文献来源:
[1]Jason Wei;Xuezhi Wang;Dale Schuurmans;Maarten Bosma;Ed Chi;Quoc Le;Denny Zhou.Chain of Thought Prompting Elicits Reasoning in Large Language Models.Google Research,2022/WXFX_Chain-of-Thought_Prompting_Elicits_Reasoning_in_Large_Language_Models.pdf
[2]Xuezhi Wang;Jason Wei;Dale Schuurmans;Quoc Le;Ed H. Chi;Sharan Narang;Aakanksha Chowdhery;Denny Zhou.Sef-Consistensy Improves Chain Of Thought Reasoning In Language Models.Google Research,2023/WXFX_Self-Consistency_Improves_Chain_of_Thought_Reasoning_in_Language_Models.pdf
文章摘要:
[1]Although scaling up language model size has reliably improved performance on a range of NLP tasks, even the largest models currently struggle with certain reasoning tasks such as math word problems, symbolic manipulation, and commonsense reasoning. This paper explores the ability of language models to generate a coherent chain of thought—a series of short sentences that mimic the reasoning process a person might have when responding to a question. Experiments show that inducing a chain of thought via prompting can enable suffificiently large language models to better perform reasoning tasks that otherwise have flat scaling curves. When combined with the 540B parameter PaLM model, chain of thought prompting achieves new state of the art of 58.1% on the GSM8K benchmark of math word problems.
[2]Chain-of-thought prompting combined with pre-trained large language models has achieved encouraging results on complex reasoning tasks. In this paper, we propose a new decoding strategy, self-consistency, to replace the naive greedy