文献来源: ,
文献链接: /BEFOREGAN.pdf
文献摘要:
Abstract
This study presents a pioneering approach in building energy forecasting by introducing a novel reformulation framework that transforms the forecasting task into an image inpainting problem. Based upon the fundamental notion that “forecasting is about generating data of the future”, we propose
BEForeGAN, an innovative deep generative approach for day-ahead
Building HVAC
Energy consumption
Forecasting based on multi-channel conditional
Generative
Adversarial
Networks (GANs) with U-Net generators. Our method is evaluated using 96,360 hourly HVAC energy consumption records from 11 buildings, demonstrating significant accuracy improvements of 17%∼76% and a substantial variability reduction of 3%∼96% compared to a suite of conventional and deep learning benchmark models across individual-building and zero-shot cross-building forecasting tasks. Notably, BEForeGAN exhibits robustness to noisy inputs, with an increase below 3% in Coefficient of Variation of Root Mean Square Error (CV-RMSE) for each 10% noise increment. This study addresses critical gaps in existing literature by showcasing the untapped potential of GANs as standalone forecasters, advocating for further exploration of two-dimensional (2D) GAN-based methods in building energy forecasting, and emphasising the need for more studies focusing on cross-building forecasting tasks. In conclusion, our findings underscore the transformative impact of GANs in revolutionising building energy forecasting practices, paving