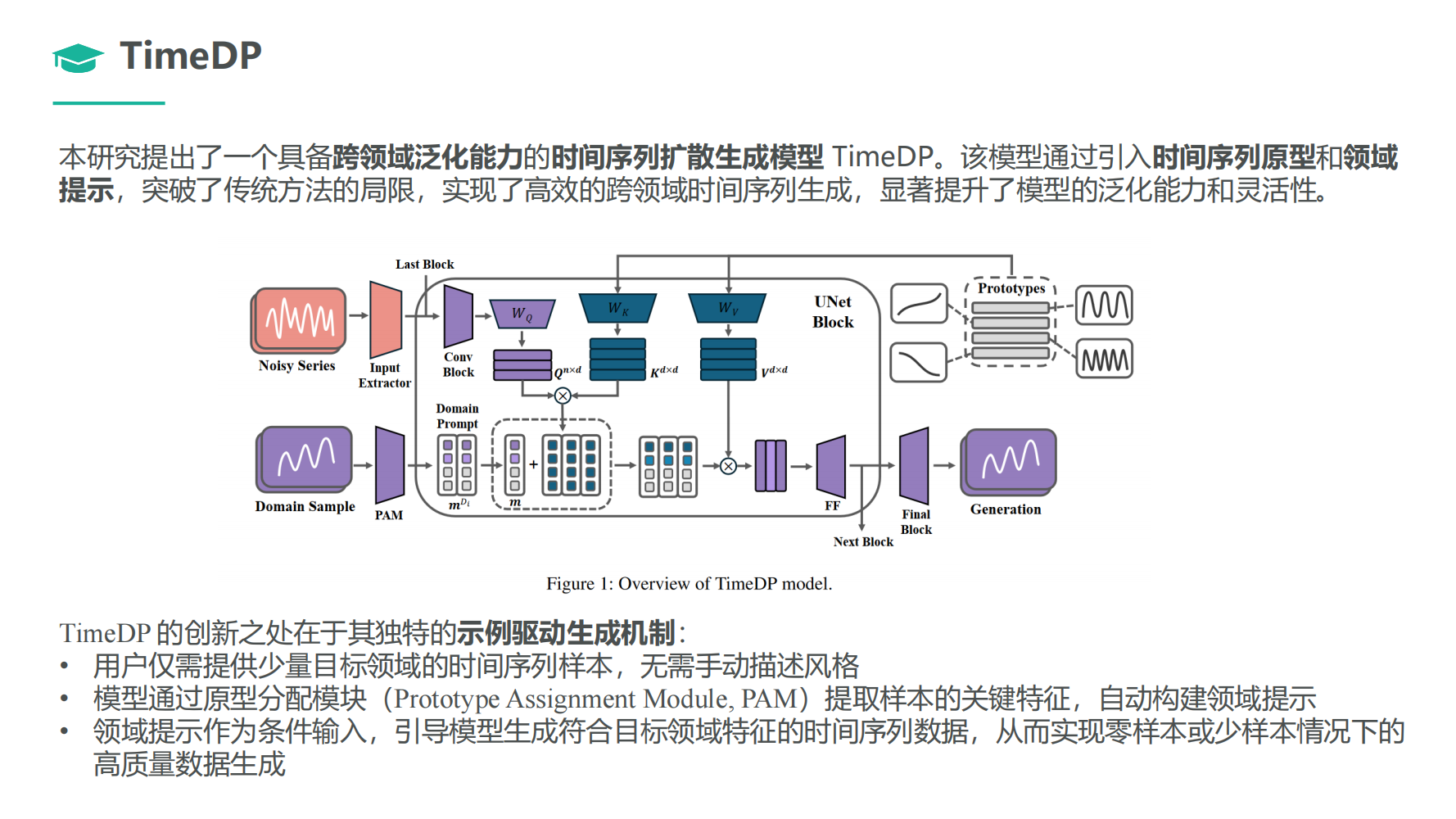
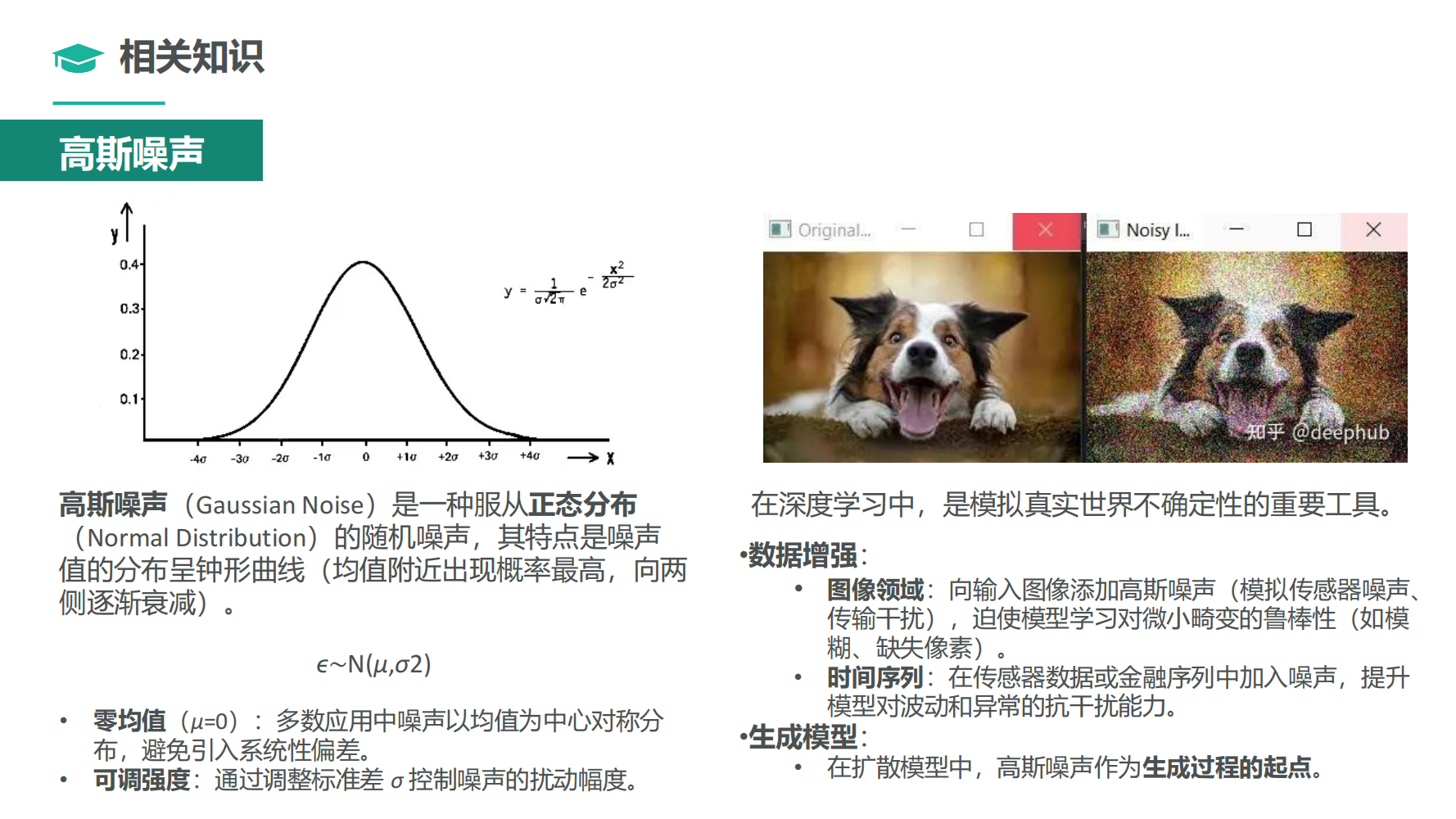
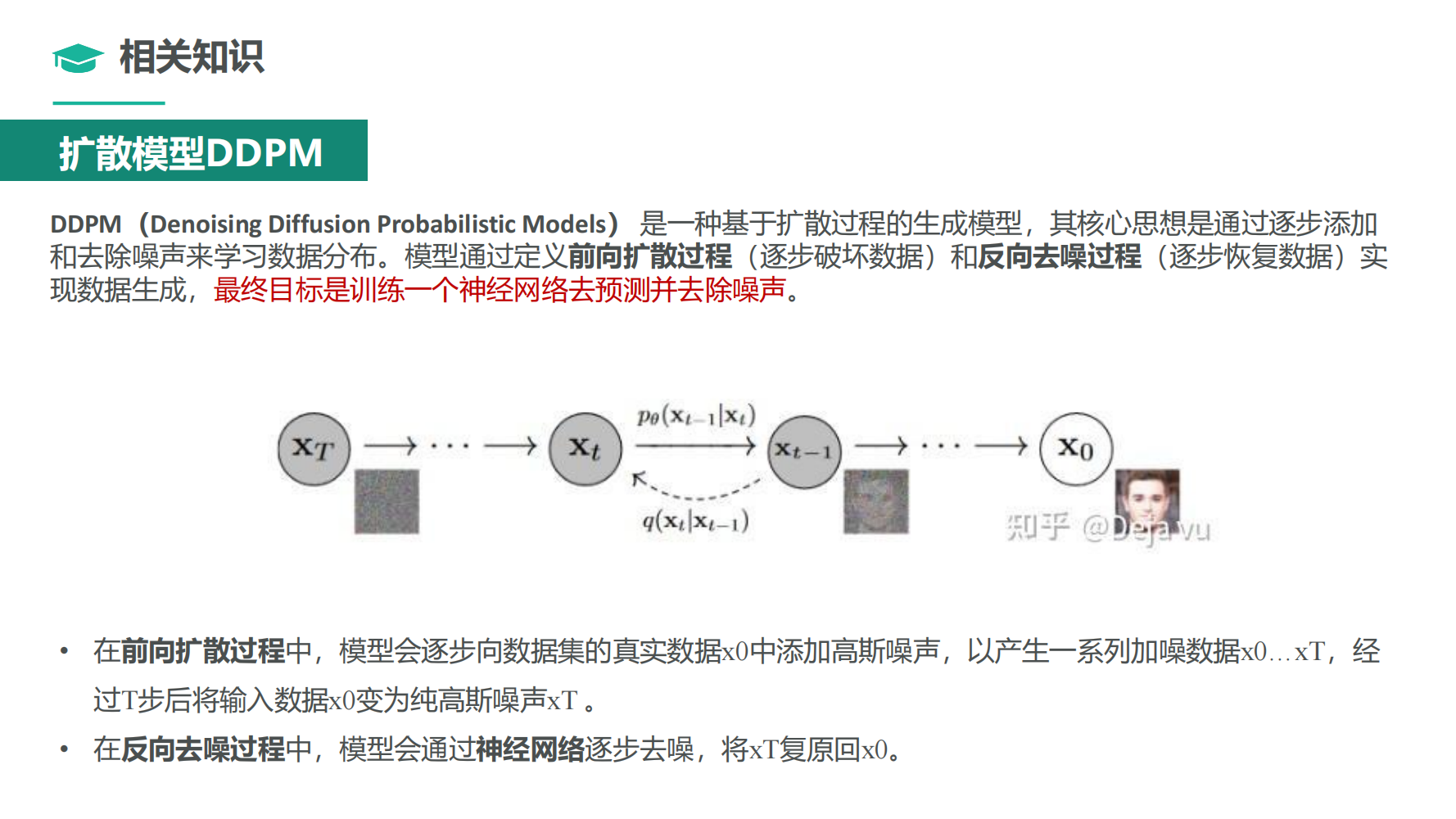
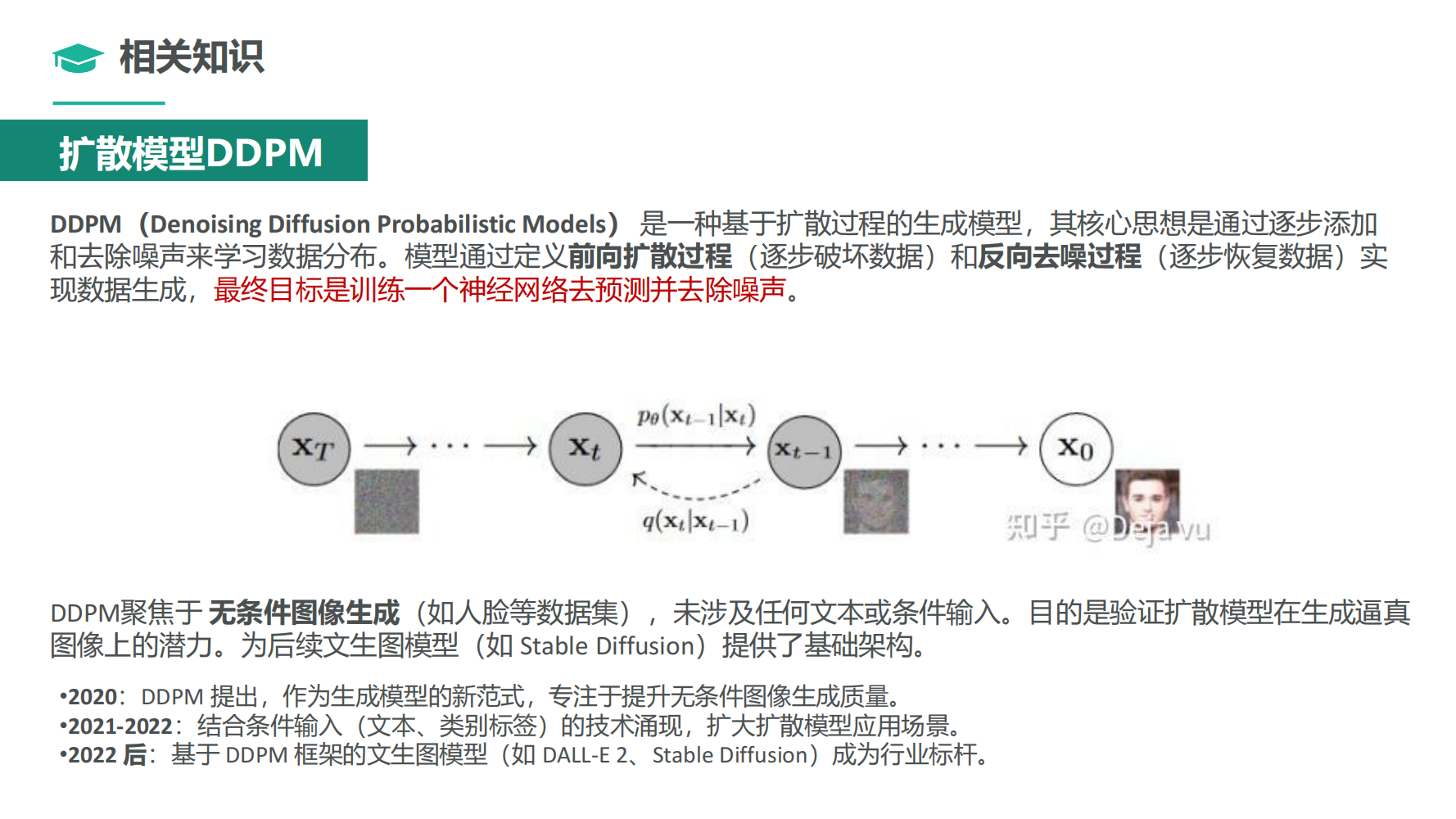