decoding used in chain-of-thought prompting. It fifirst samples a diverse set of reasoning paths instead of only taking the greedy one, and then selects the most consistent answer by marginalizing out the sampled reasoning paths. Self-consistency leverages the intuition that a complex reasoning problem typically admits multiple different ways of thinking leading to its unique correct answer. Our extensive empirical evaluation shows that self-consistency boosts the performance of chain-of-thought prompting with a striking margin on a range of popular arithmetic and commonsense reasoning benchmarks, including GSM8K (+17.9%), SVAMP (+11.0%), AQuA (+12.2%), StrategyQA (+6.4%) and ARC-challenge (+3.9%).
分享内容:
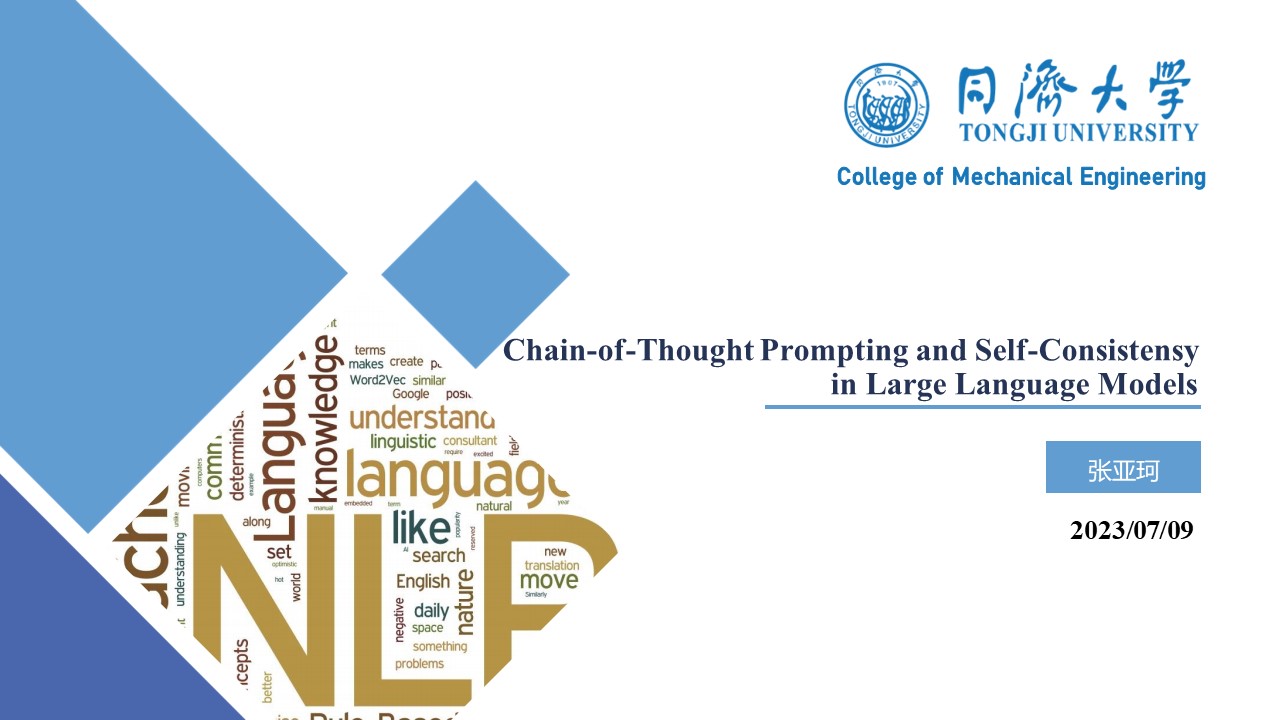
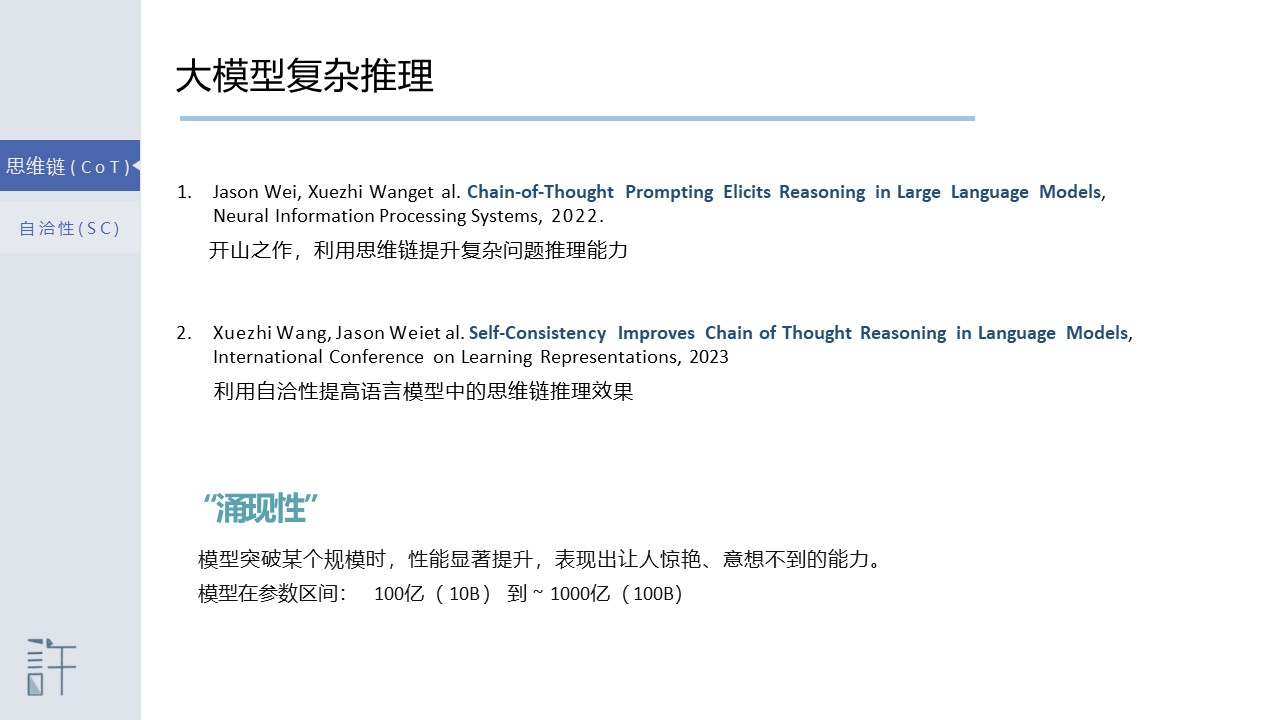