the way for enhanced energy-efficient building management and beyond.
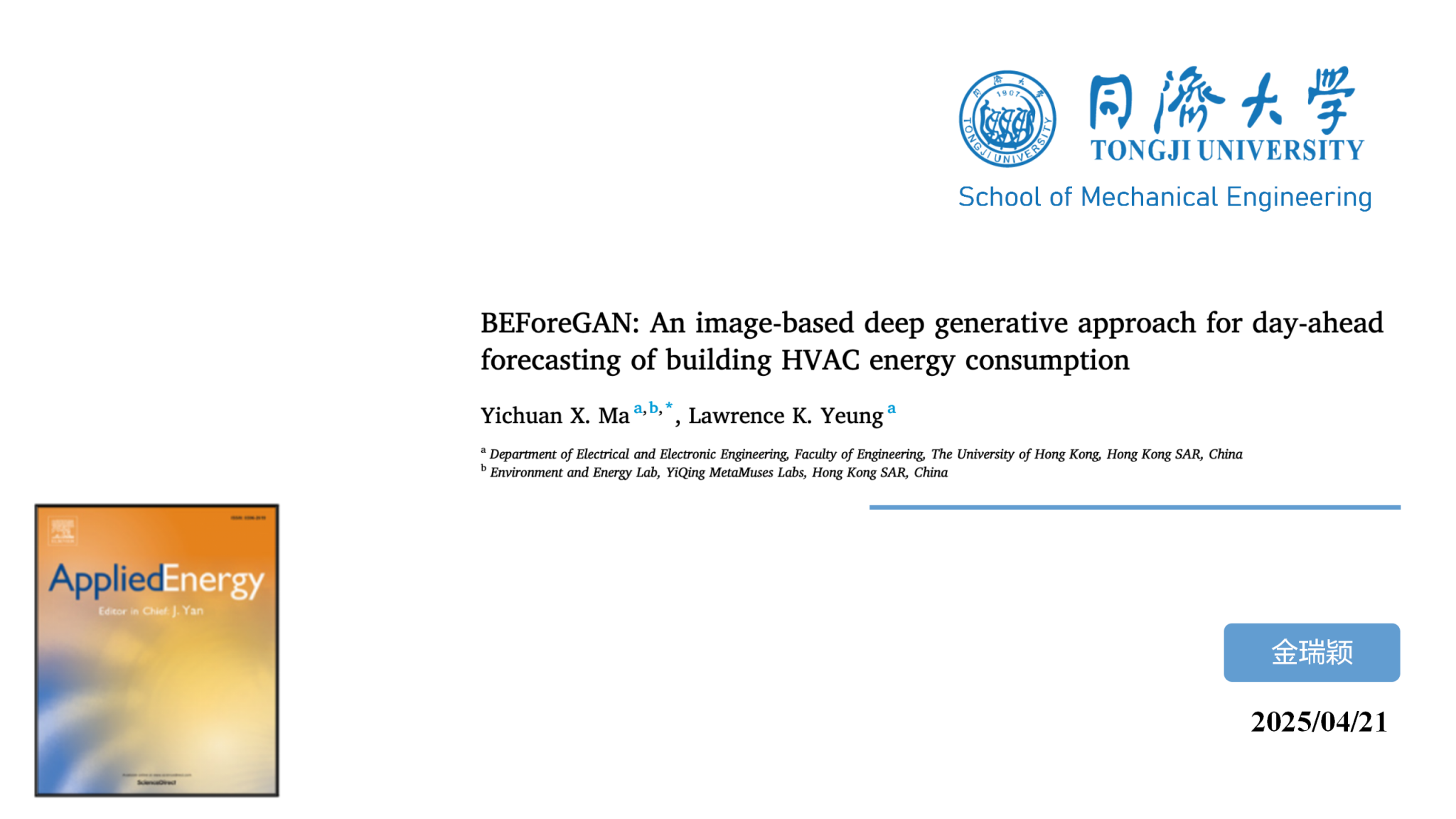
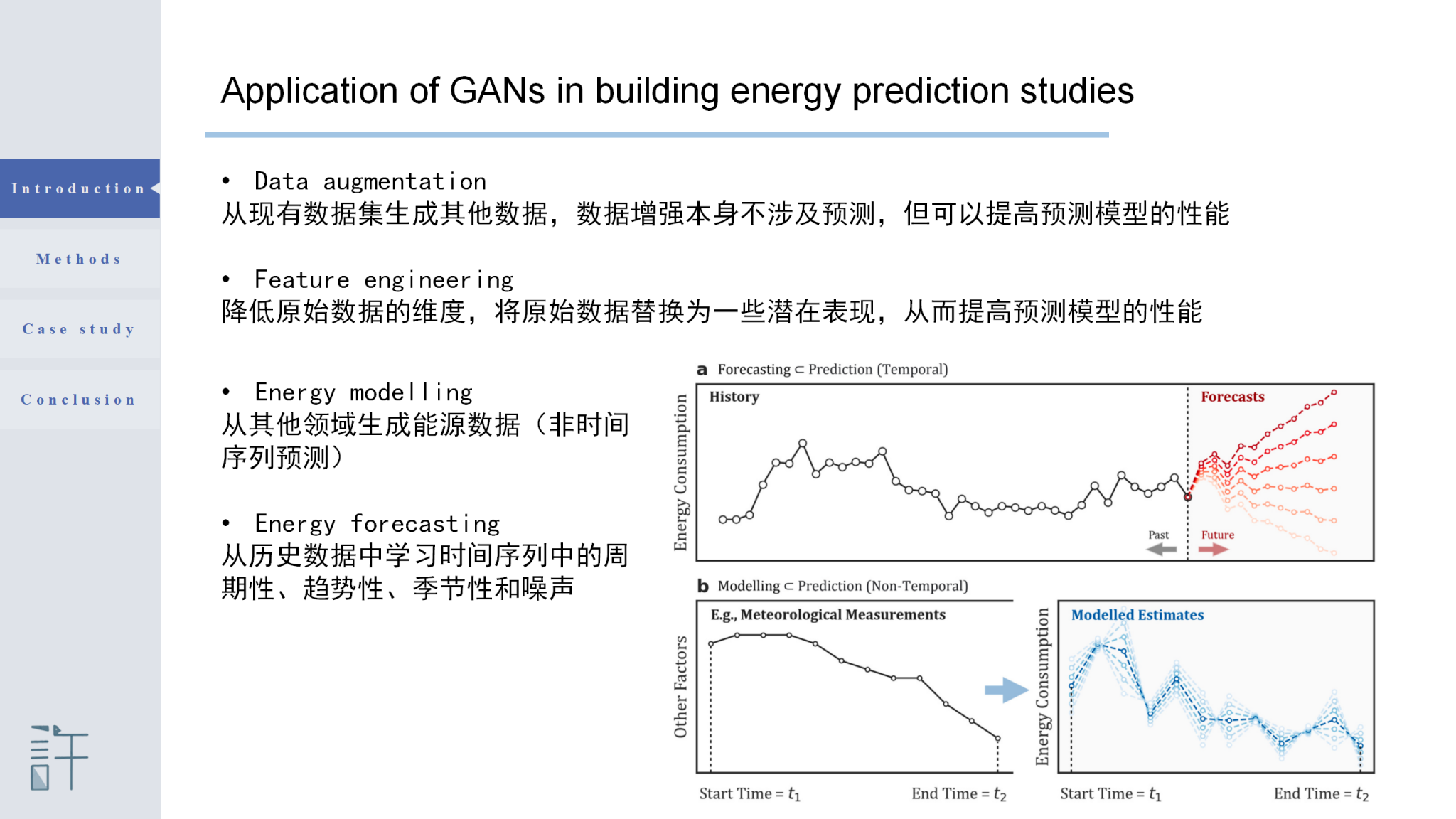
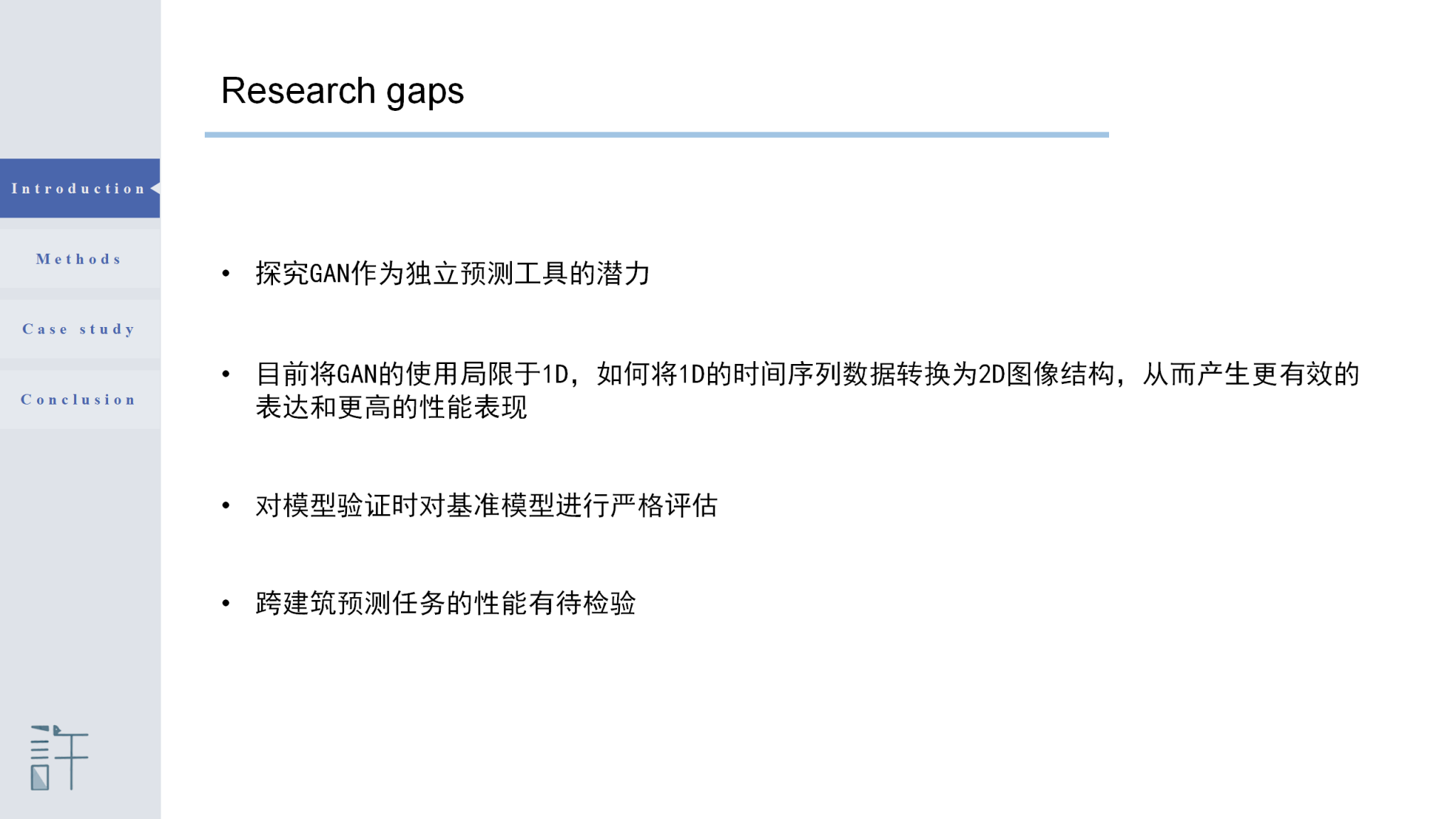
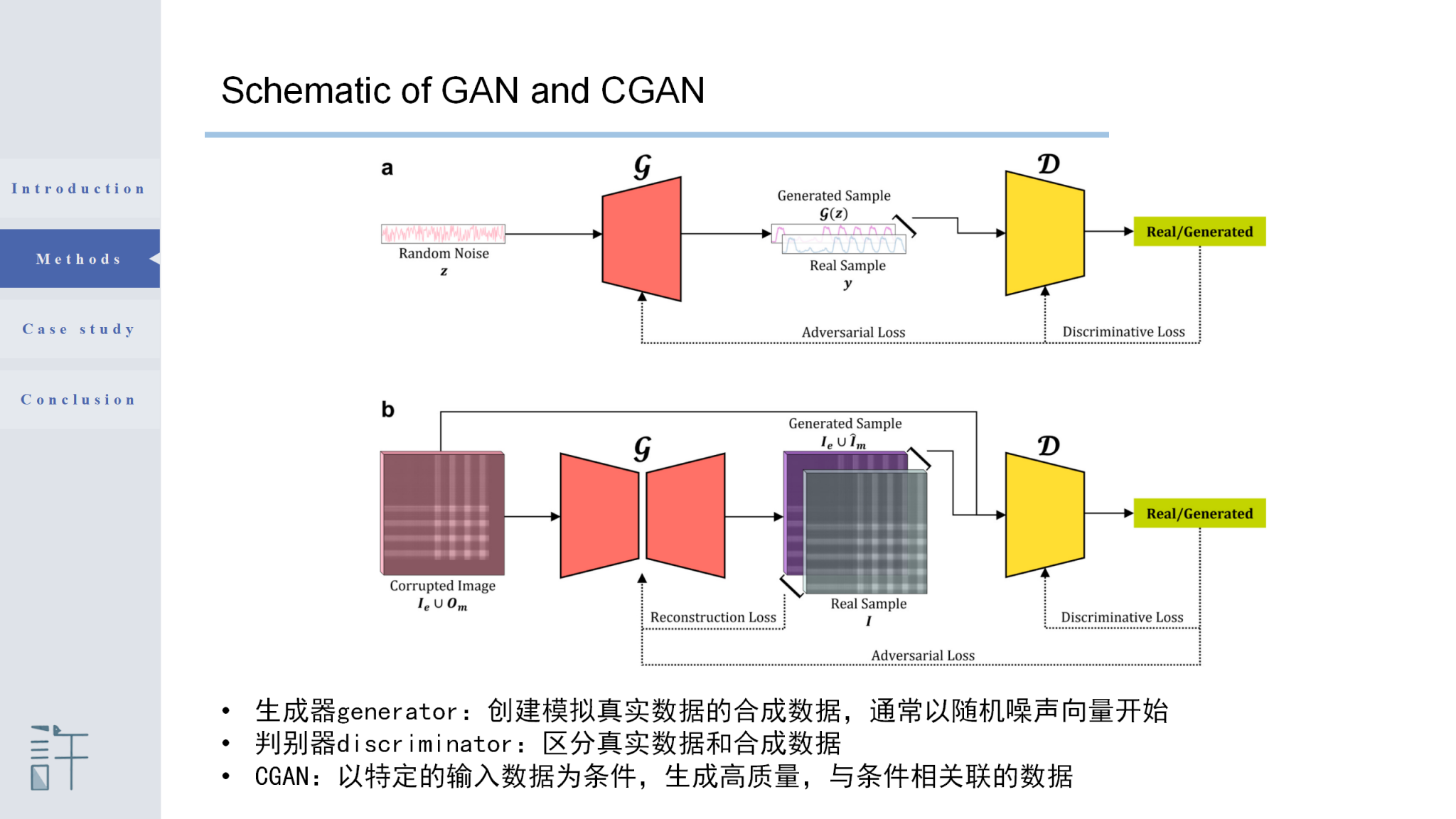
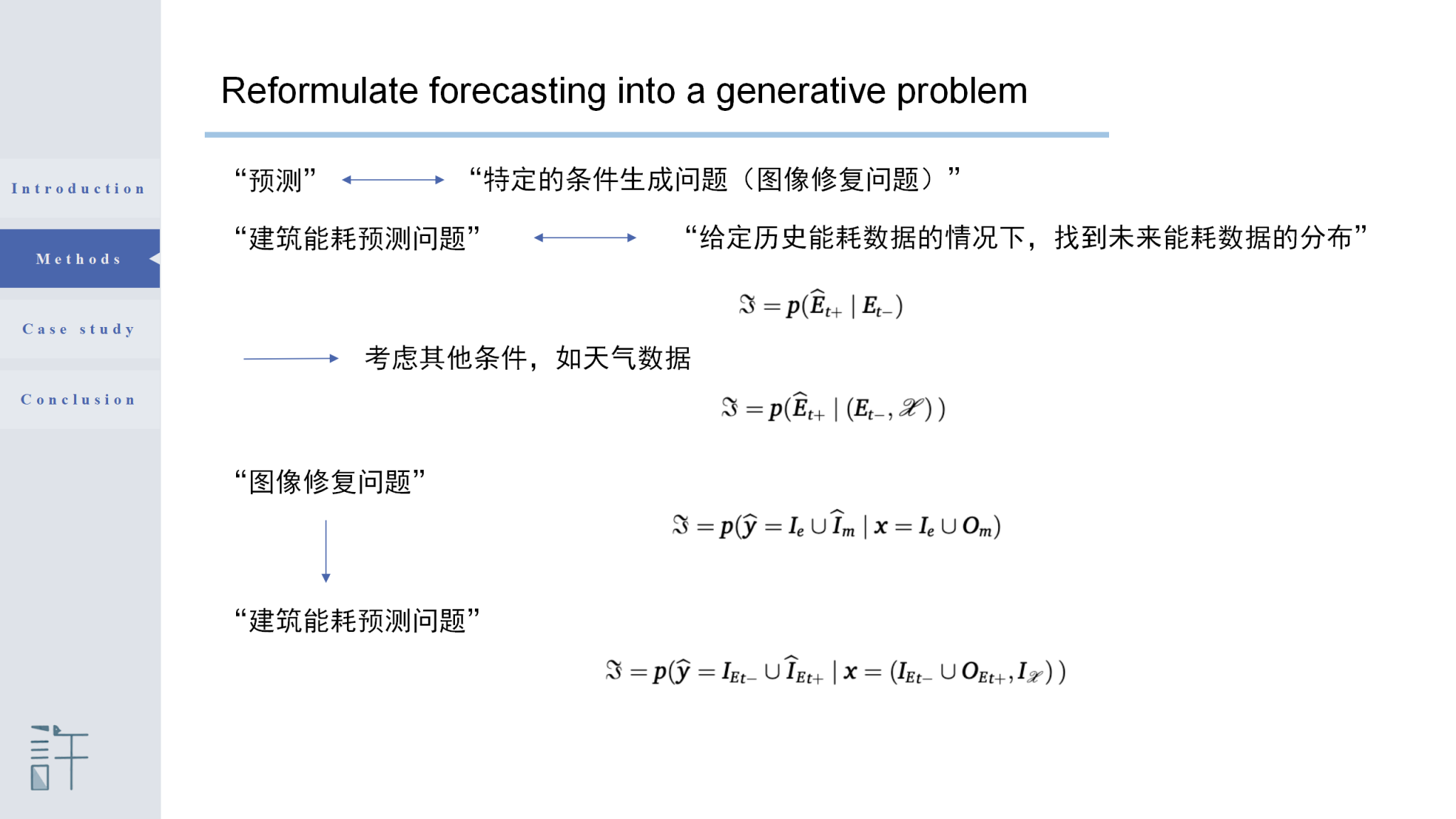
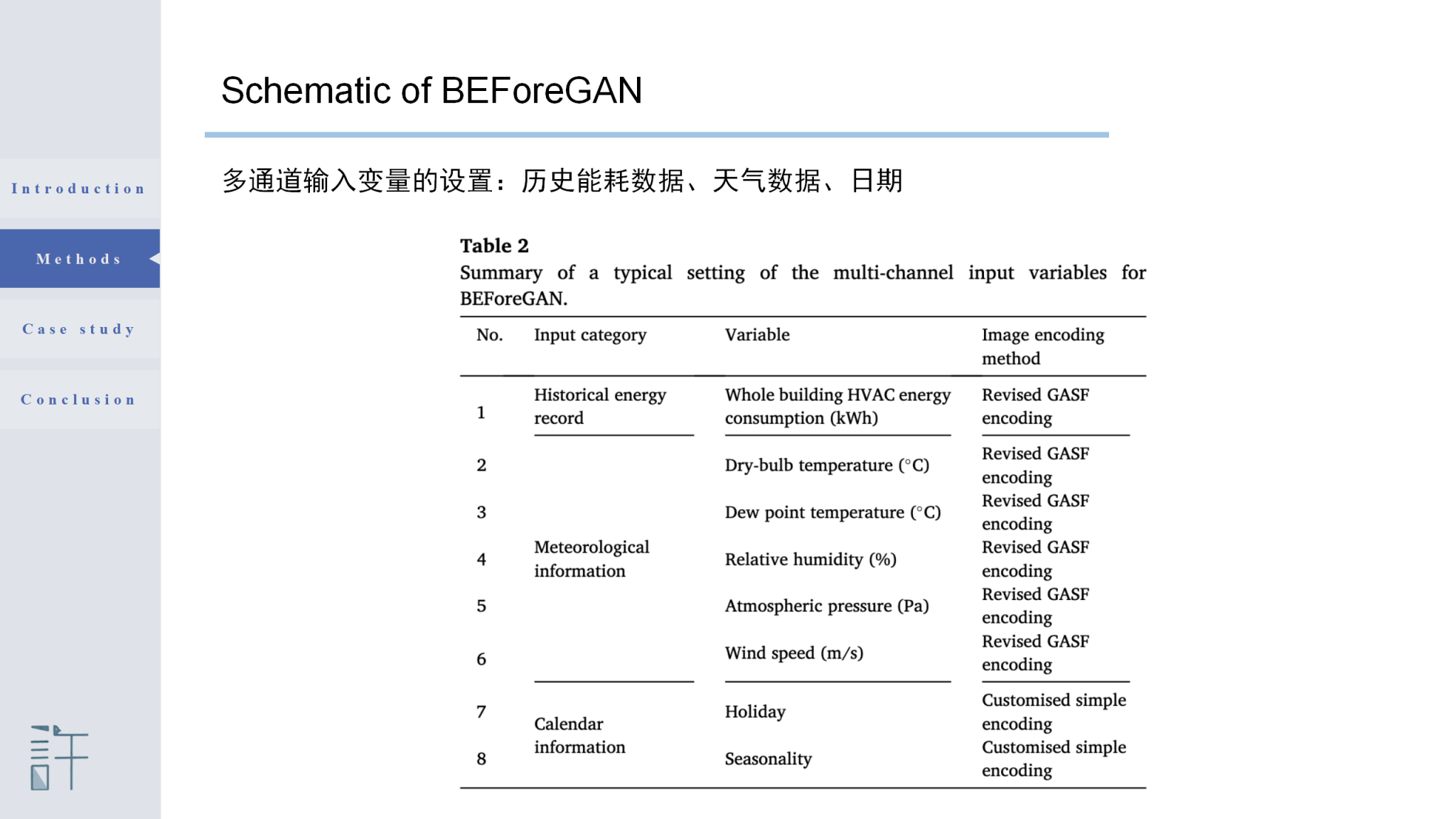