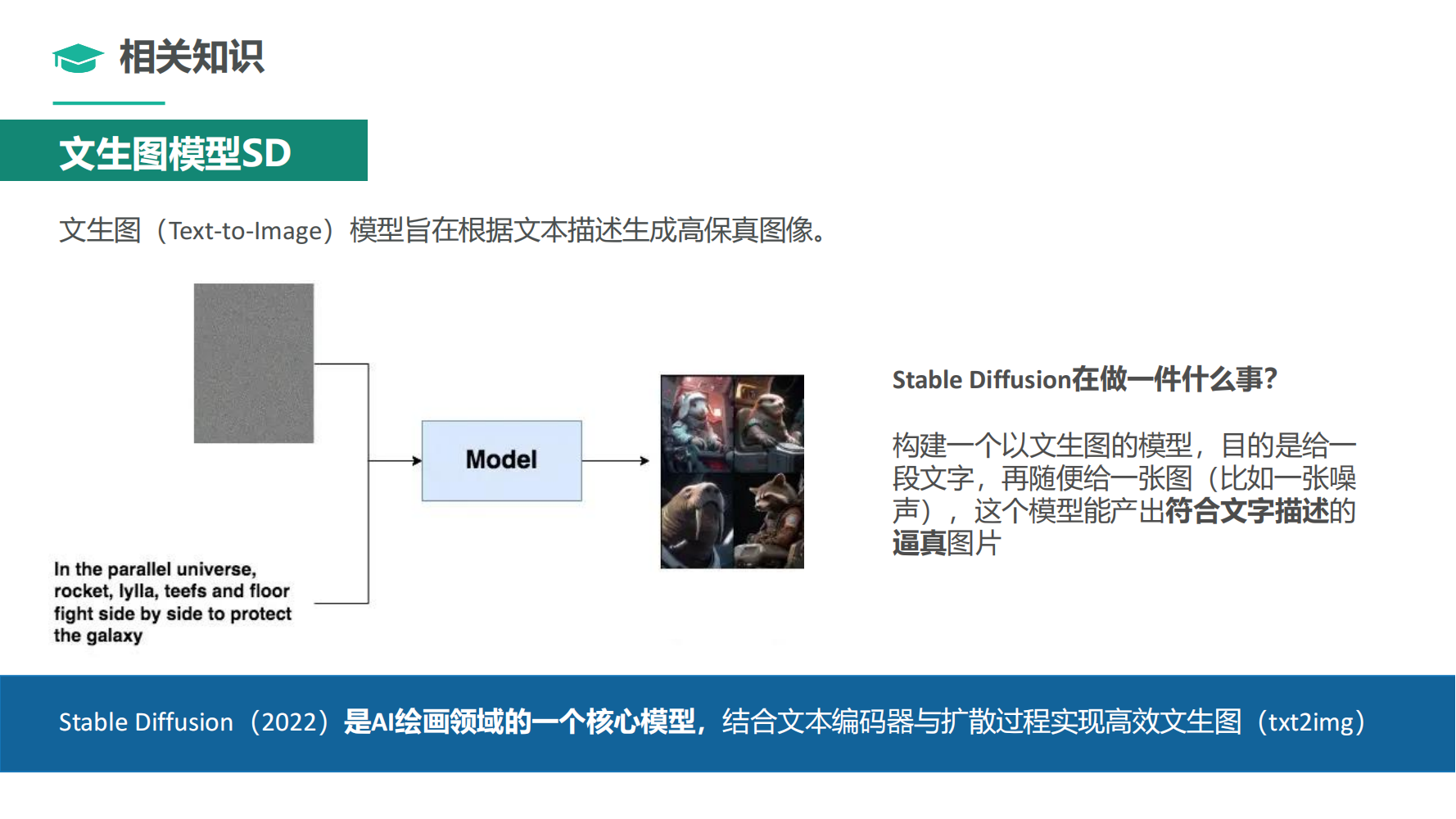
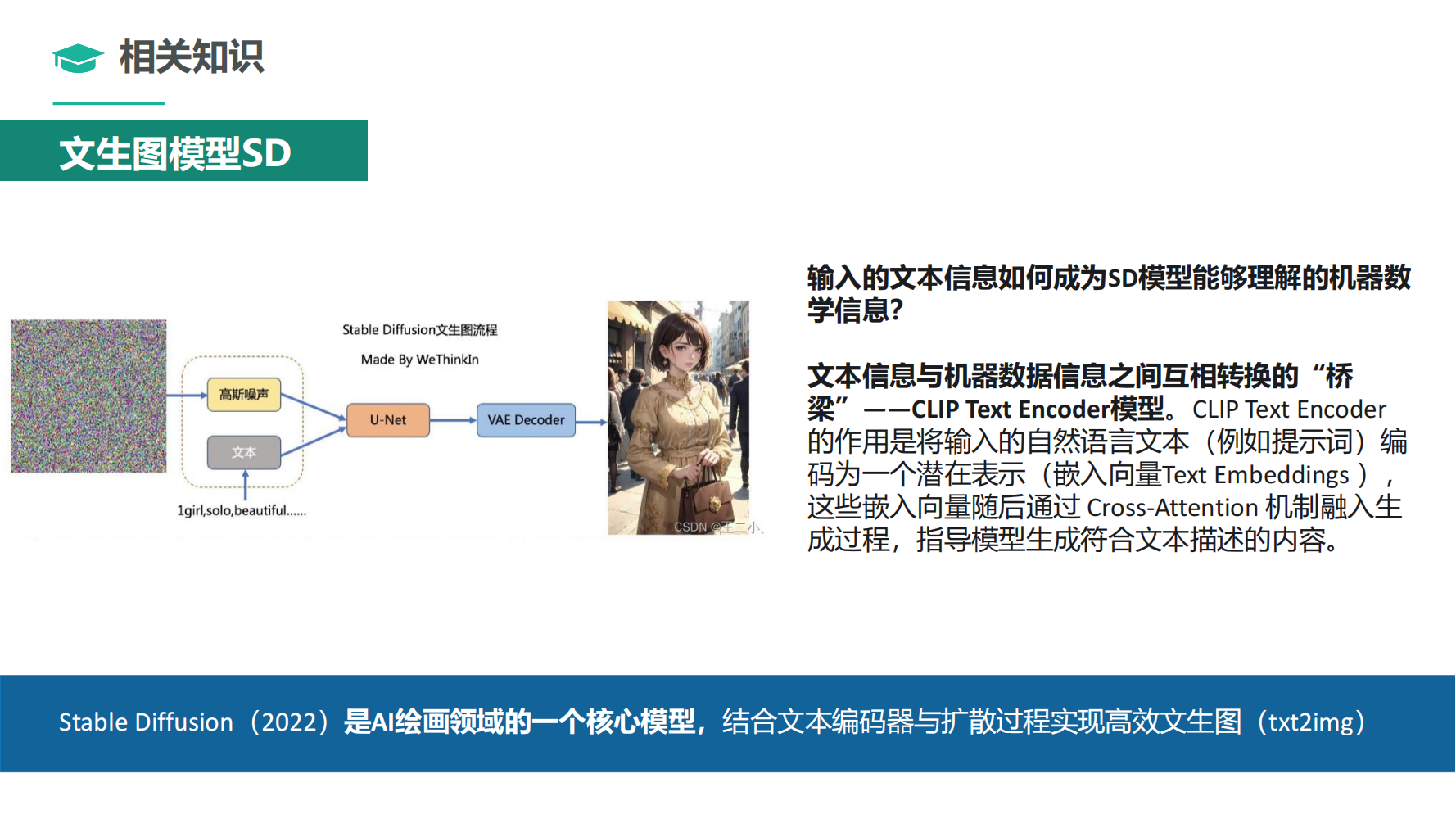
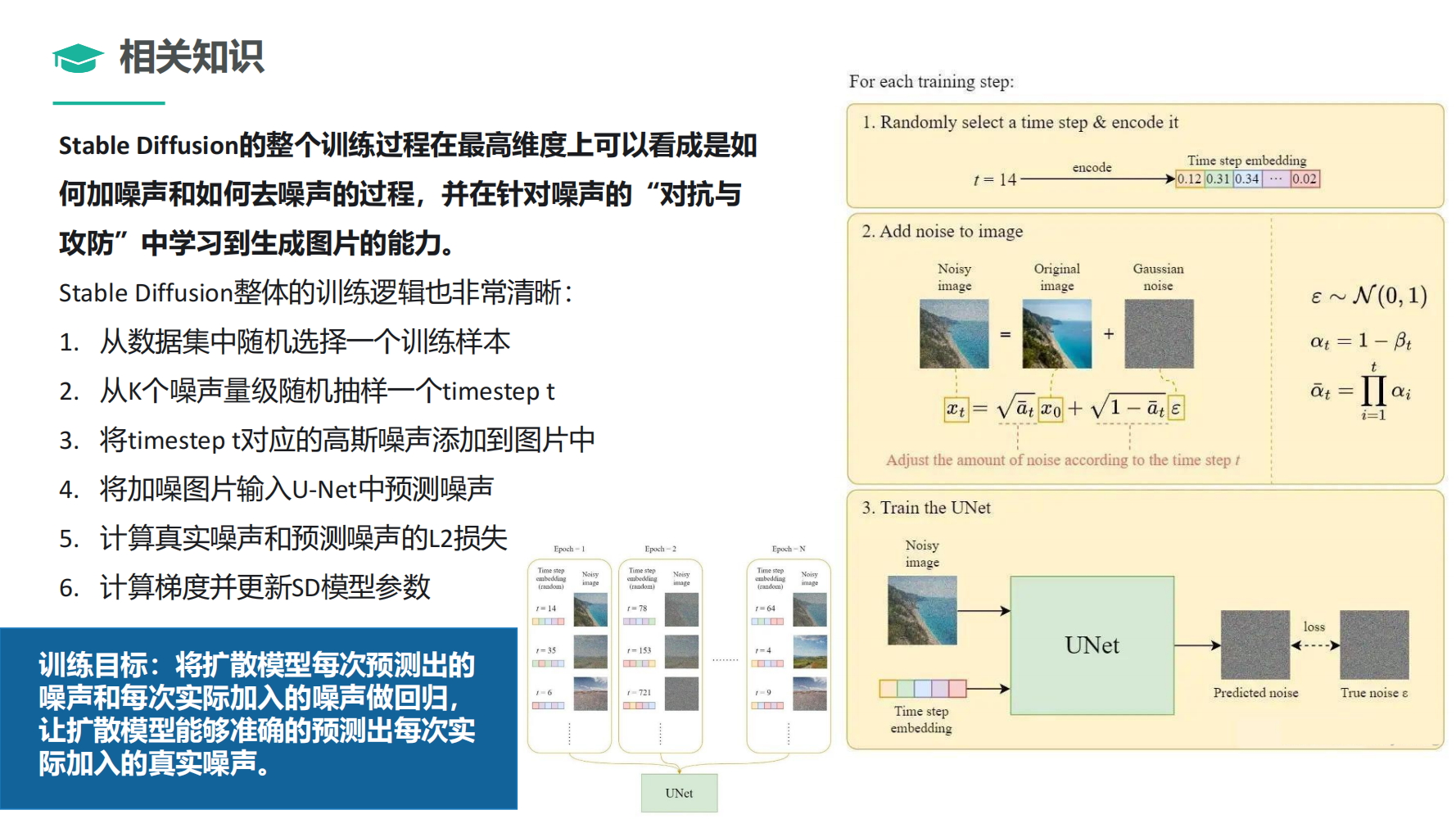