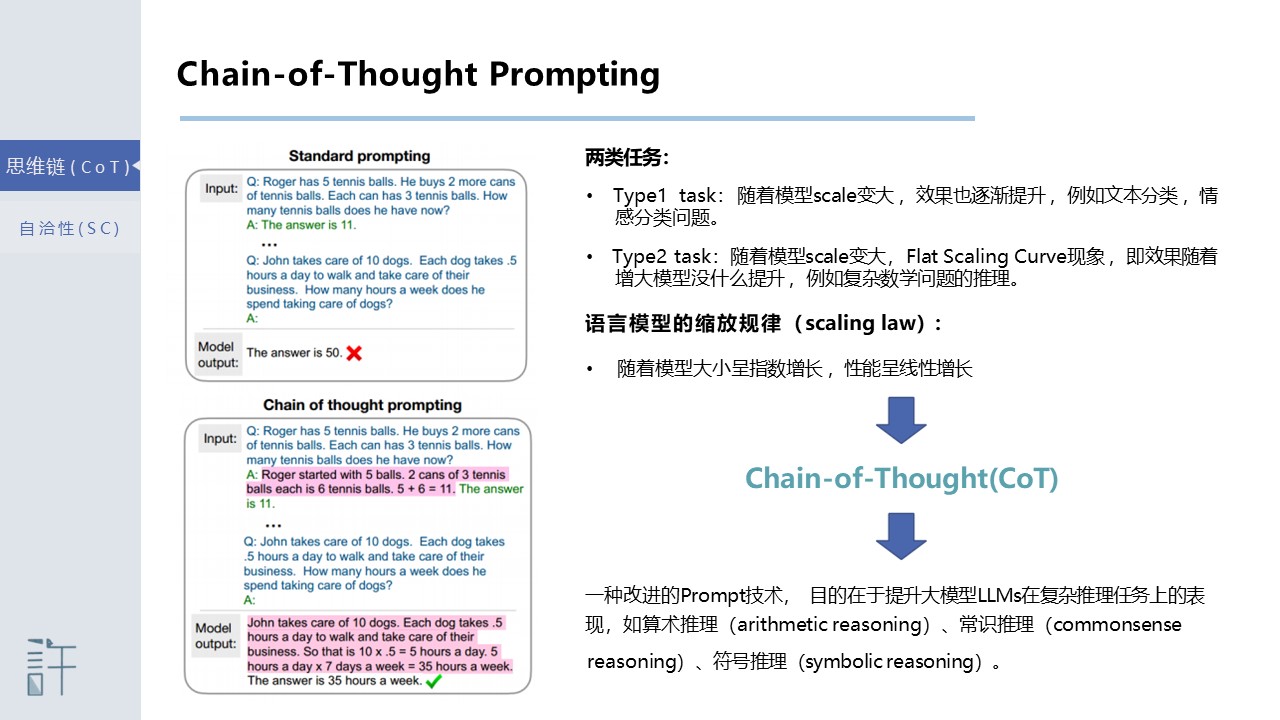
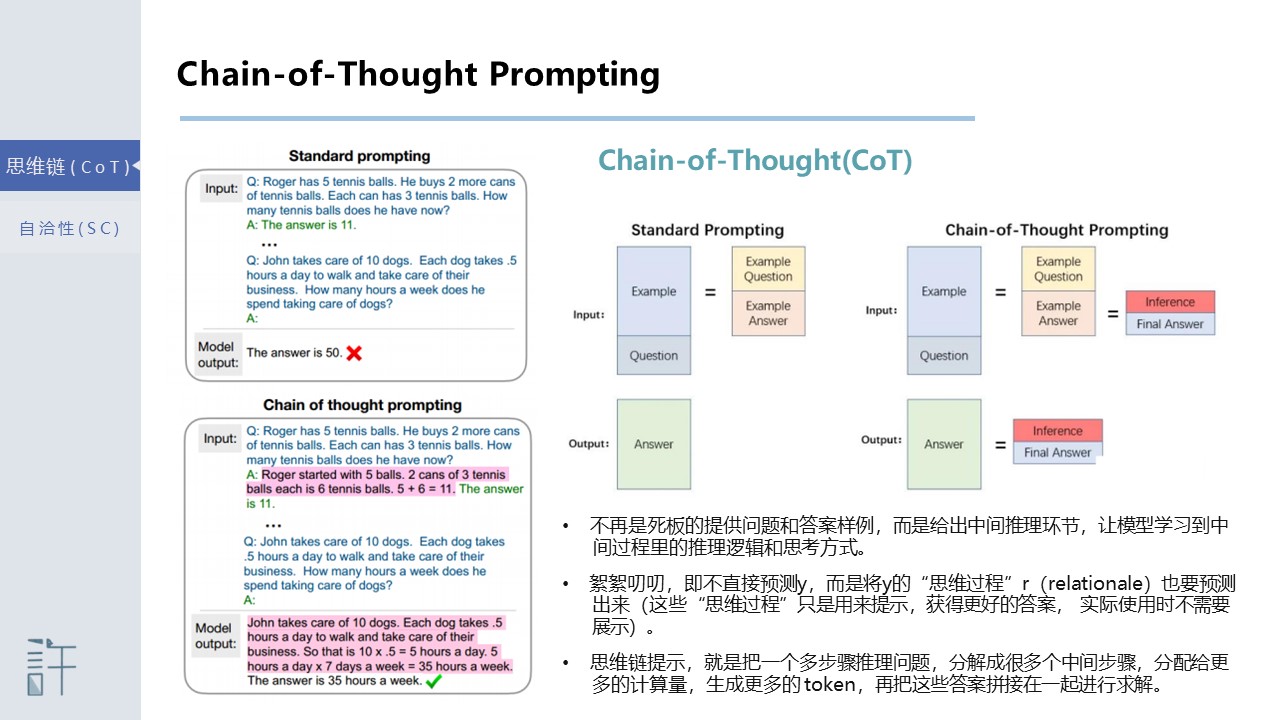
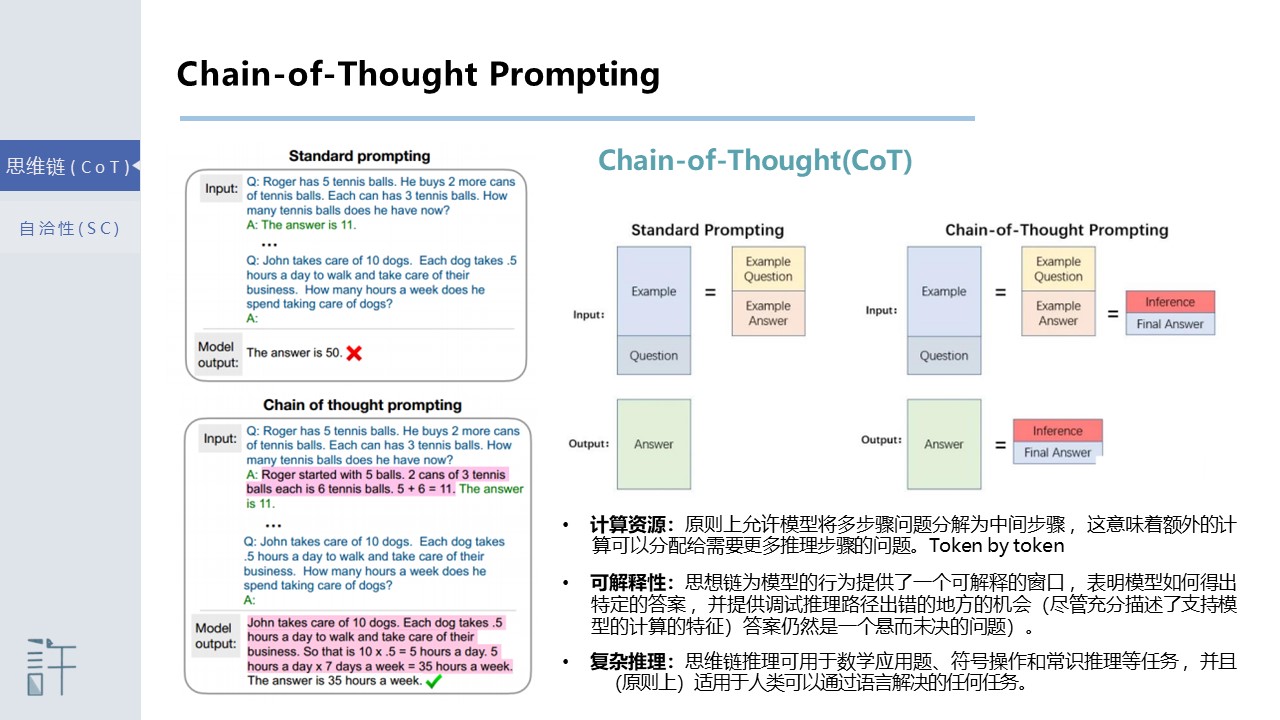
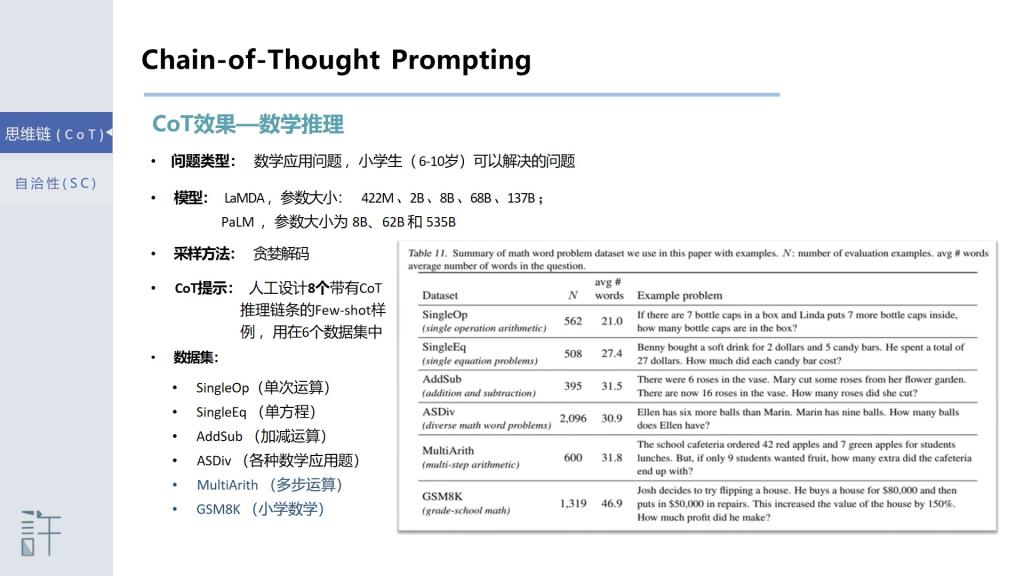
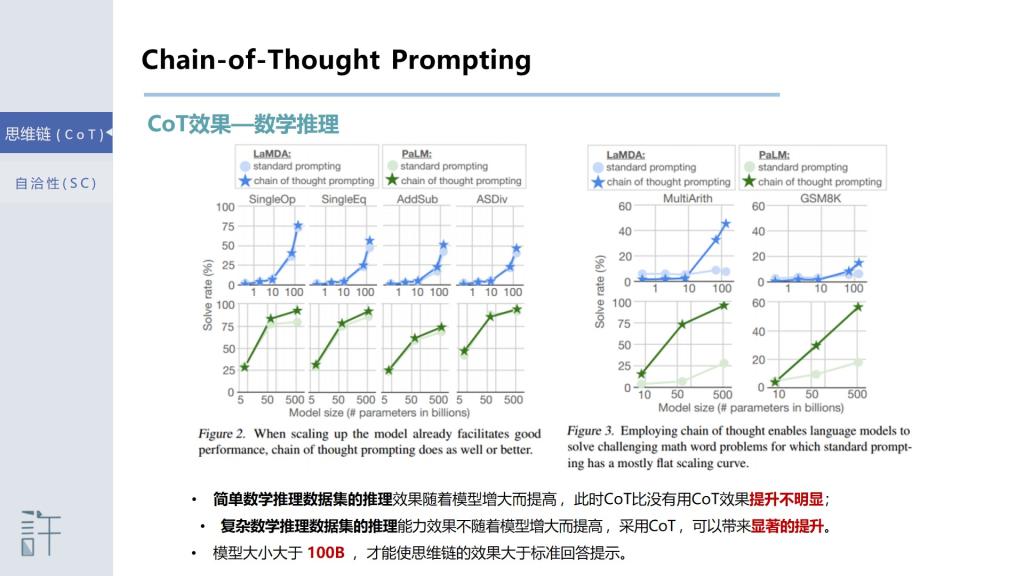
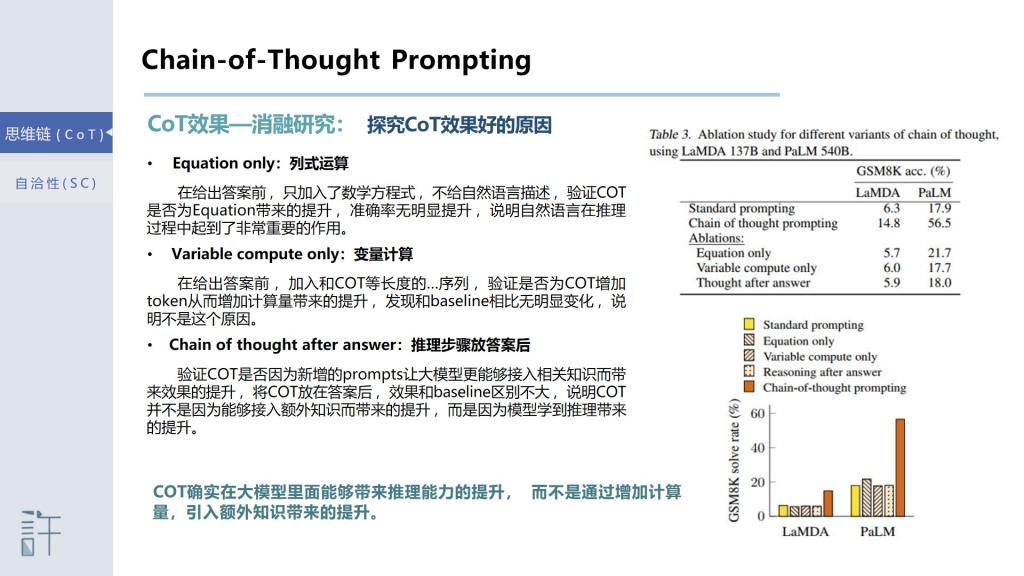
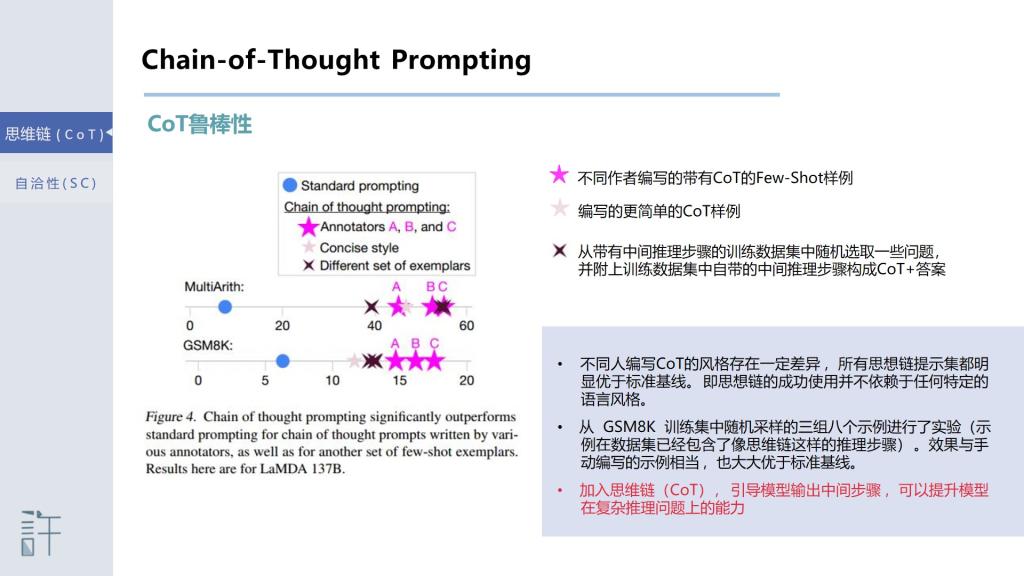
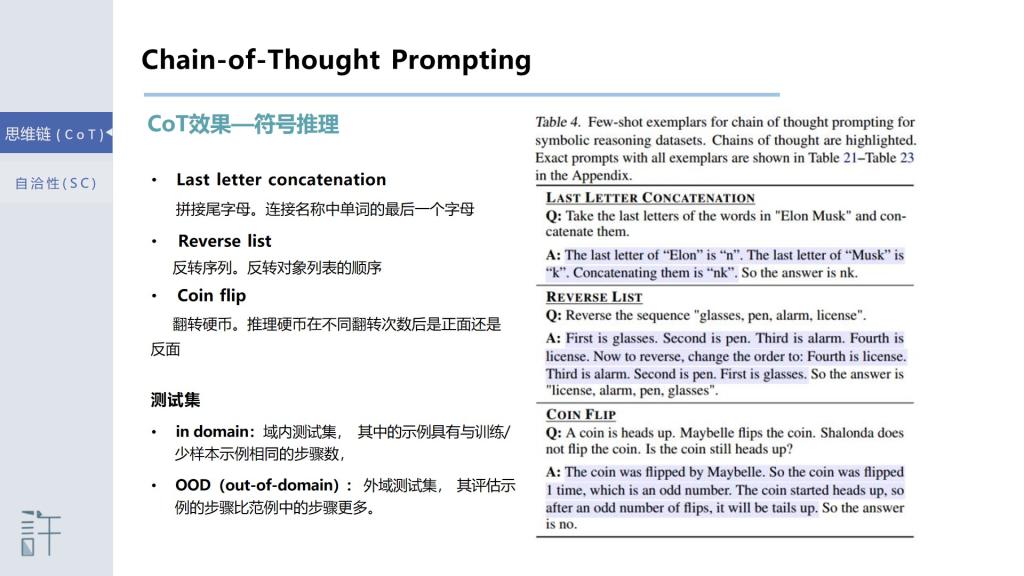
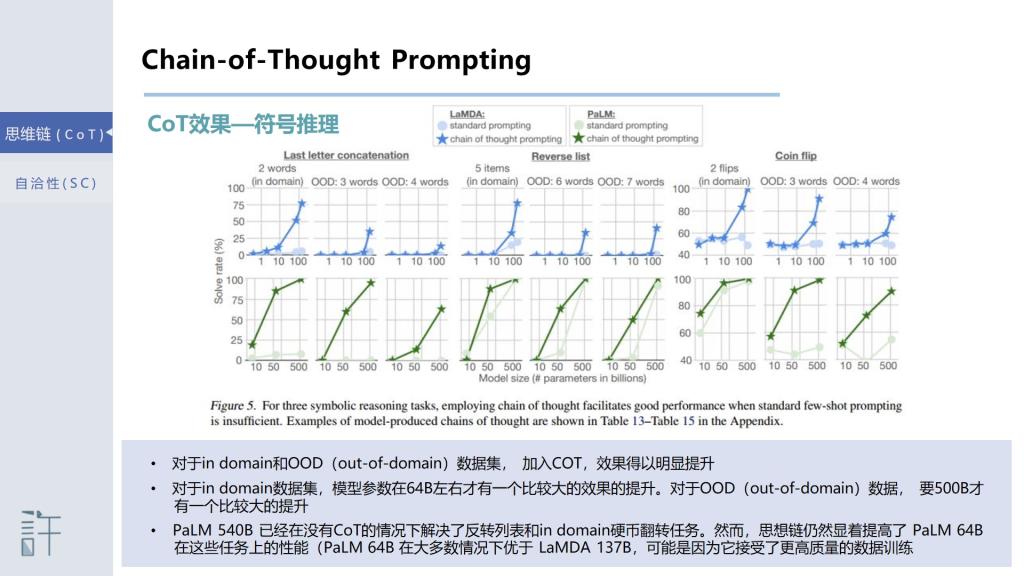
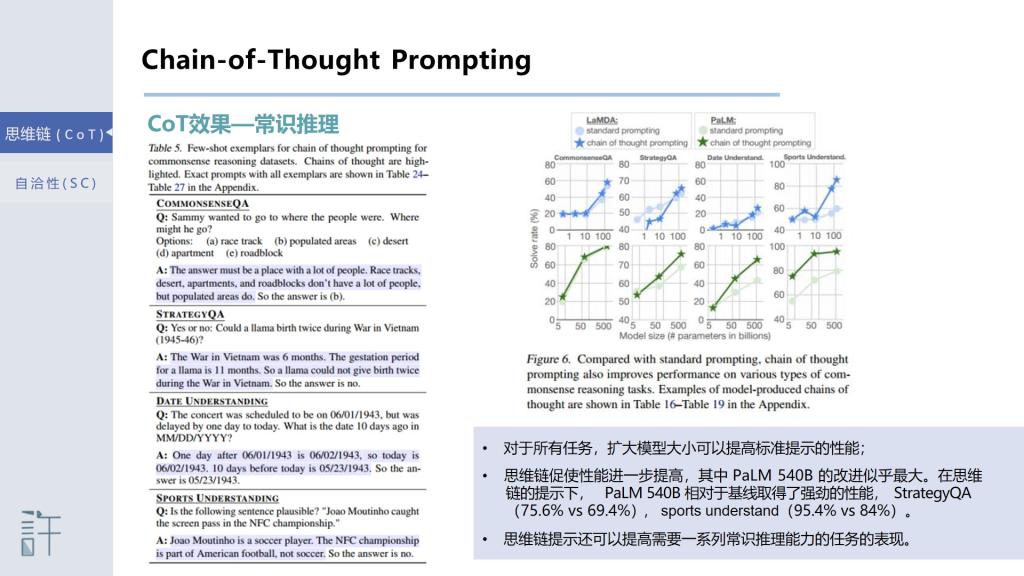