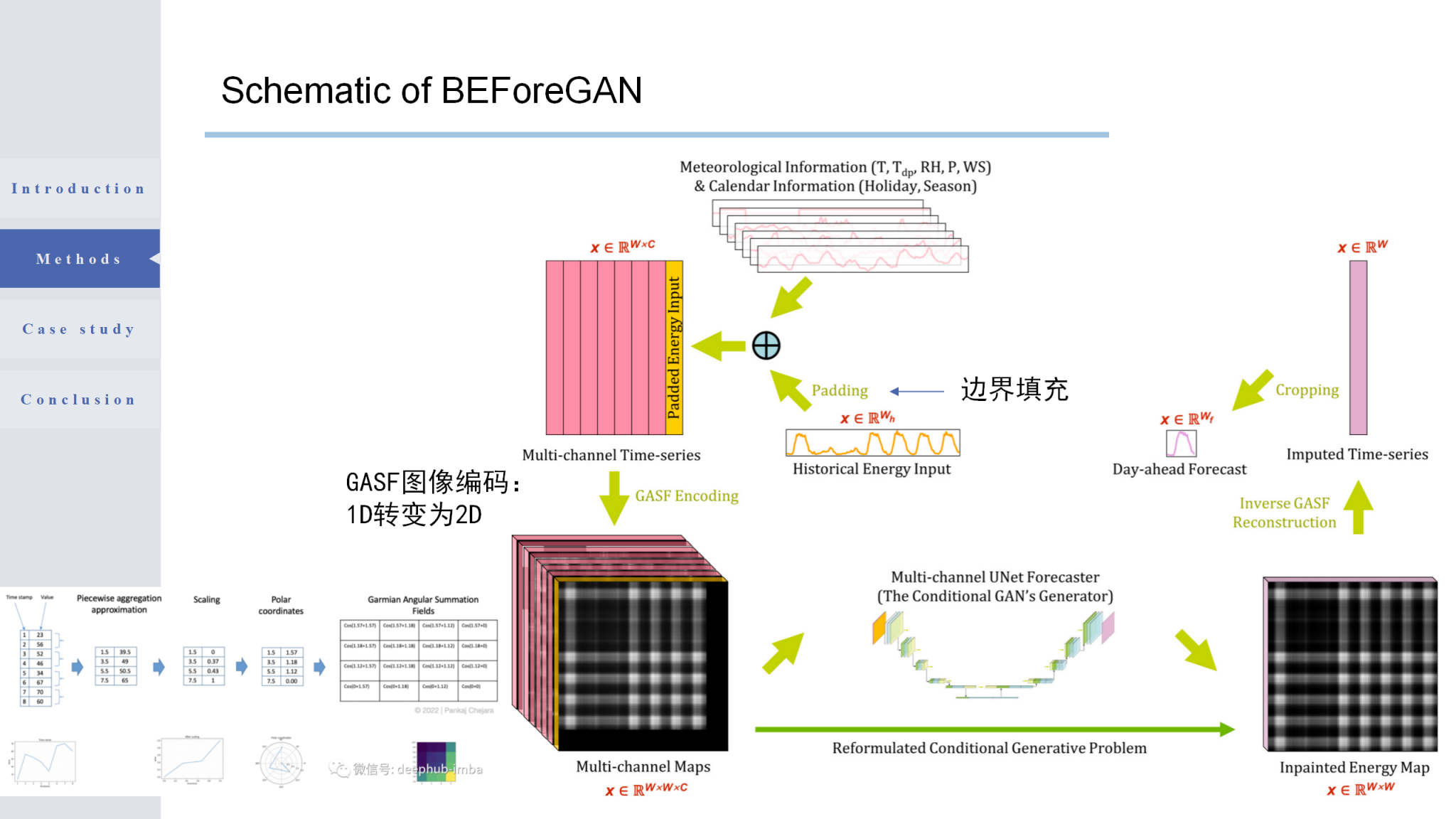
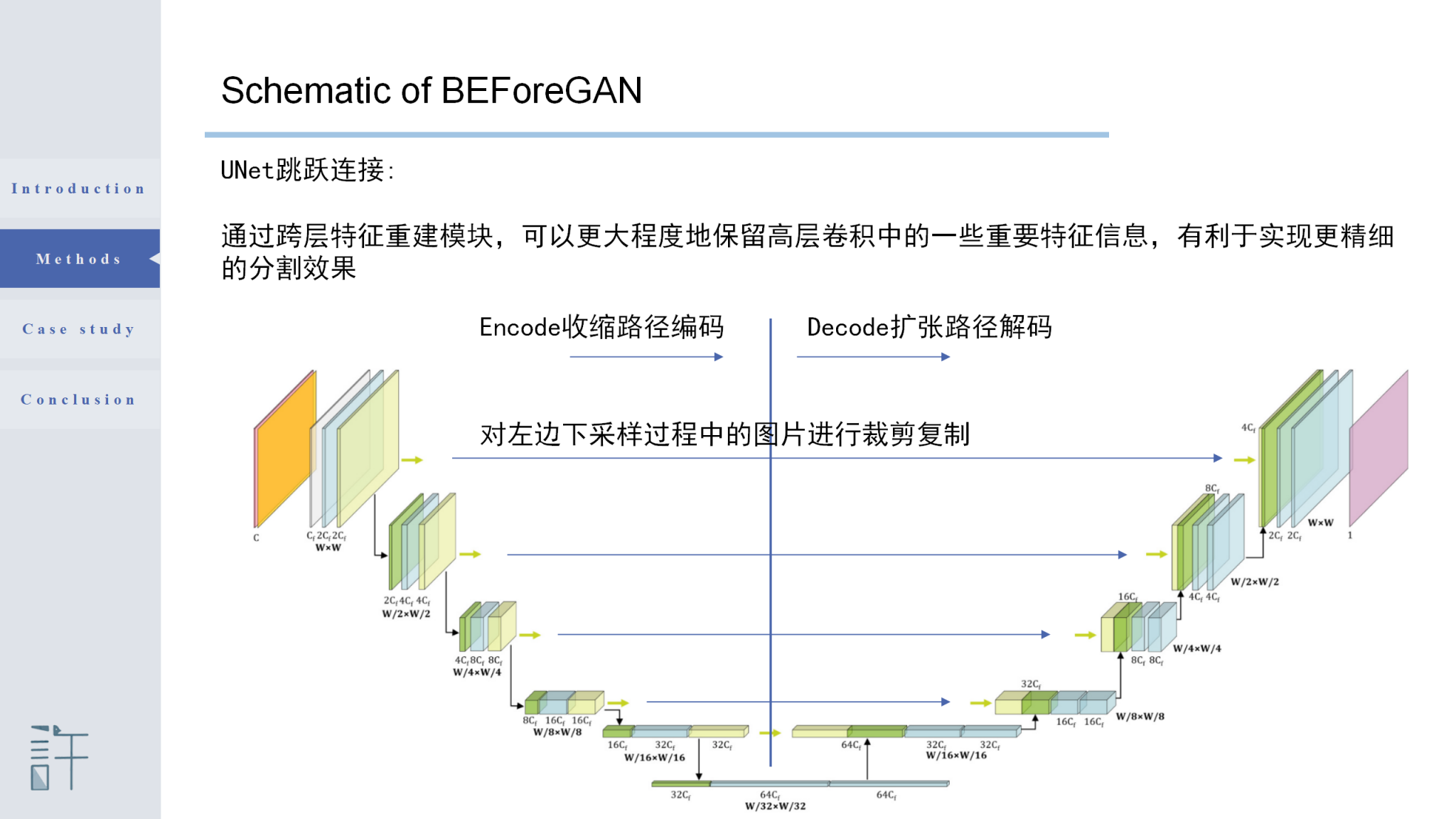
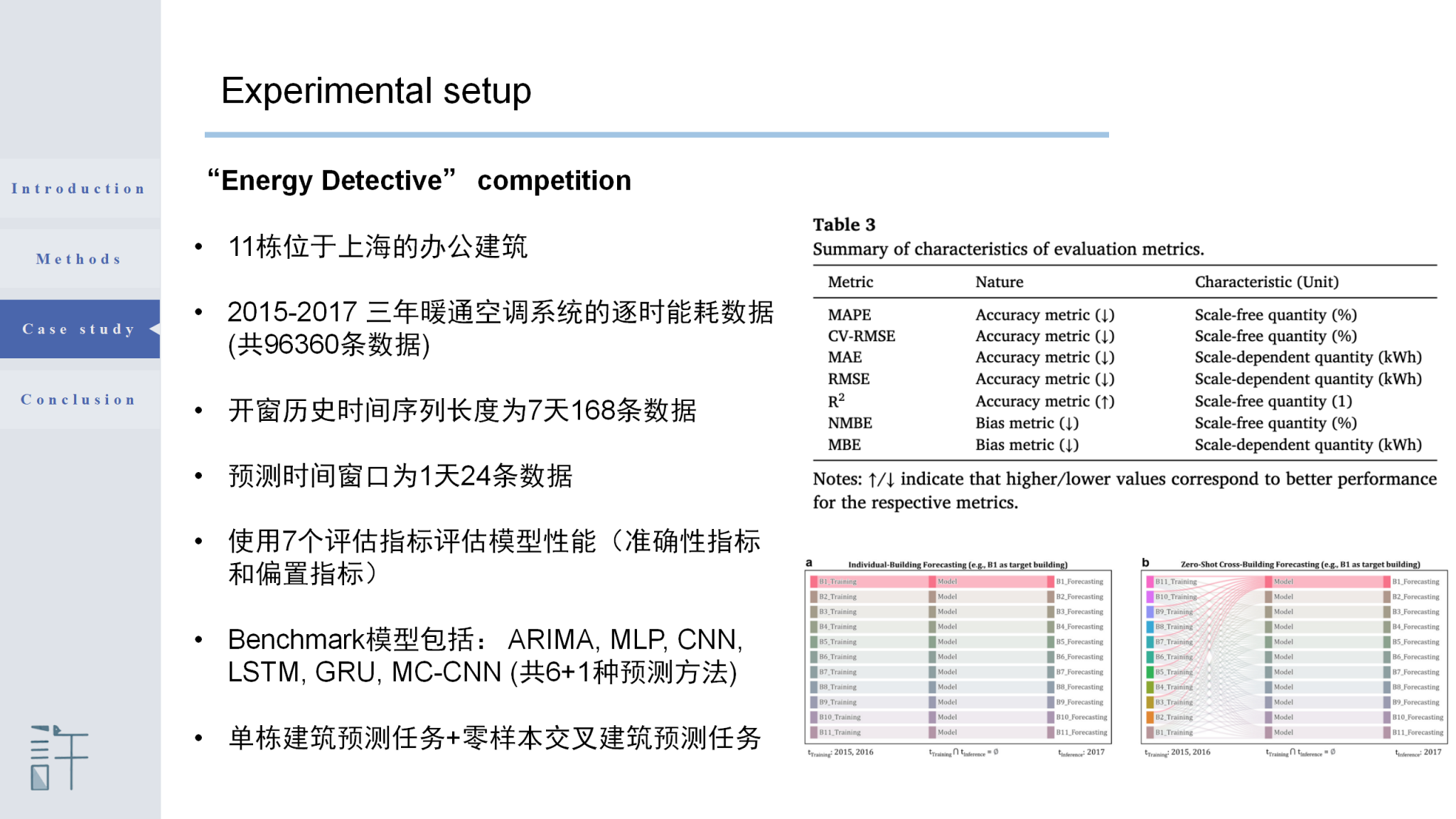
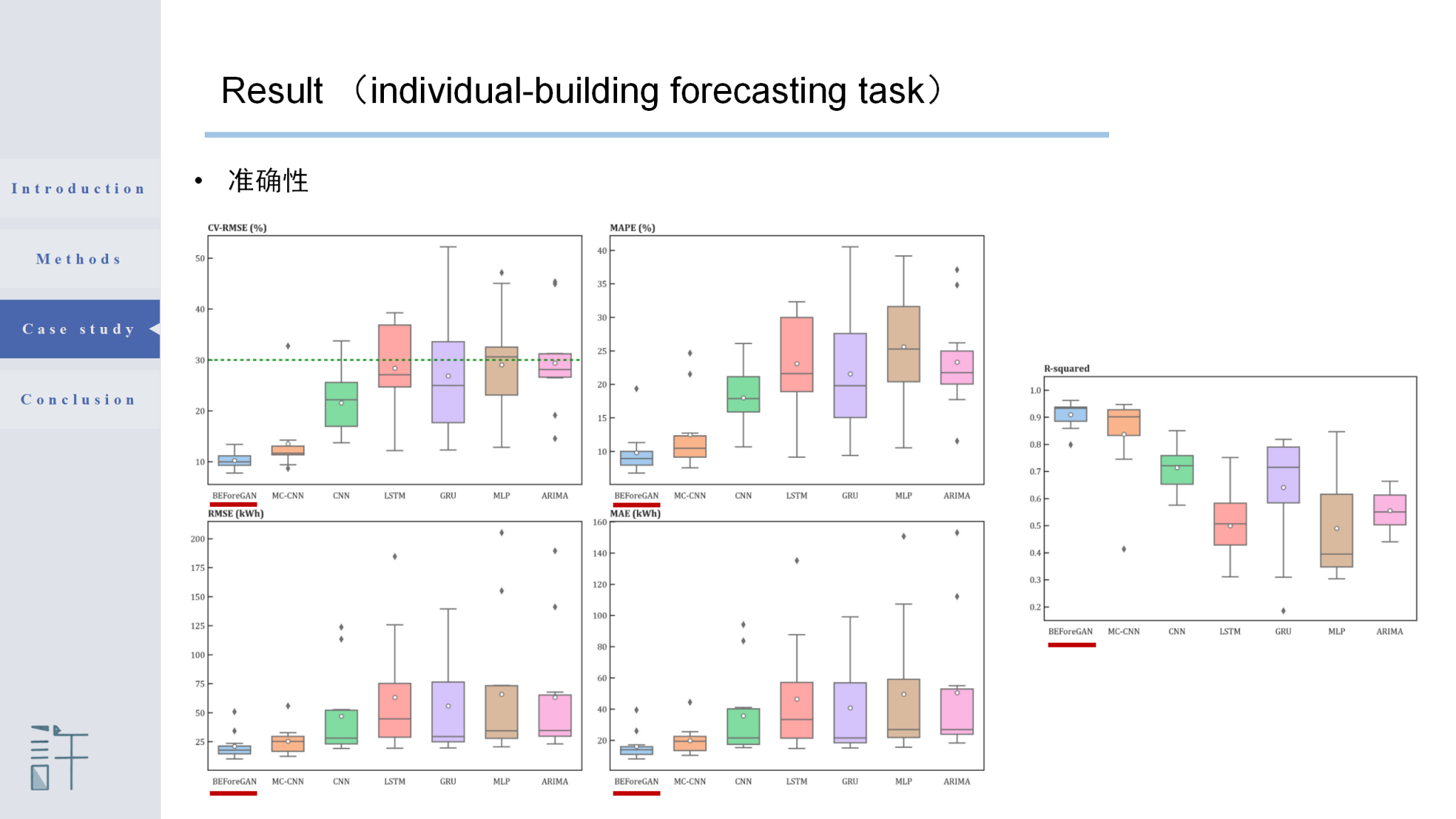
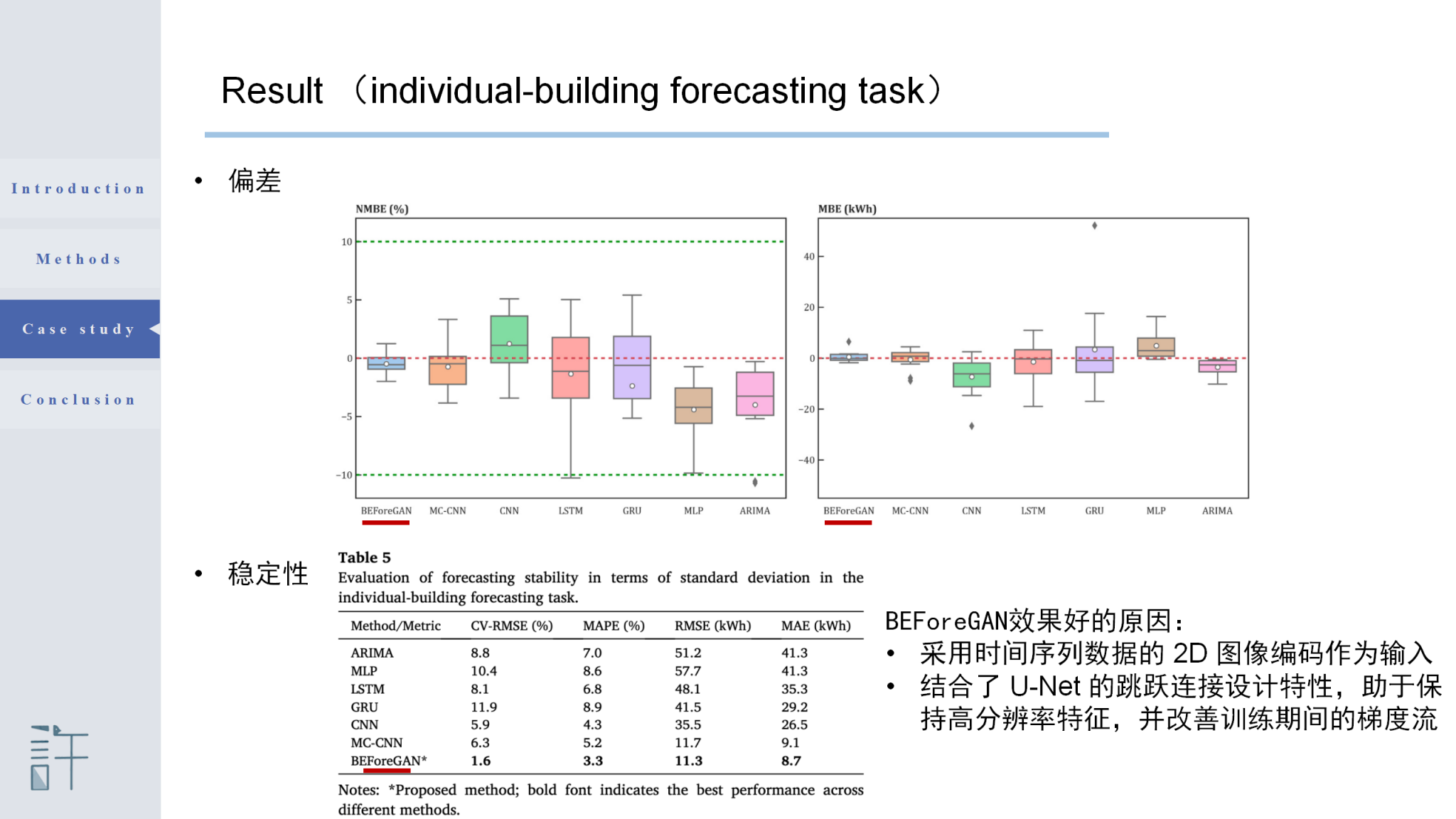
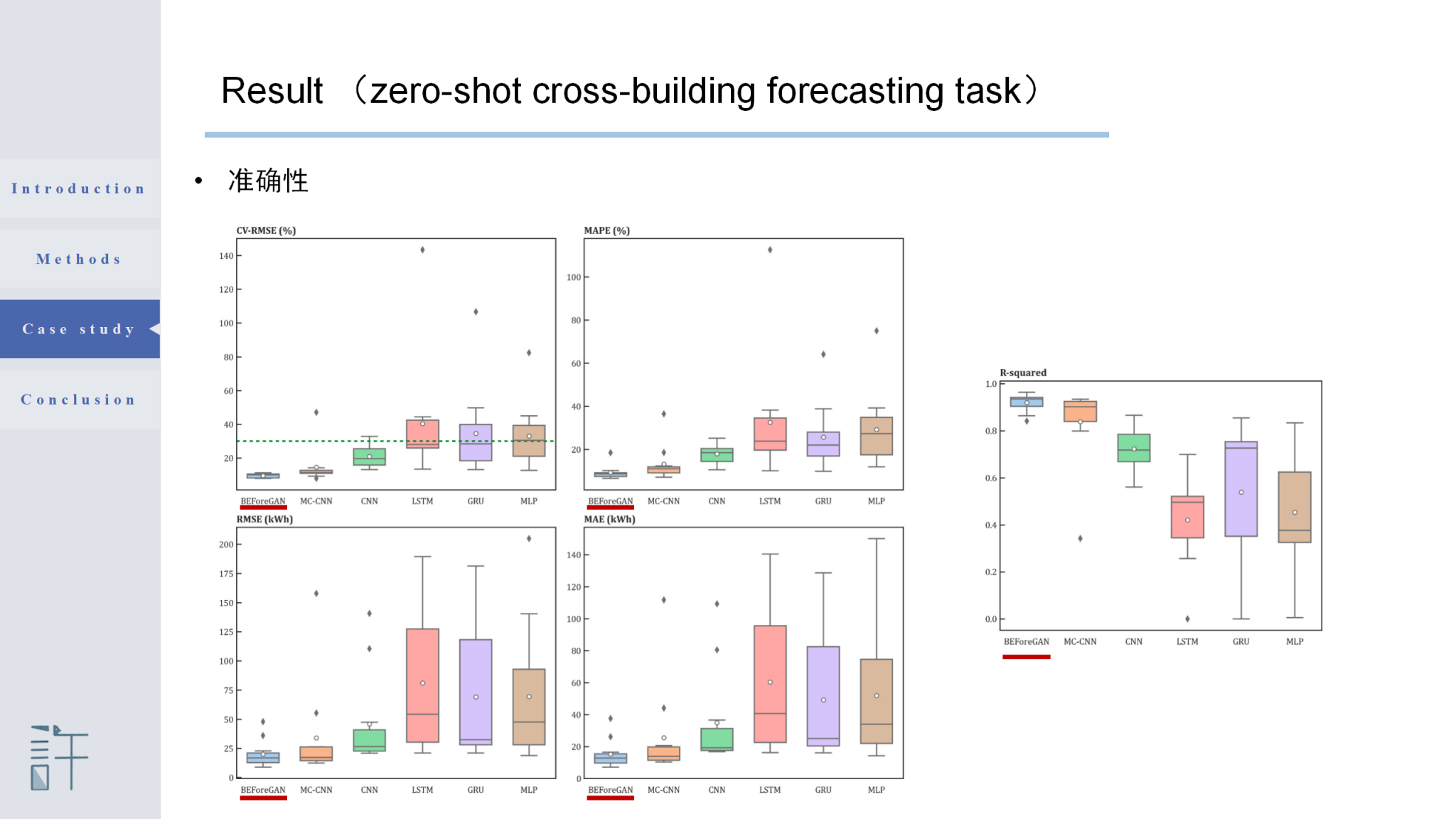
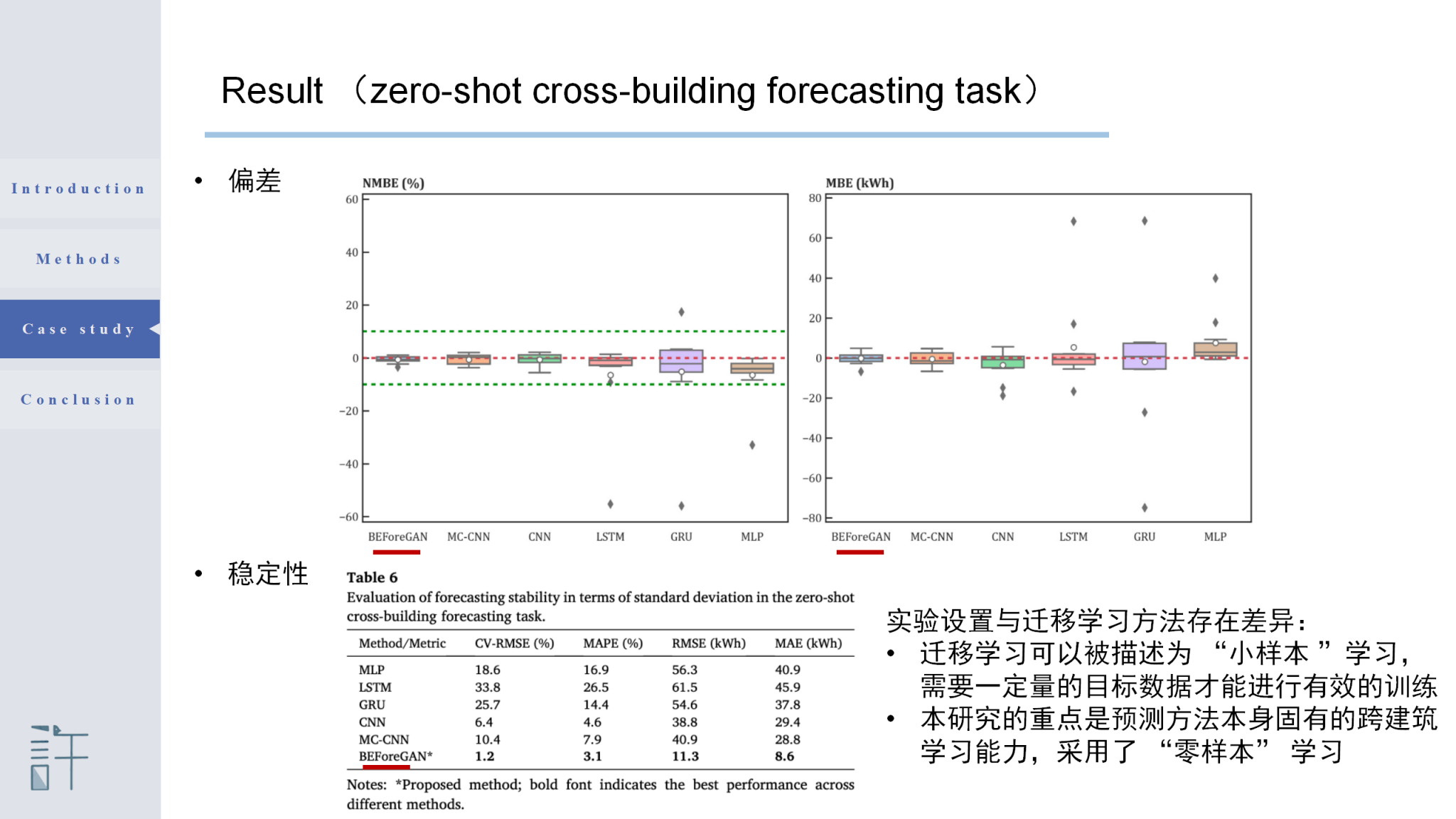