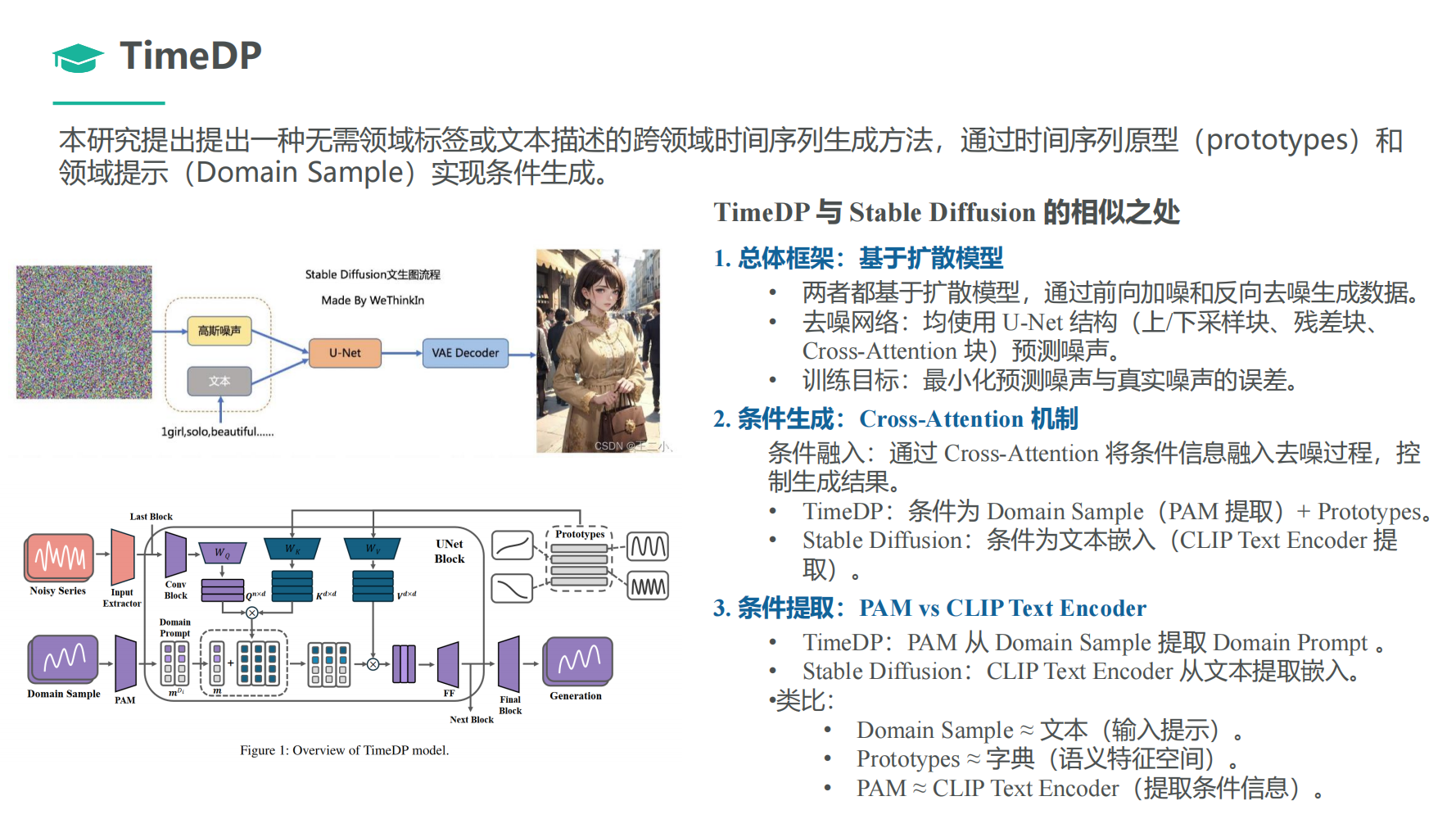
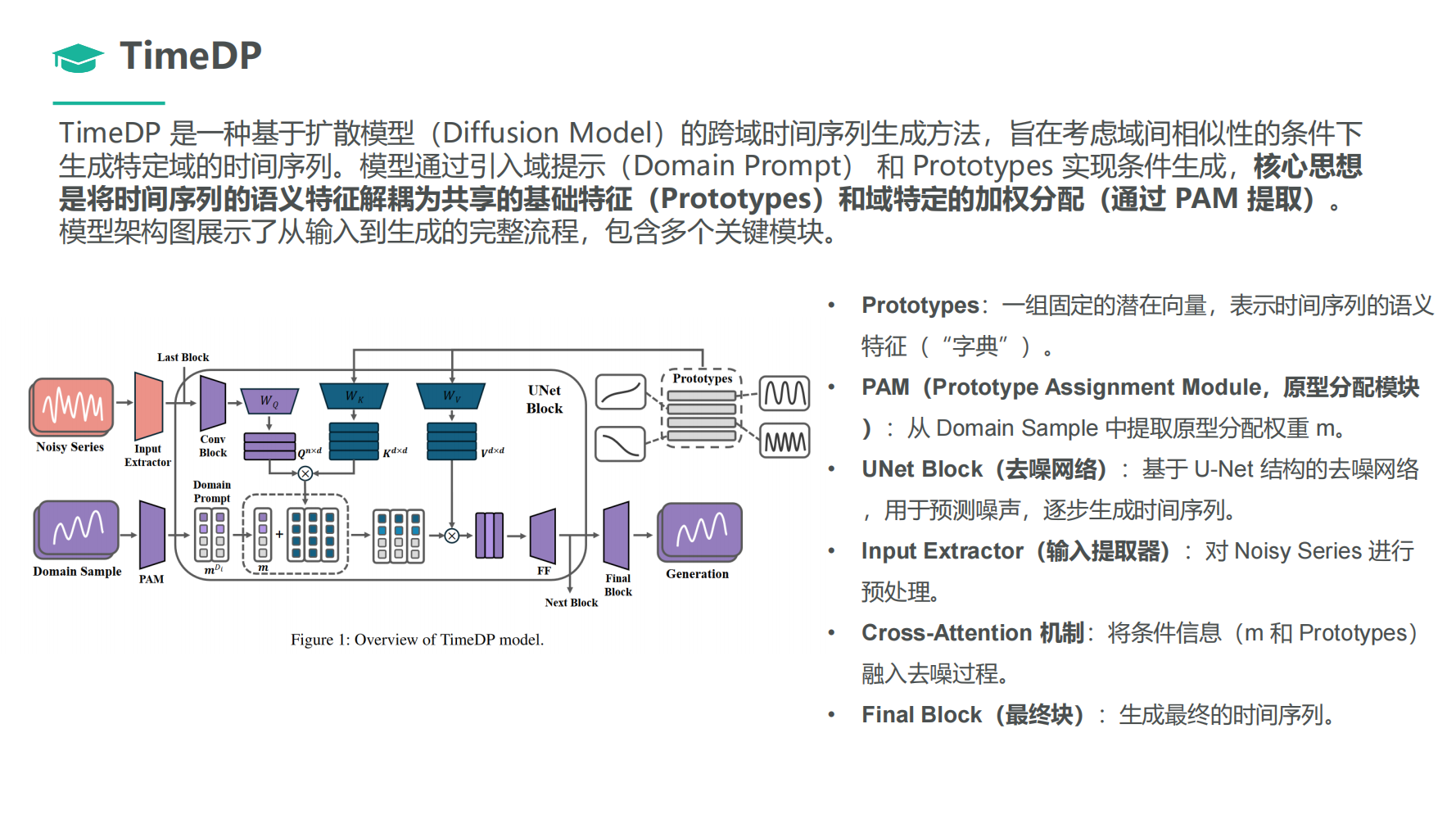
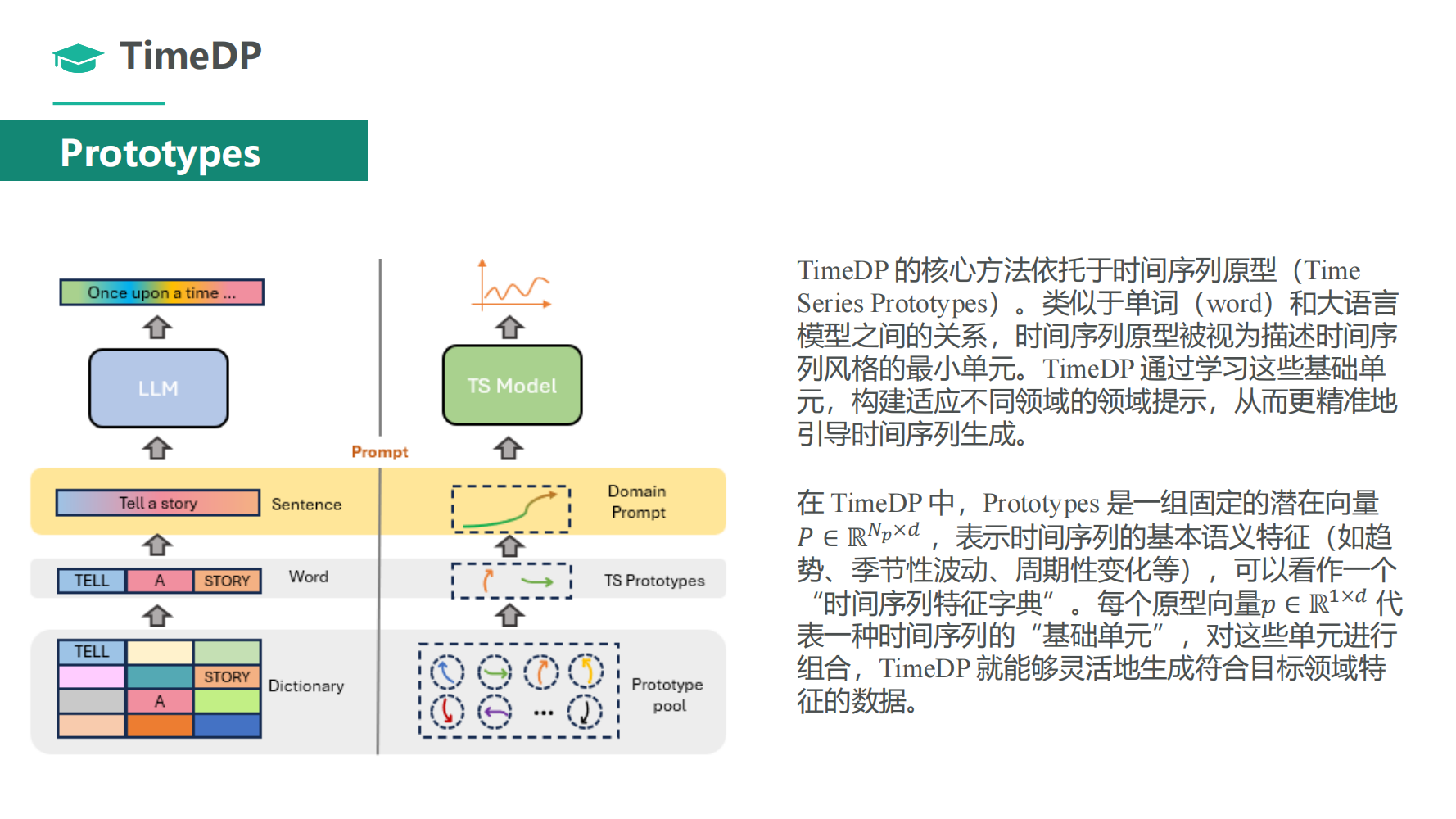
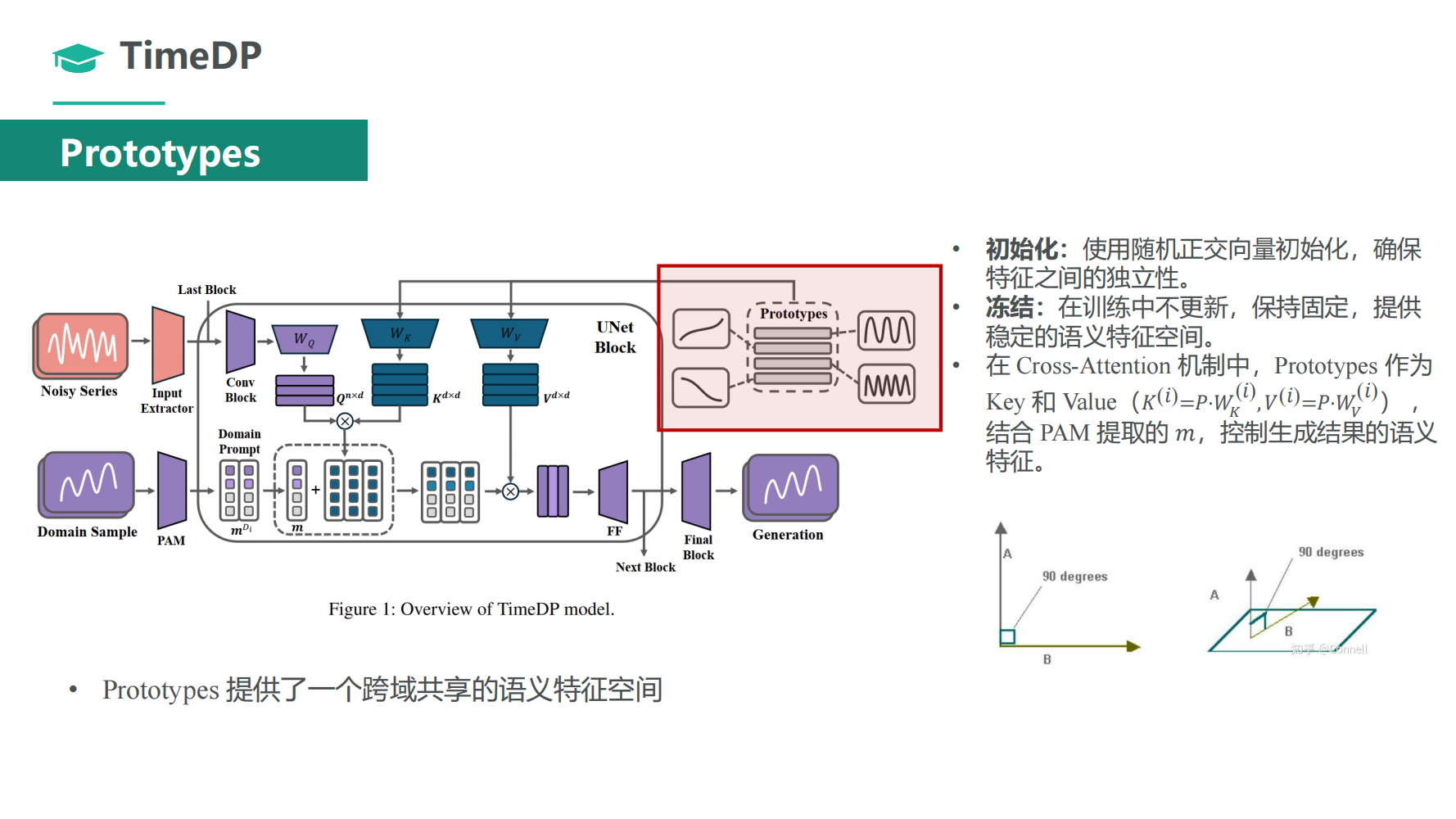