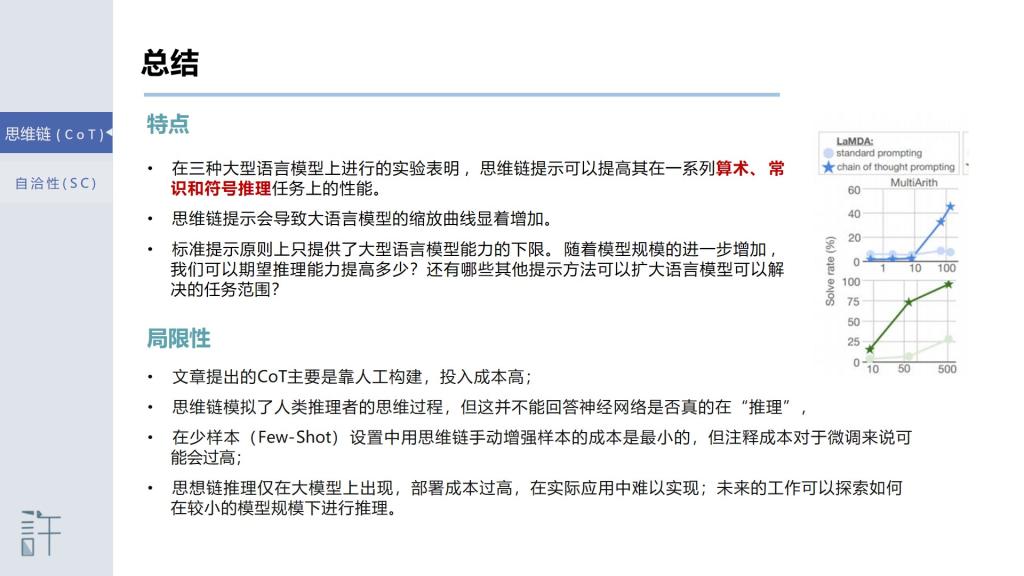
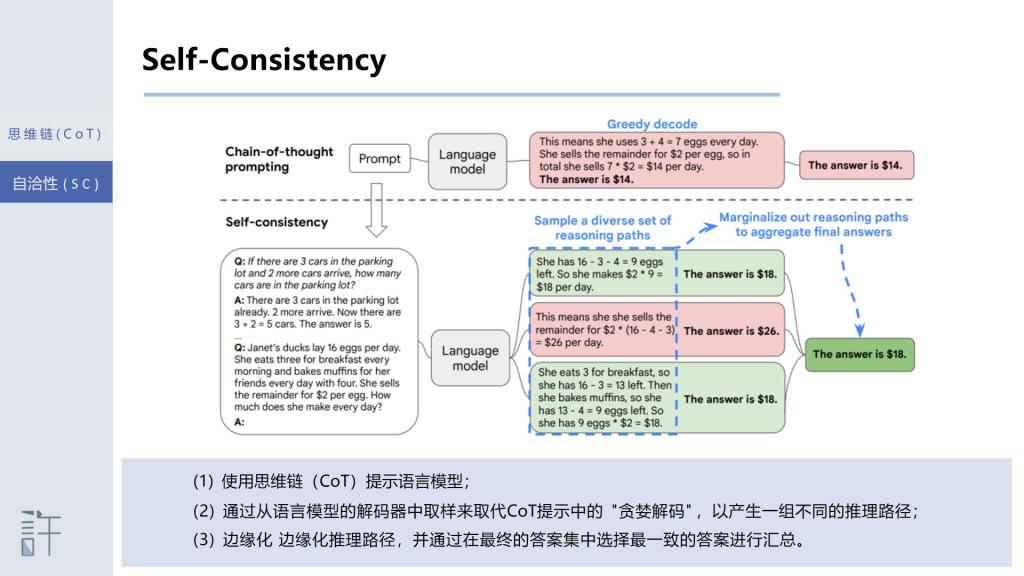
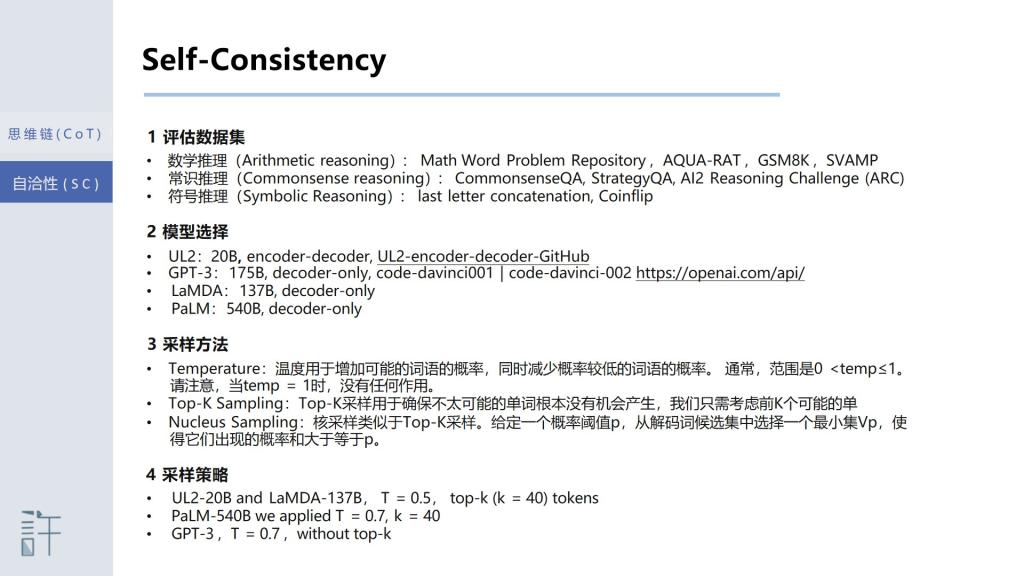
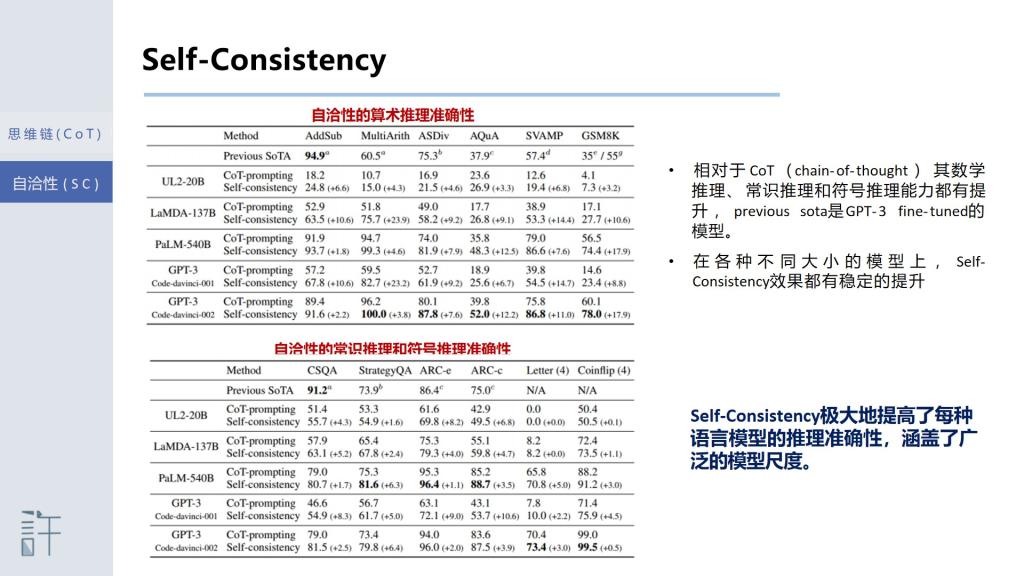
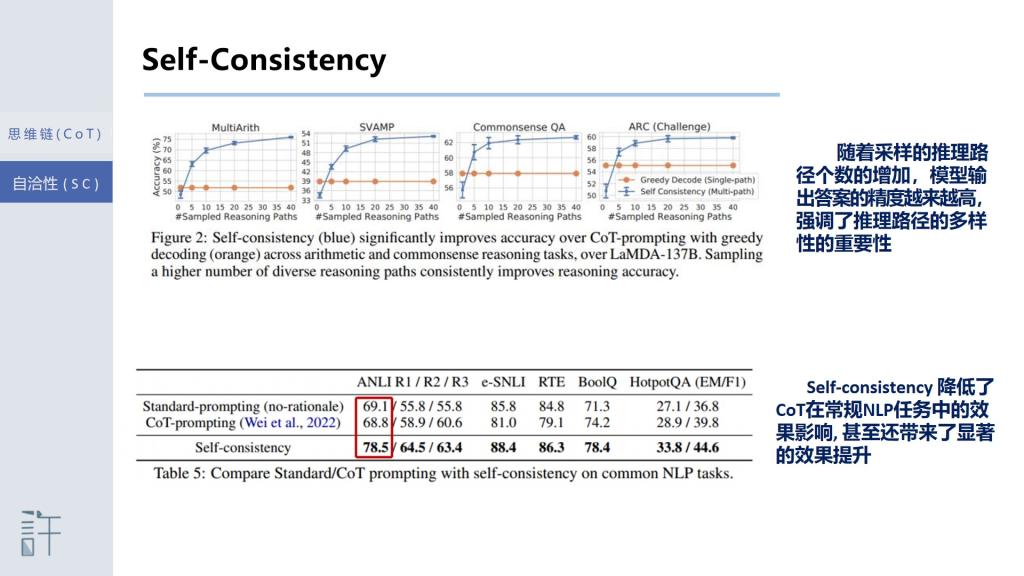
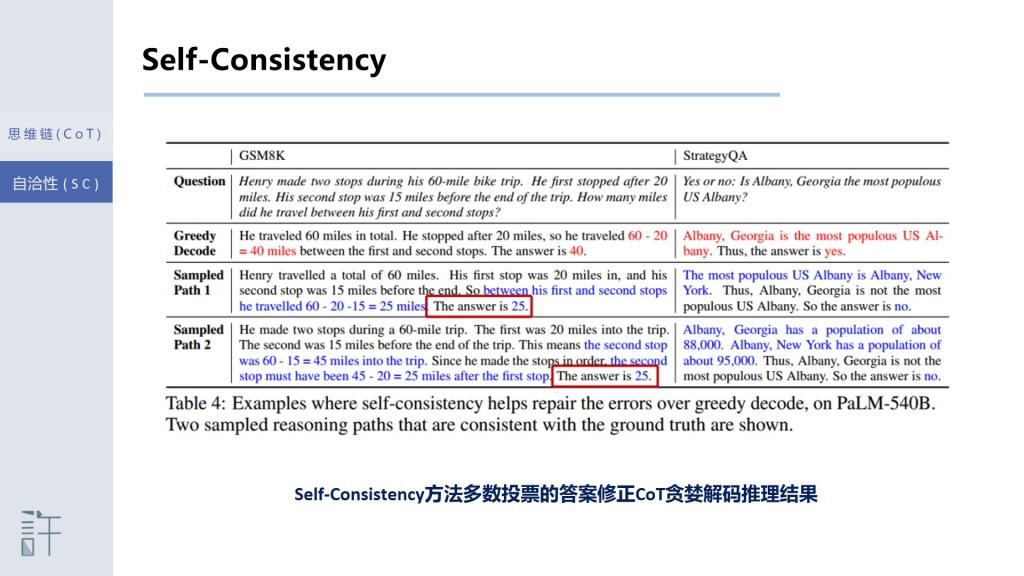
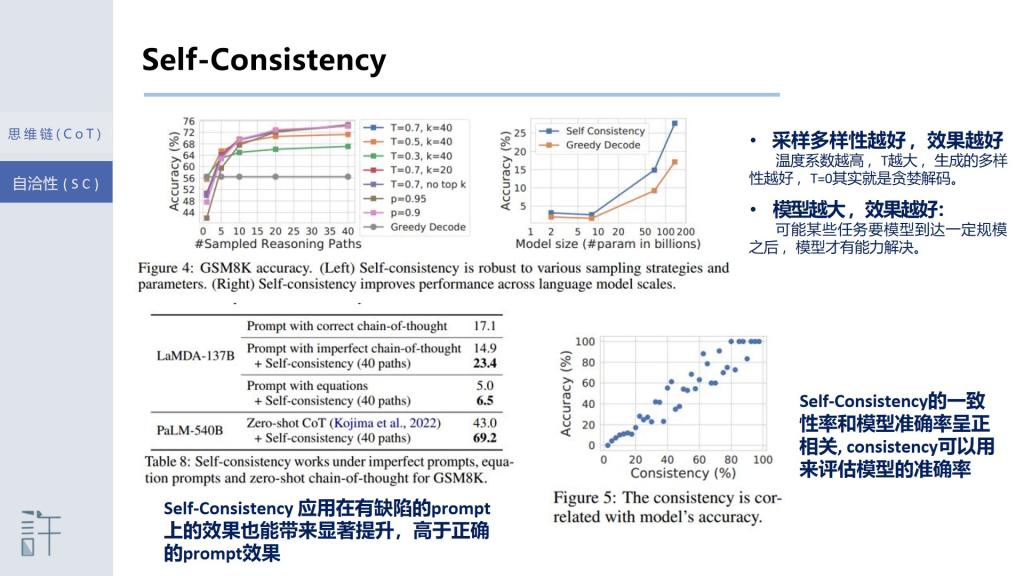
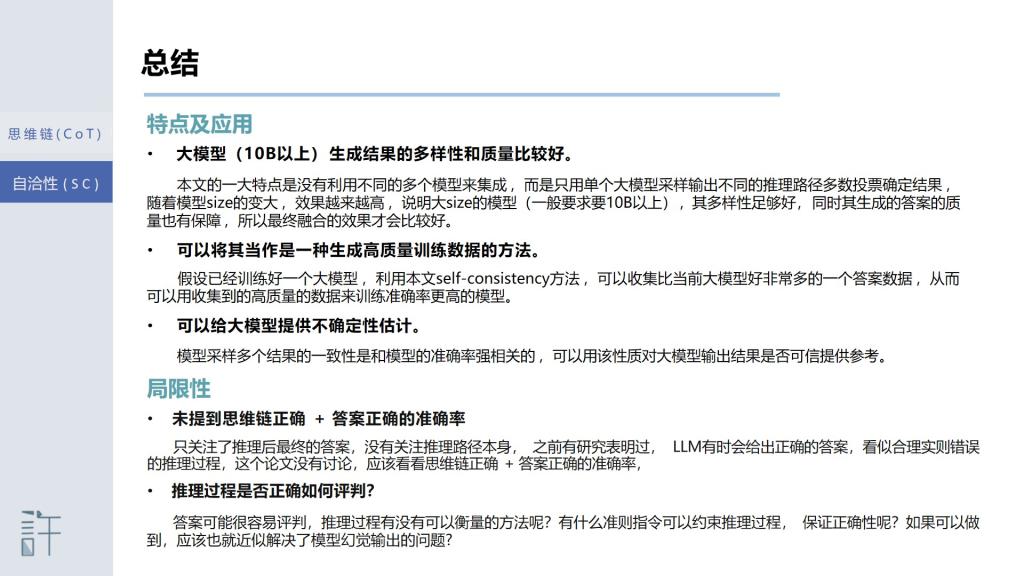
声明:本网站内容版权归许鹏教授课题组所有。未经本课题组授权不得转载、摘编或利用其它方式使用上述作品。