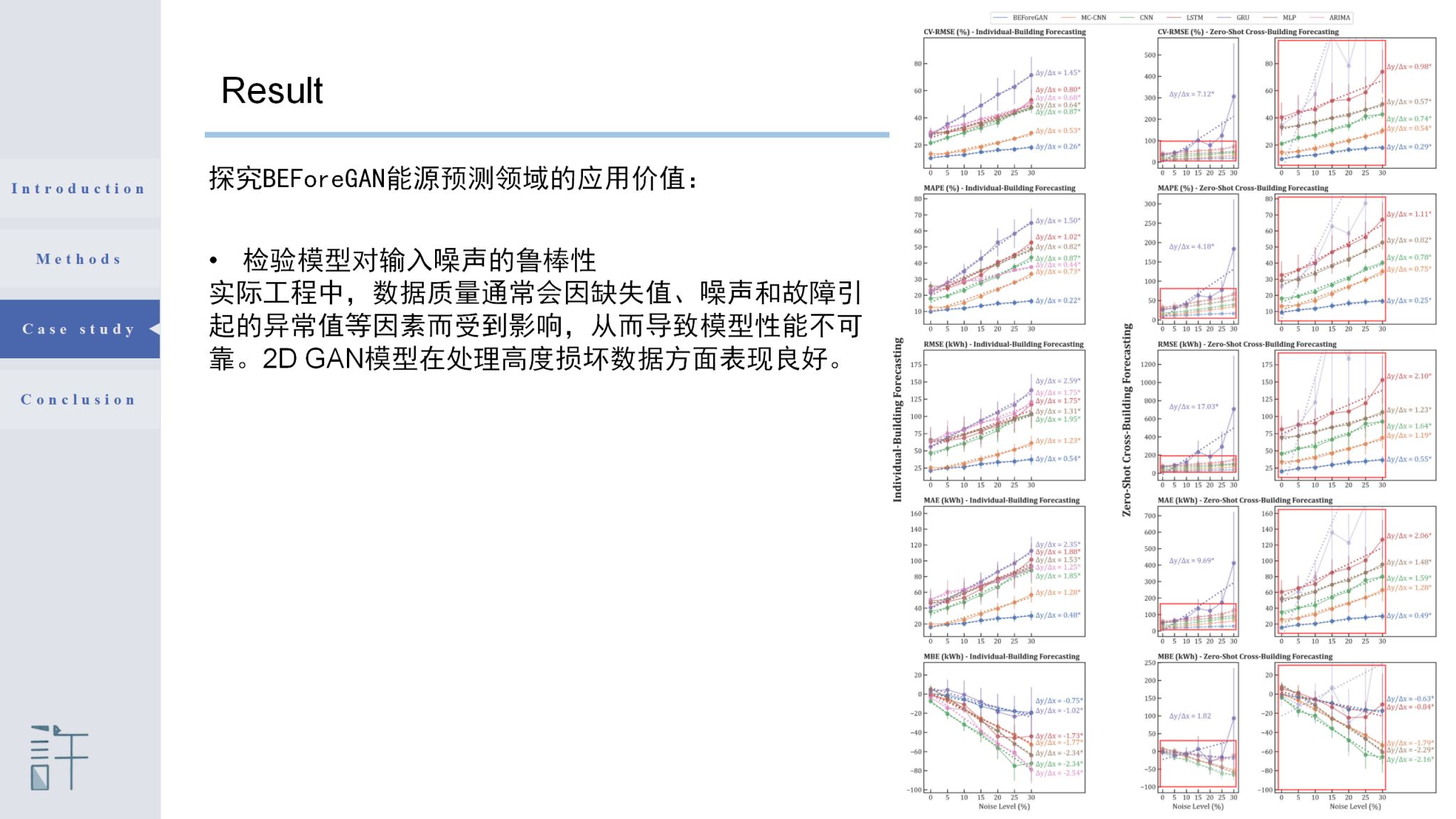
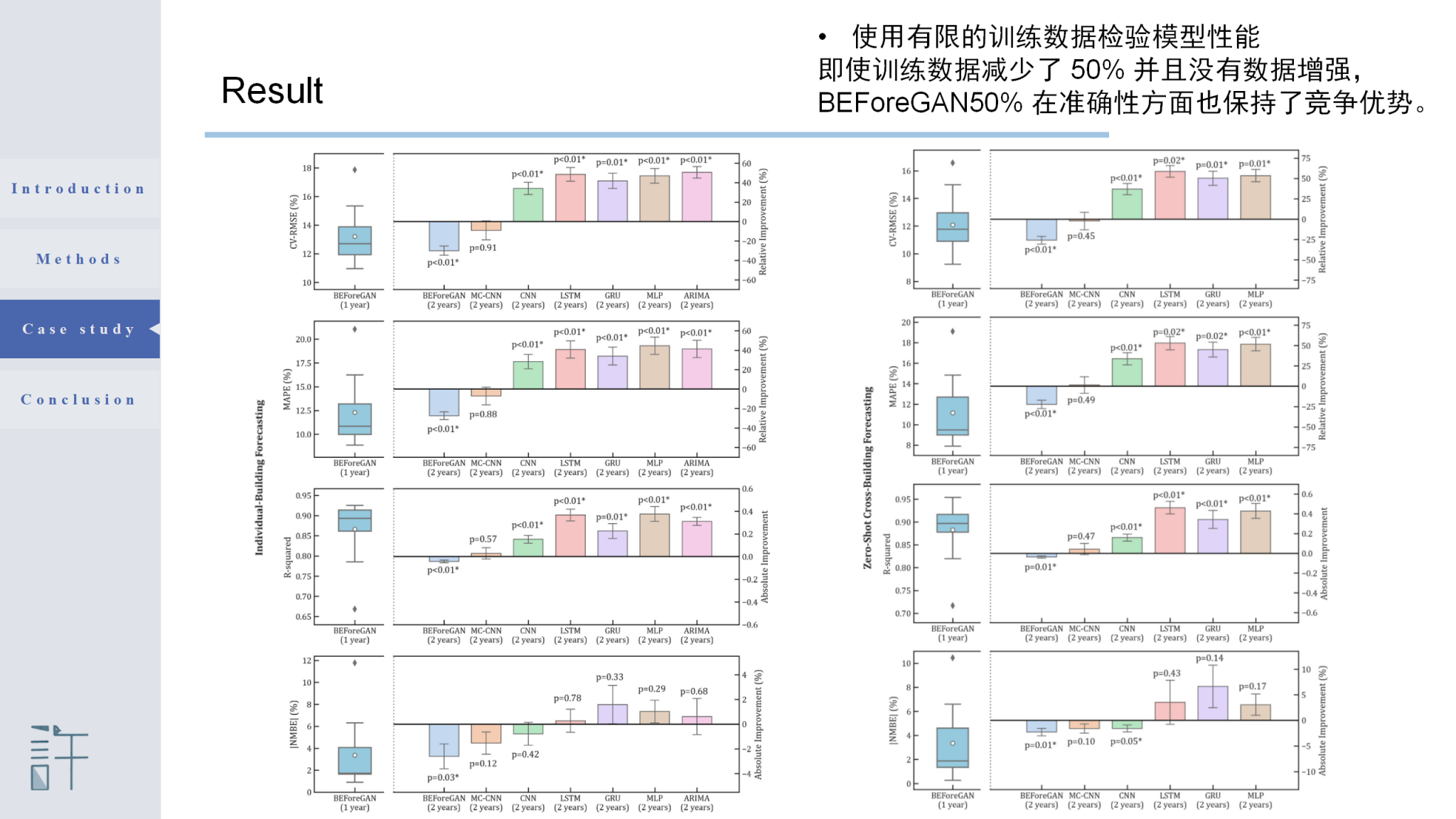
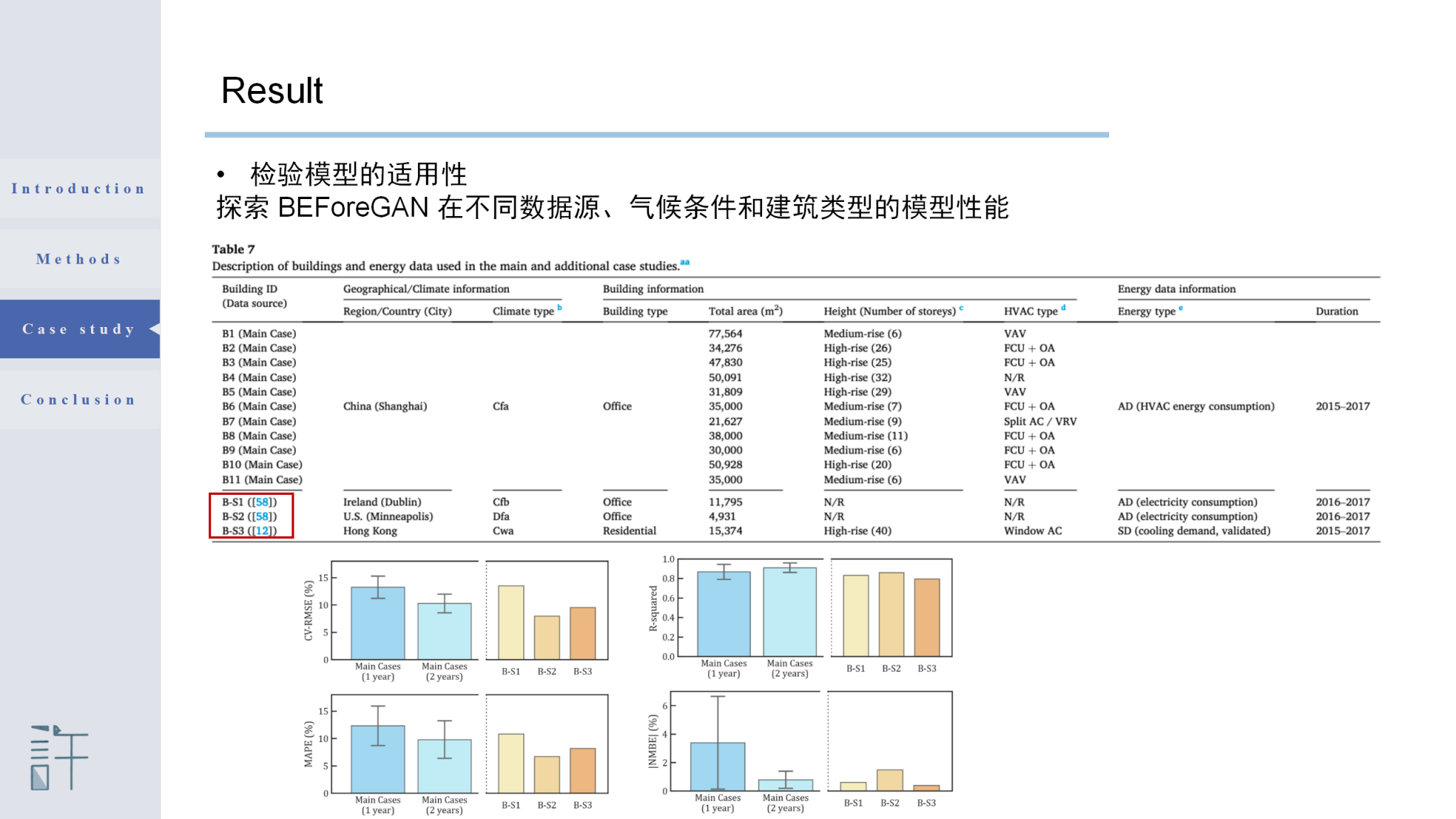
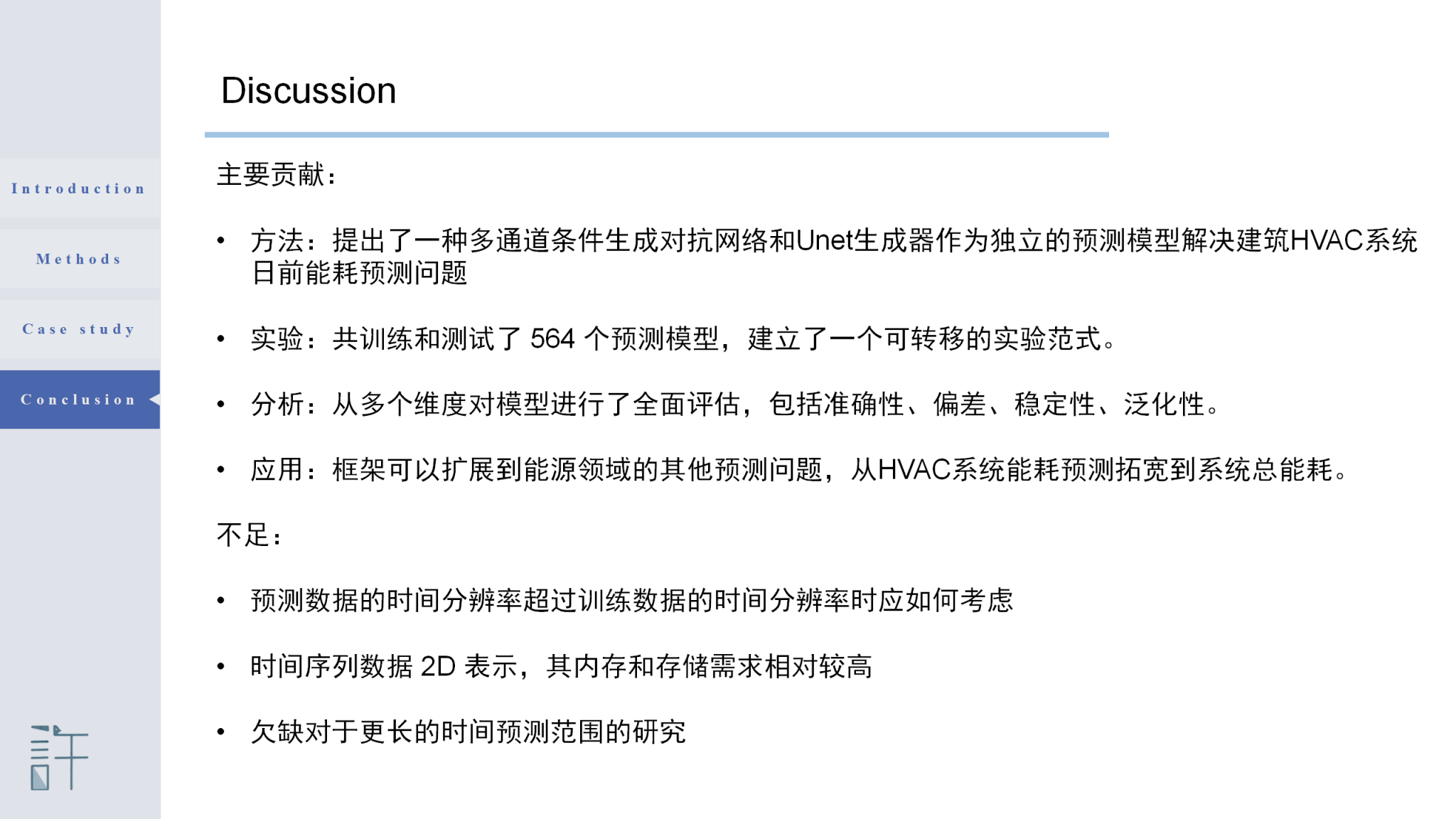
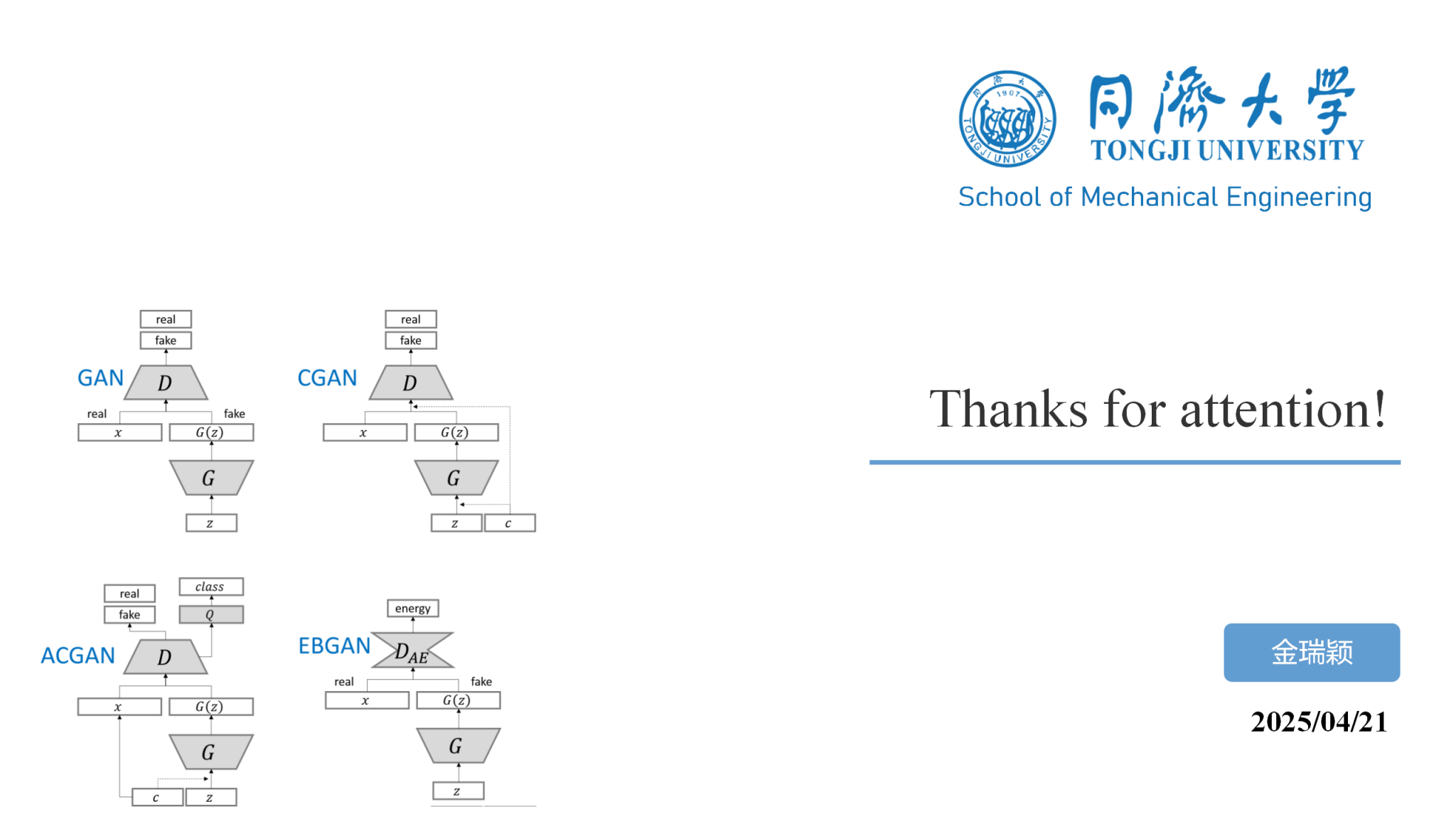
声明:本网站内容版权归许鹏教授课题组所有。未经本课题组授权不得转载、摘编或利用其它方式使用上述作品。
视频链接:金瑞颖_BEForeGAN:一种基于图像的深度生成方法,用于建筑物暖通空调能耗的提前预测_哔哩哔哩_bilibili