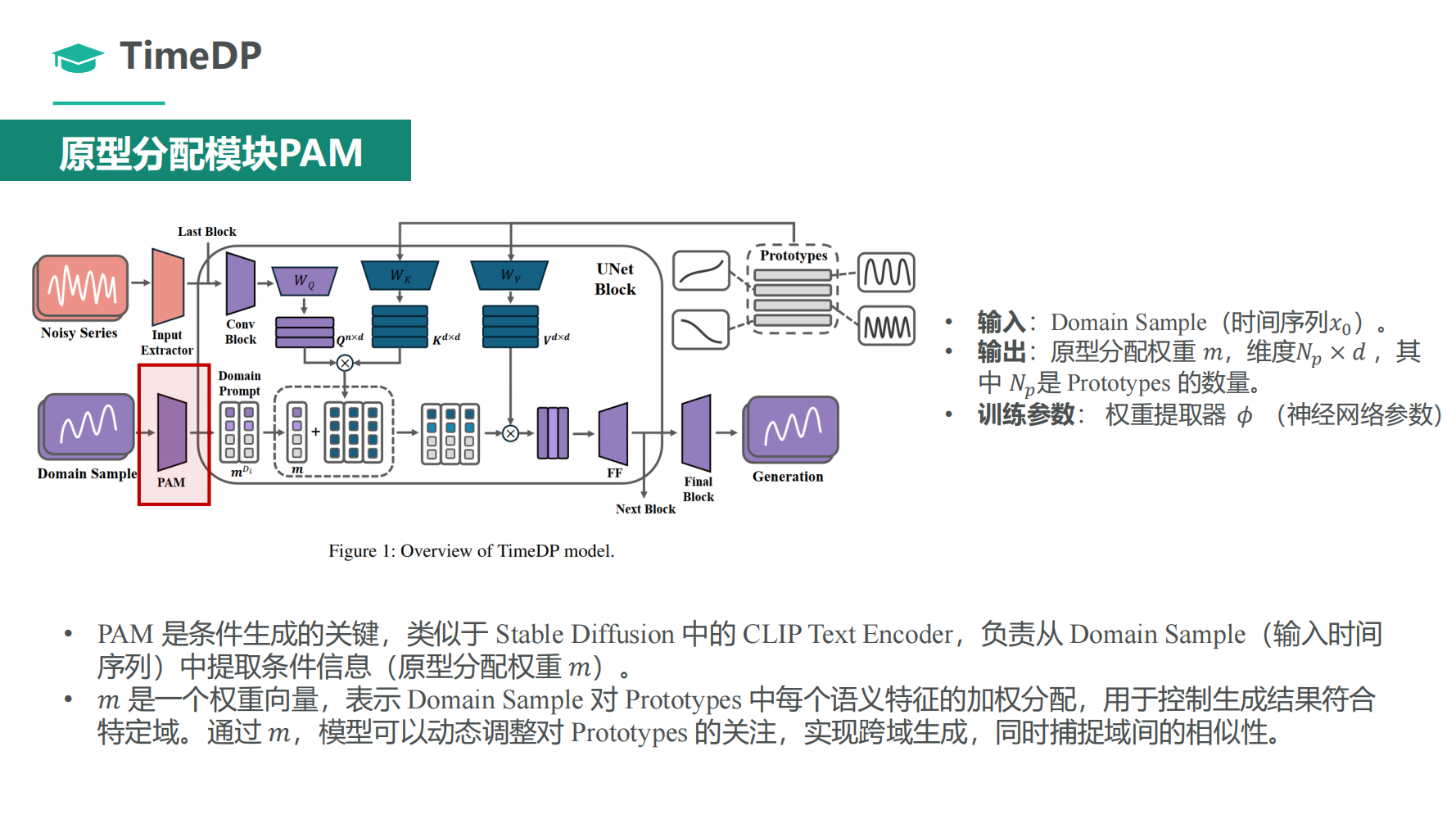
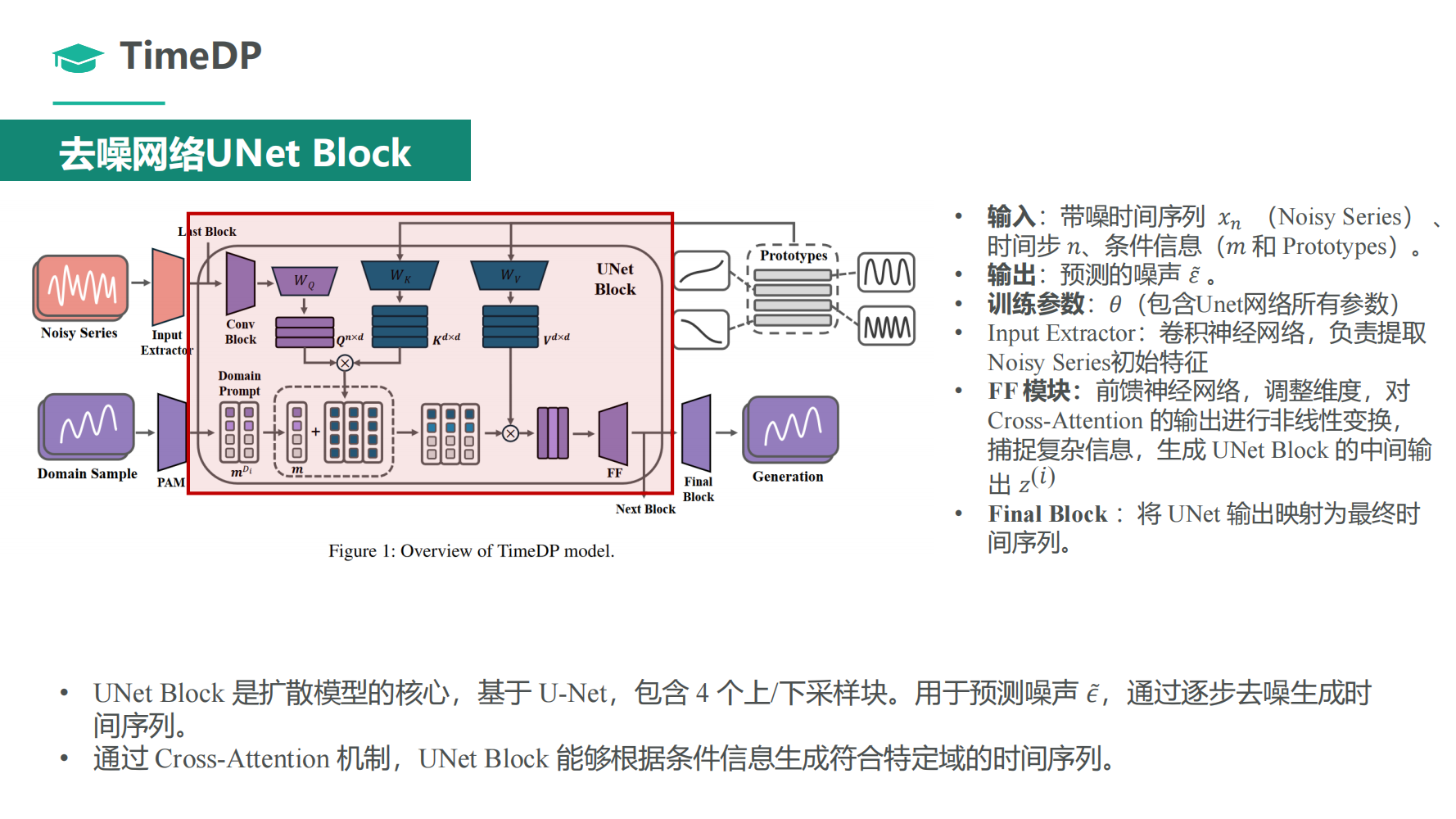
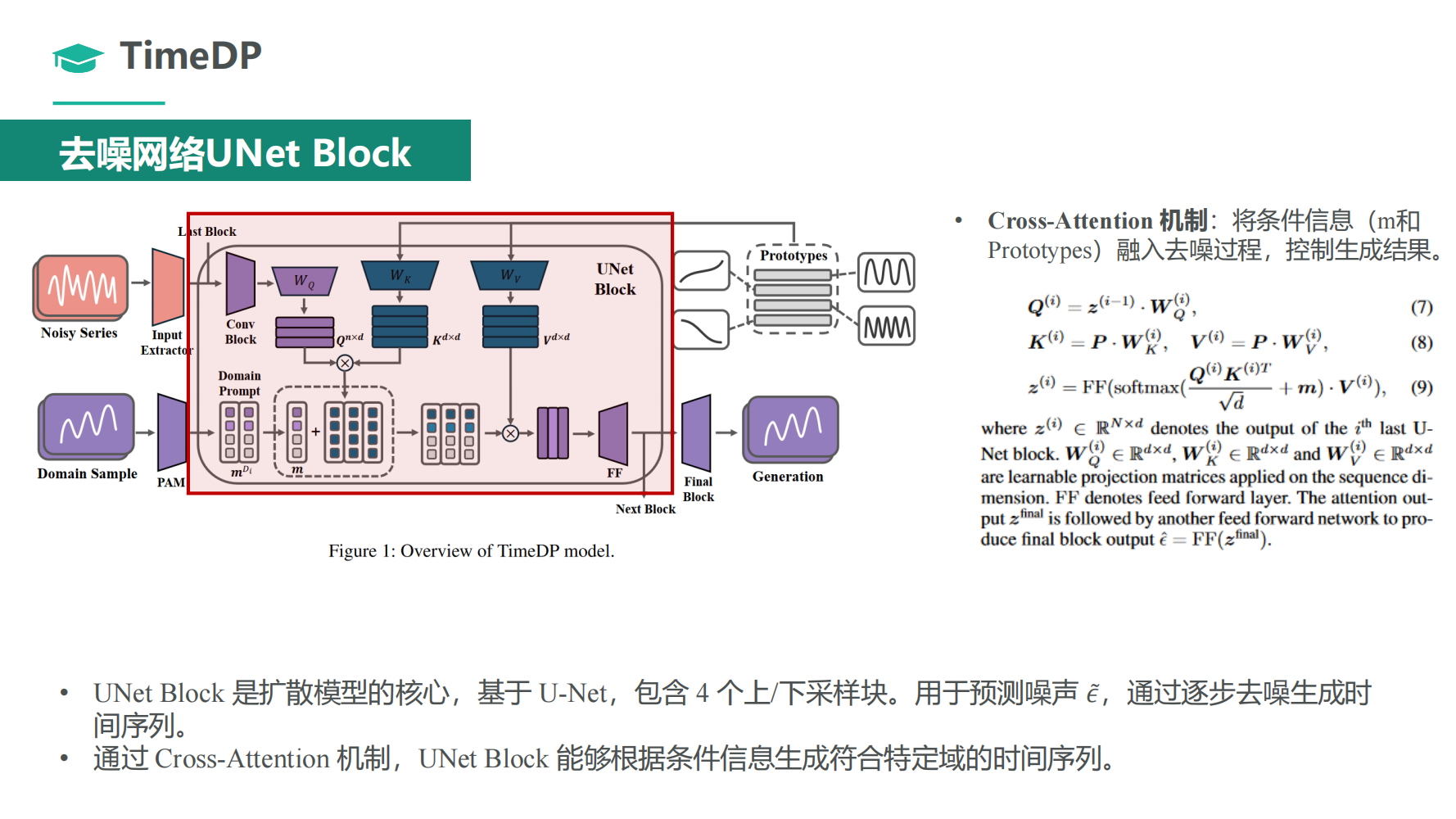
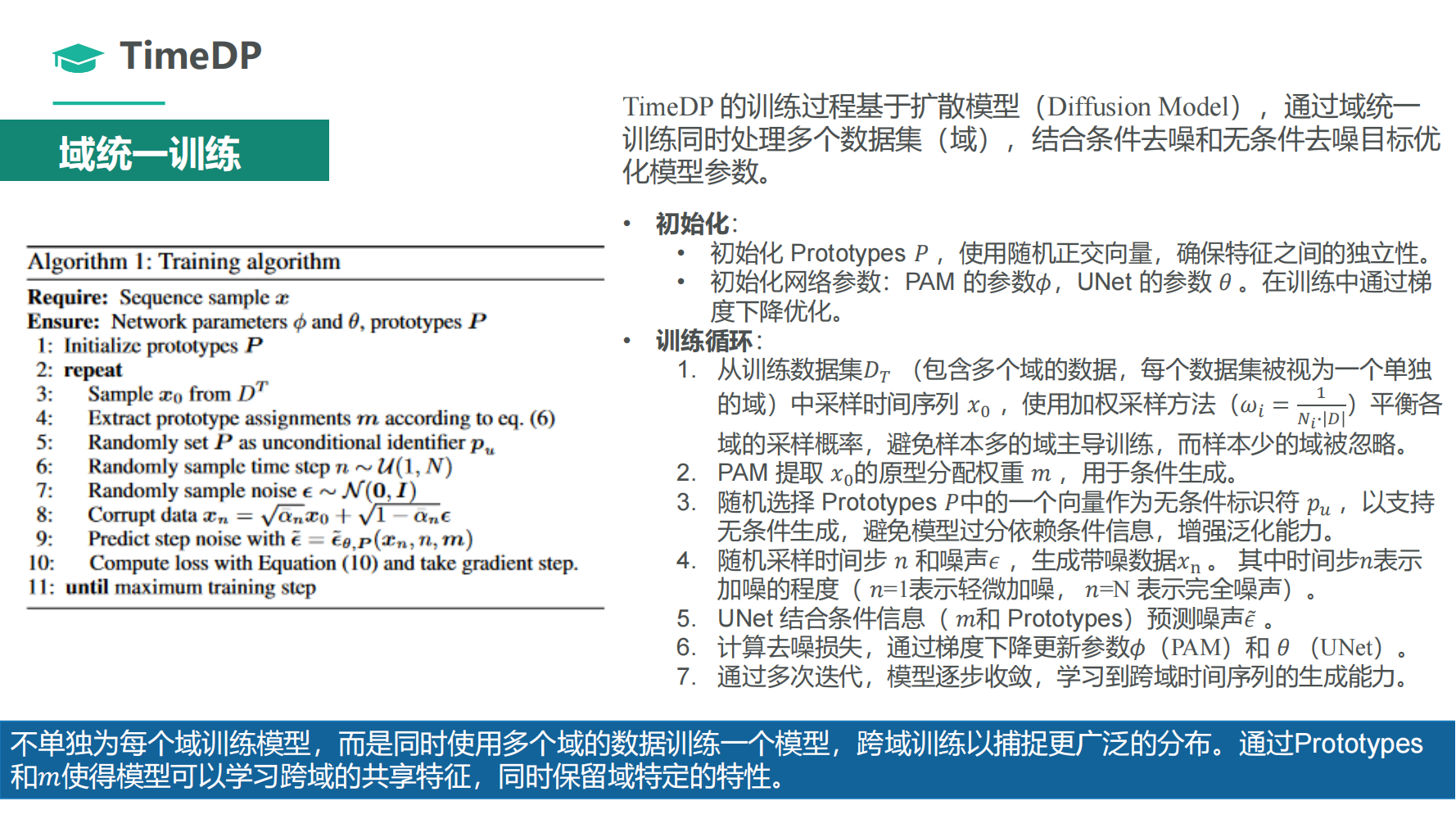
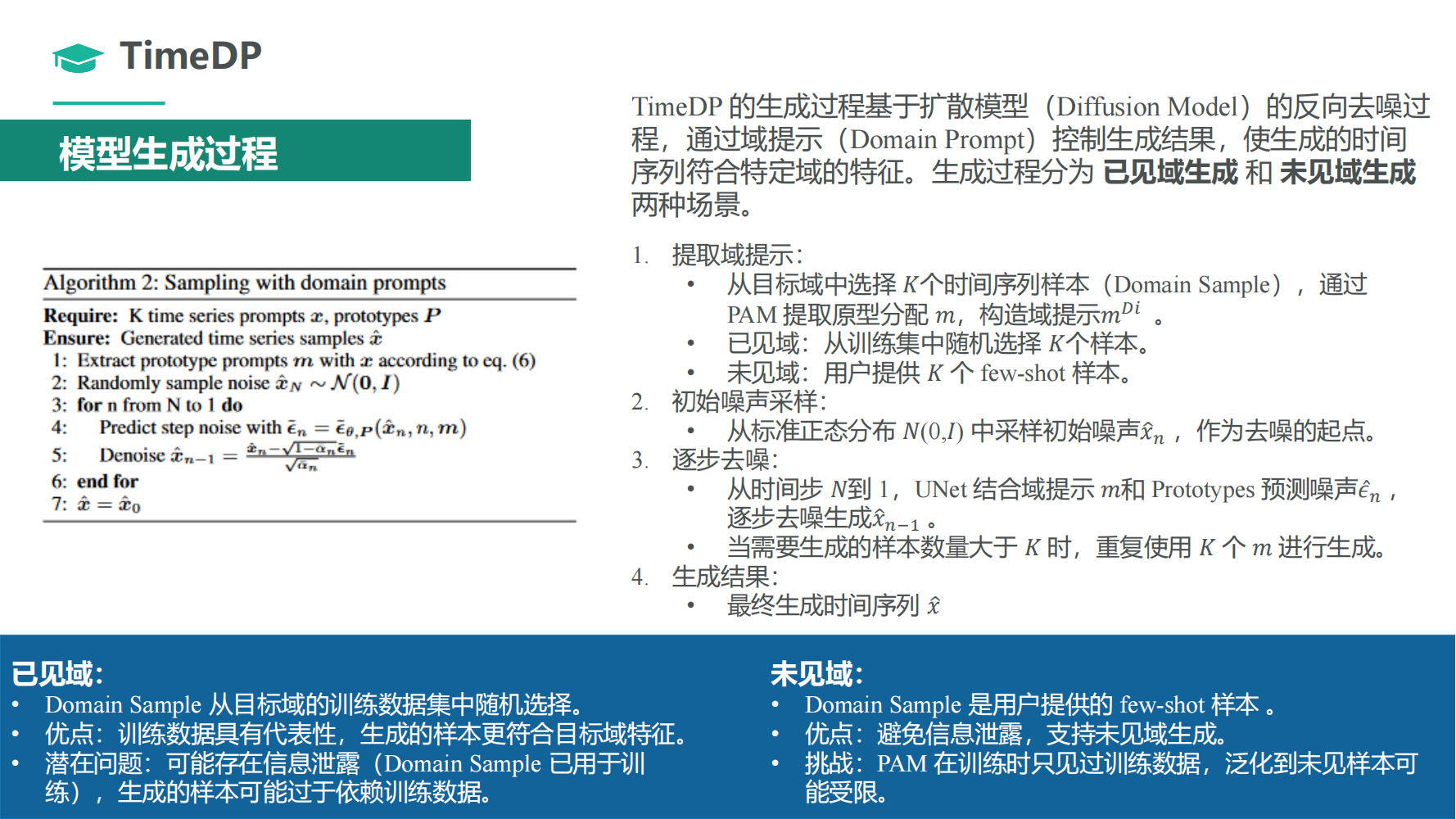
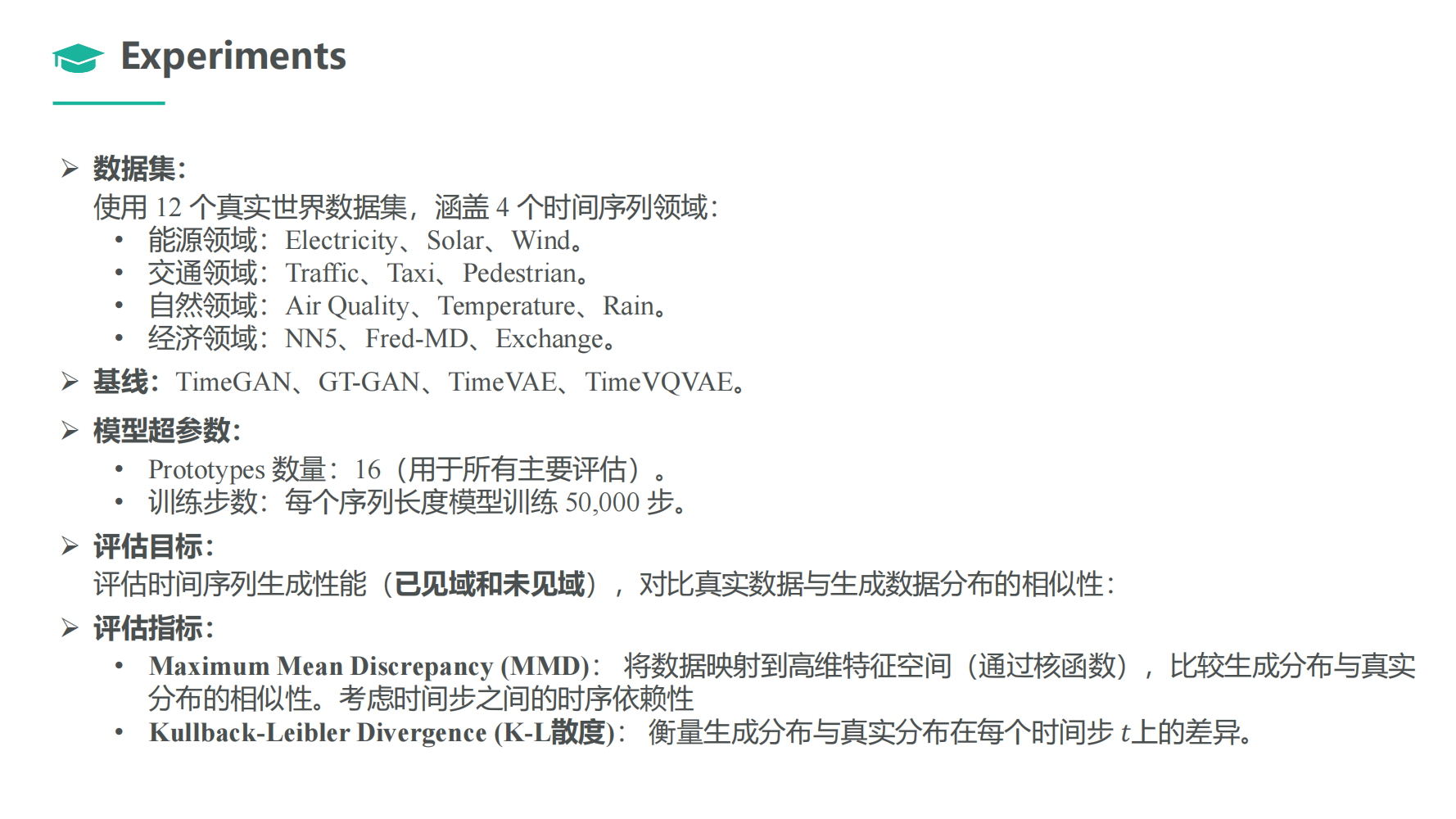
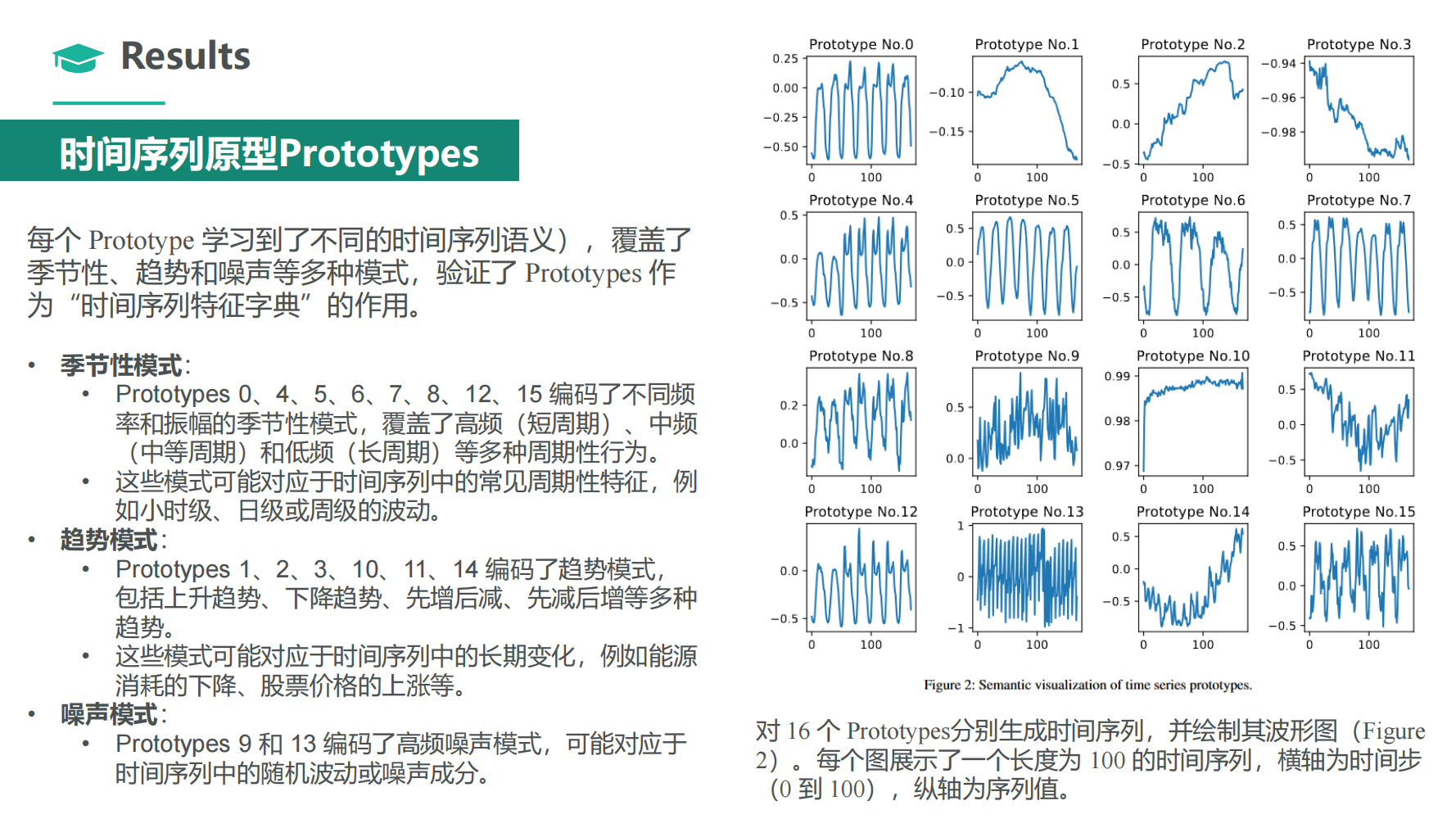
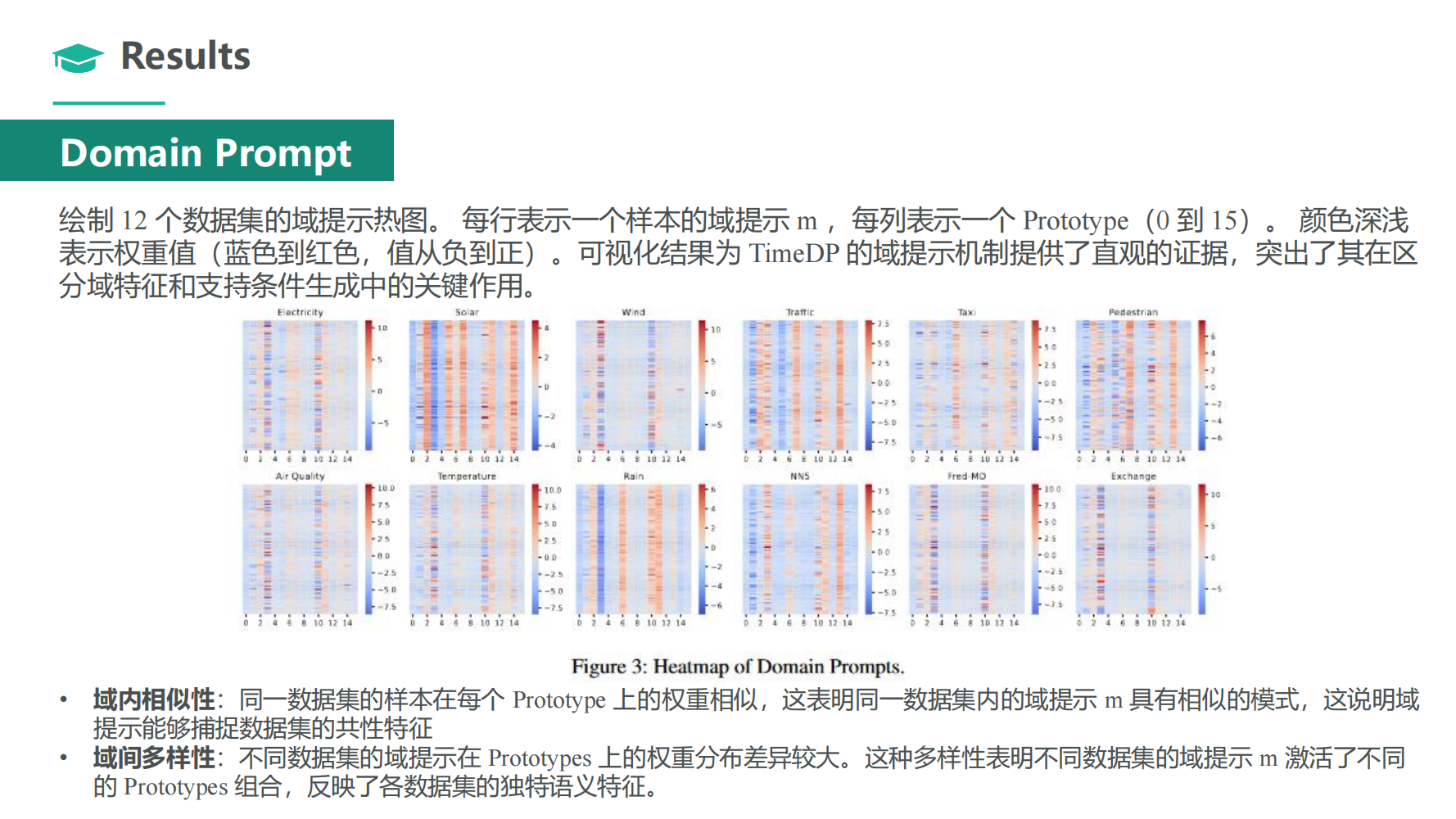
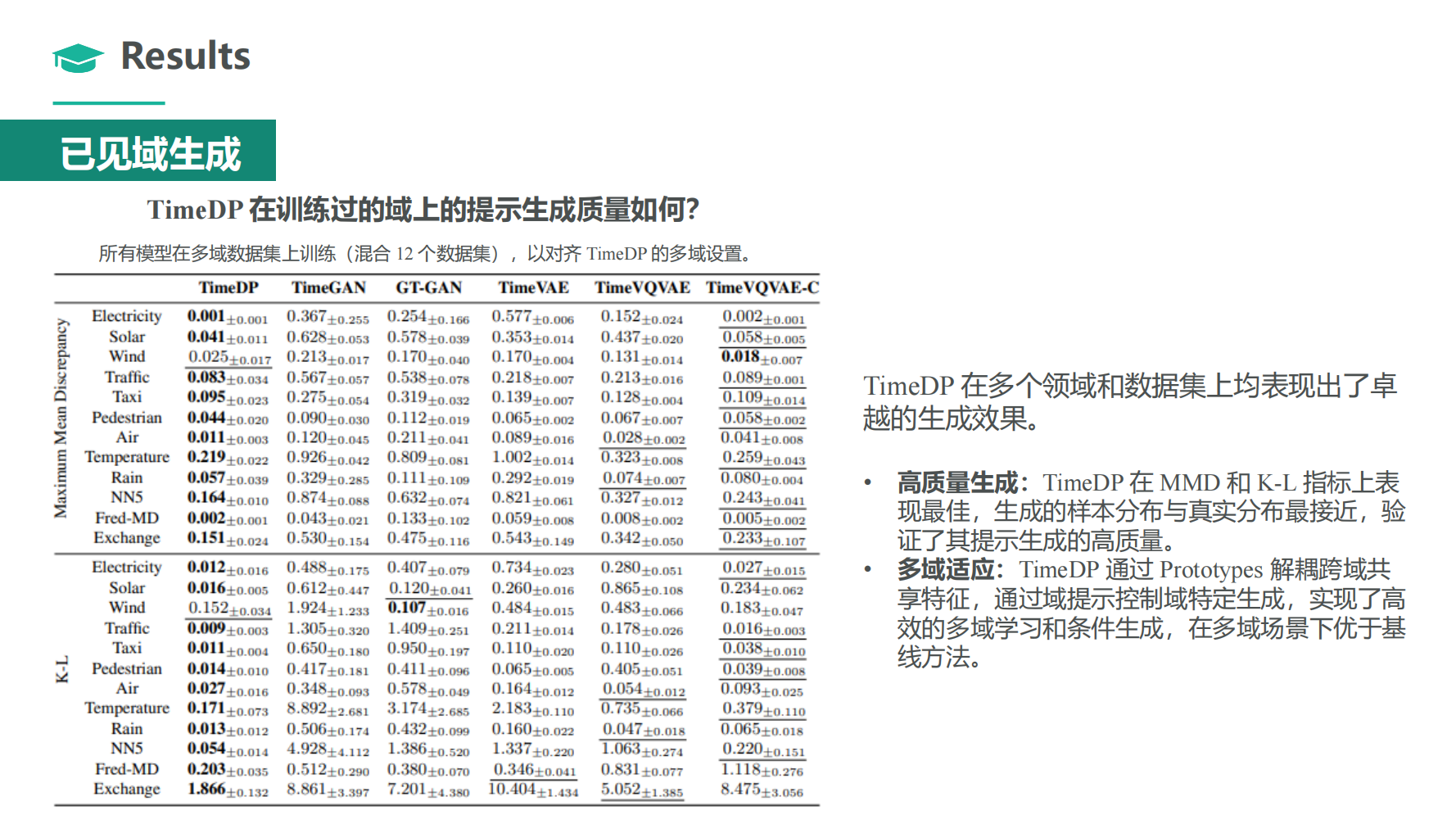
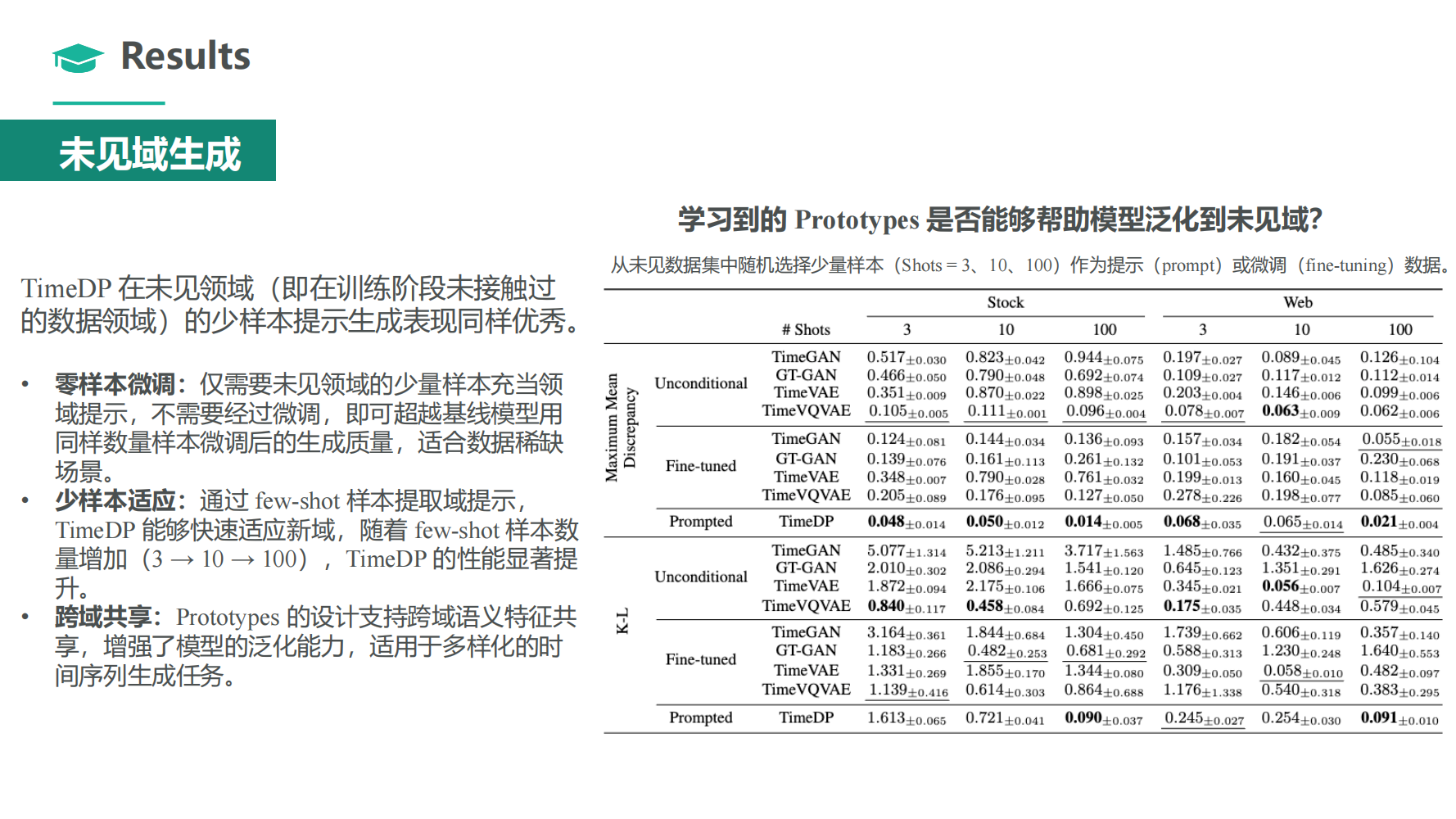
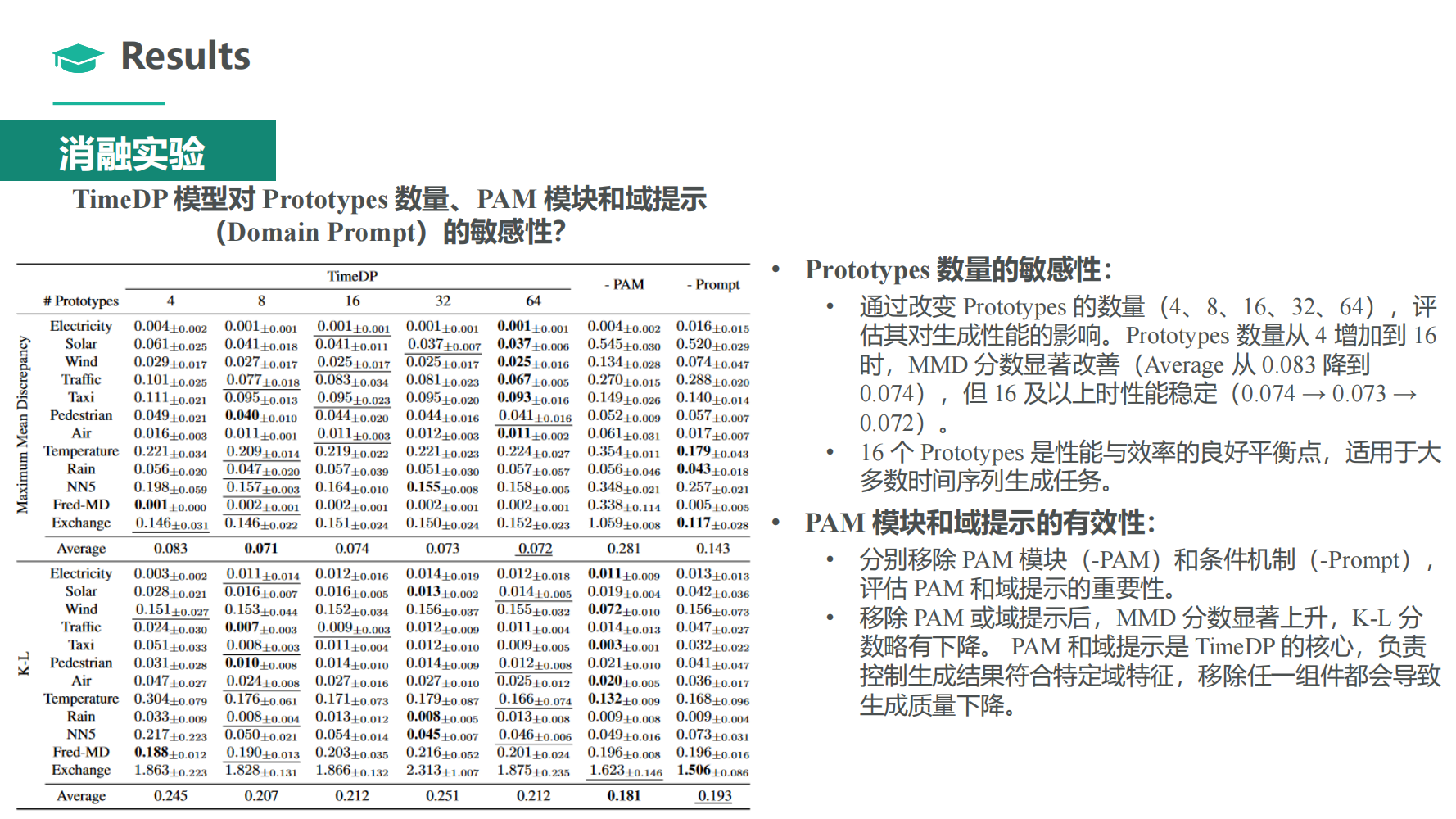
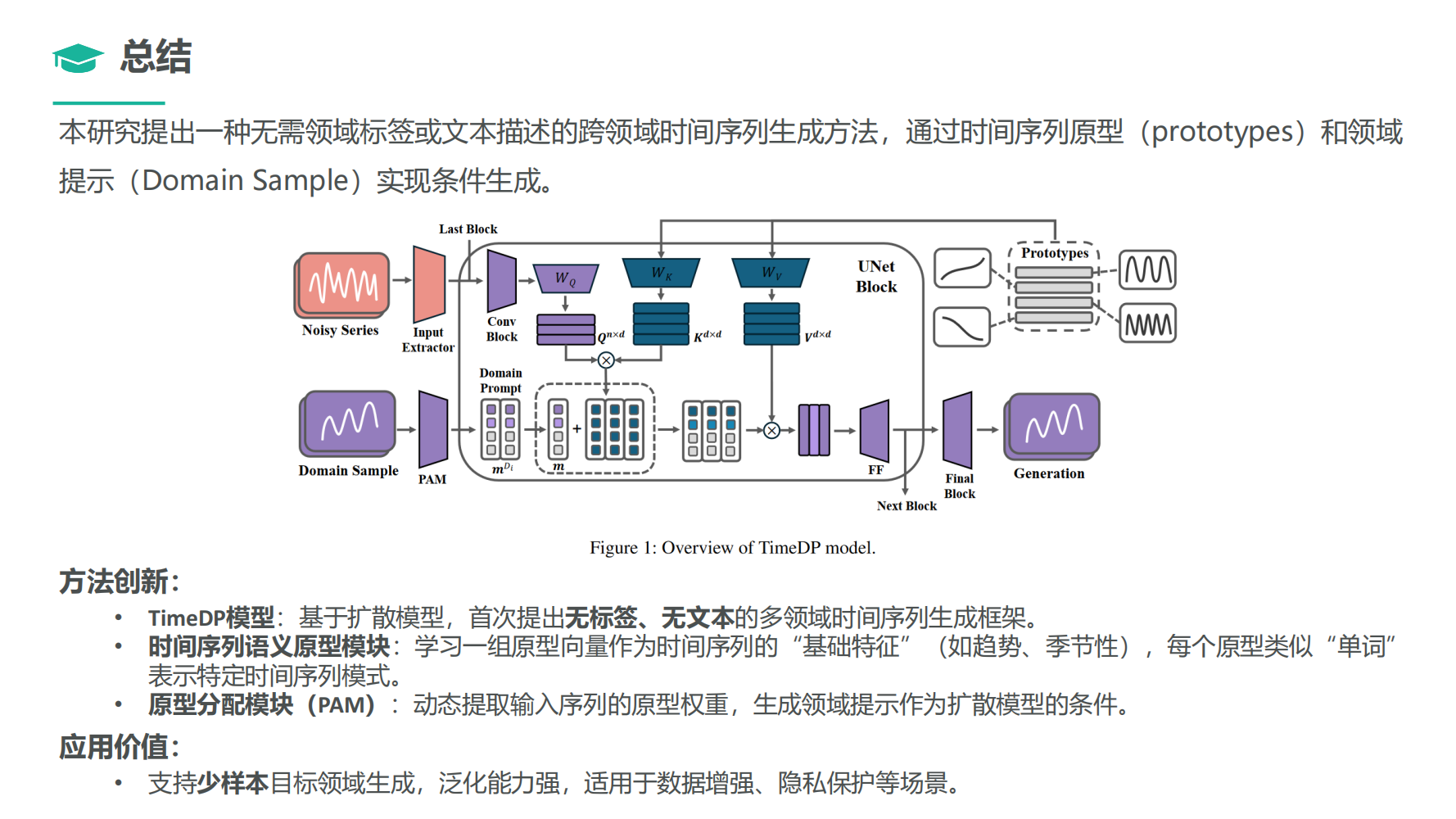
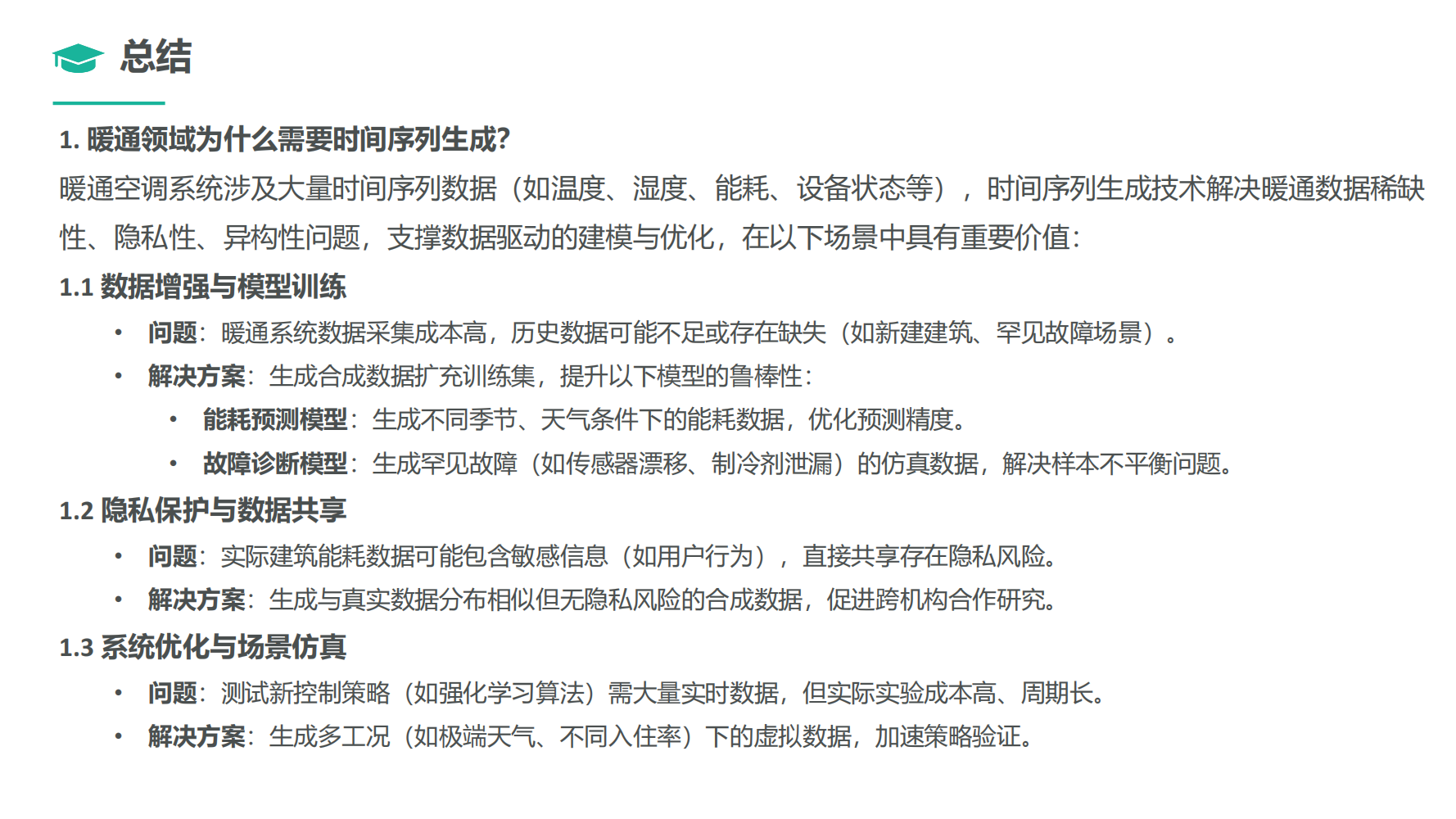
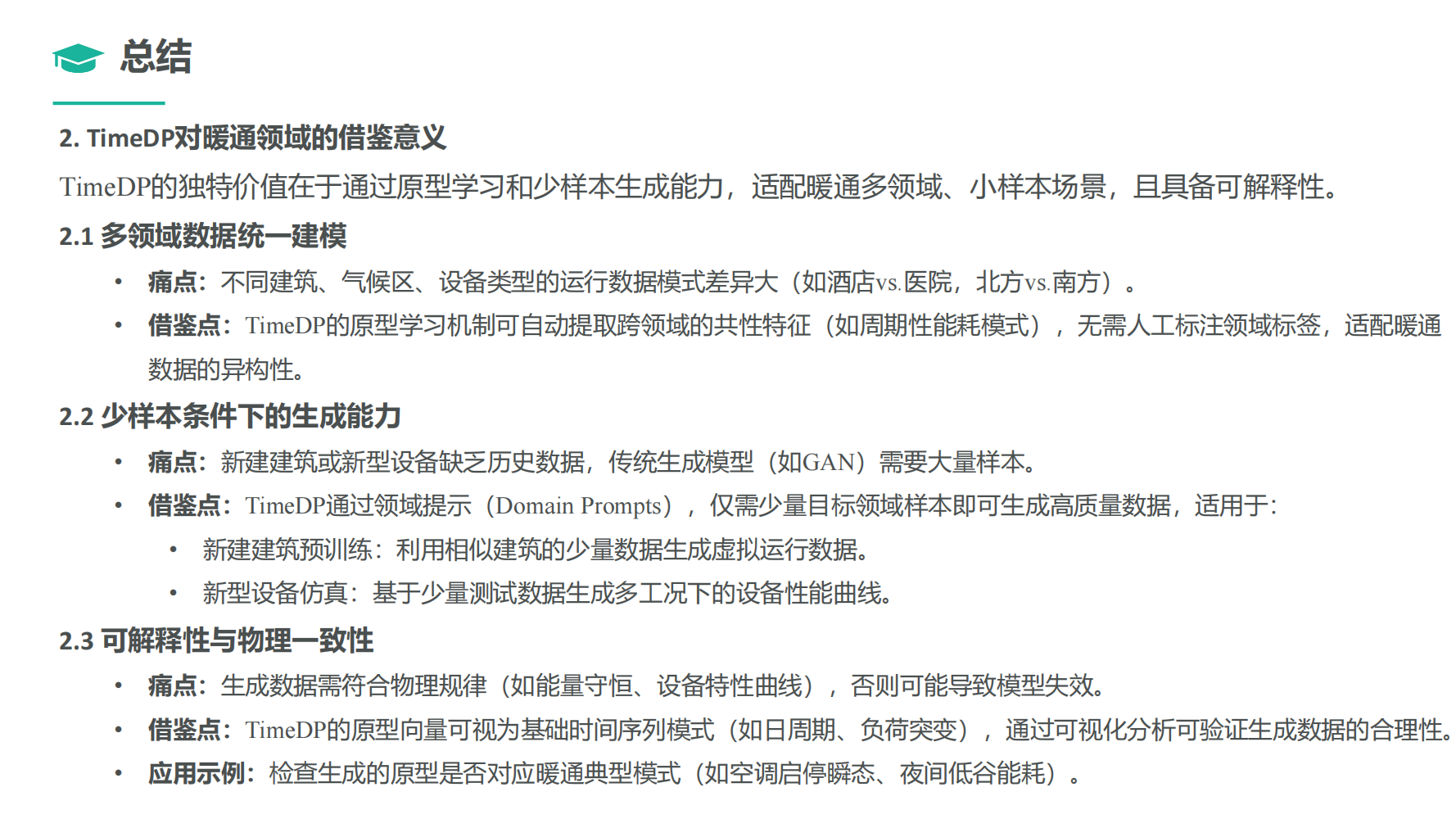
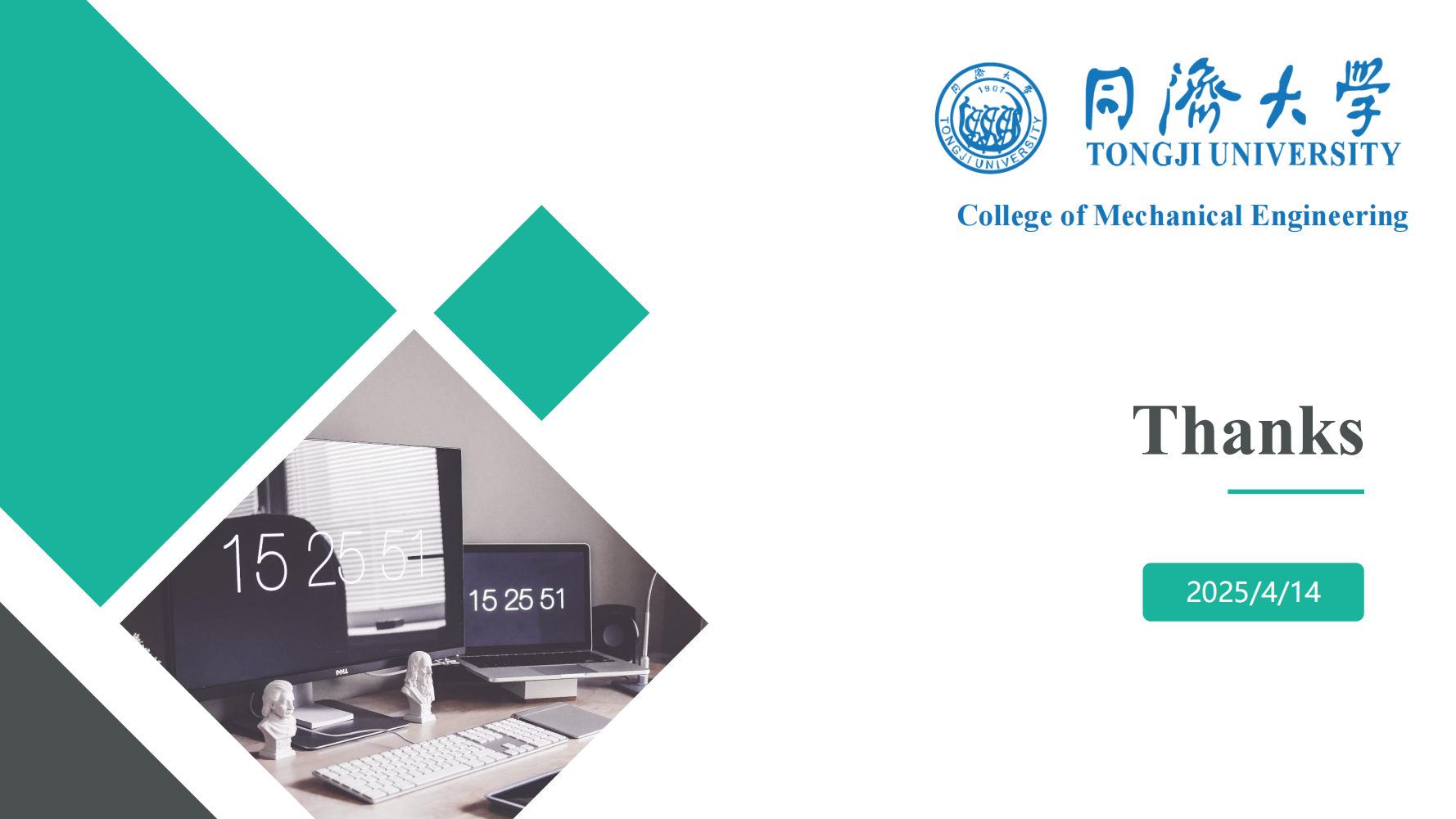
声明:本网站内容版权归许鹏教授课题组所有。未经本课题组授权不得转载、摘编或利用其它方式使用上述作品。
视频链接:张亚柯_基于领域提示学习生成多领域时间序列_哔哩哔哩_bilibili