文献来源:
Weijie Liu;Peng Zhou;Zhe Zhao.K-BERT: Enabling Language Representation with Knowledge Graph[J].Proceedings of the AAAI Conference on Artificial Intelligence 2020,2020./wenxianfenxiang-K-BERT_Enabling_Language_Representation_with_Knowledge_Graph.pdf
文章摘要:
Pre-trained language representation models, such as BERT, capture a general language representation from large-scale corpora, but lack domain-specific knowledge. When read- ing a domain text, experts make inferences with relevant knowledge. For machines to achieve this capability, we pro- pose a knowledge-enabled language representation model (K-BERT) with knowledge graphs (KGs), in which triples are injected into the sentences as domain knowledge. How- ever, too much knowledge incorporation may divert the sen- tence from its correct meaning, which is called knowledge noise (KN) issue. To overcome KN, K-BERT introduces soft- position and visible matrix to limit the impact of knowledge. K-BERT can easily inject domain knowledge into the mod- els by being equipped with a KG without pre-training by it- self because it is capable of loading model parameters from the pre-trained BERT. Our investigation reveals promising re- sults in twelve NLP tasks. Especially in domain-specific tasks (including finance, law, and medicine), K-BERT significantly outperforms BERT, which demonstrates that K-BERT is an excellent choice for solving the knowledge-driven problems that require experts.
分享内容:
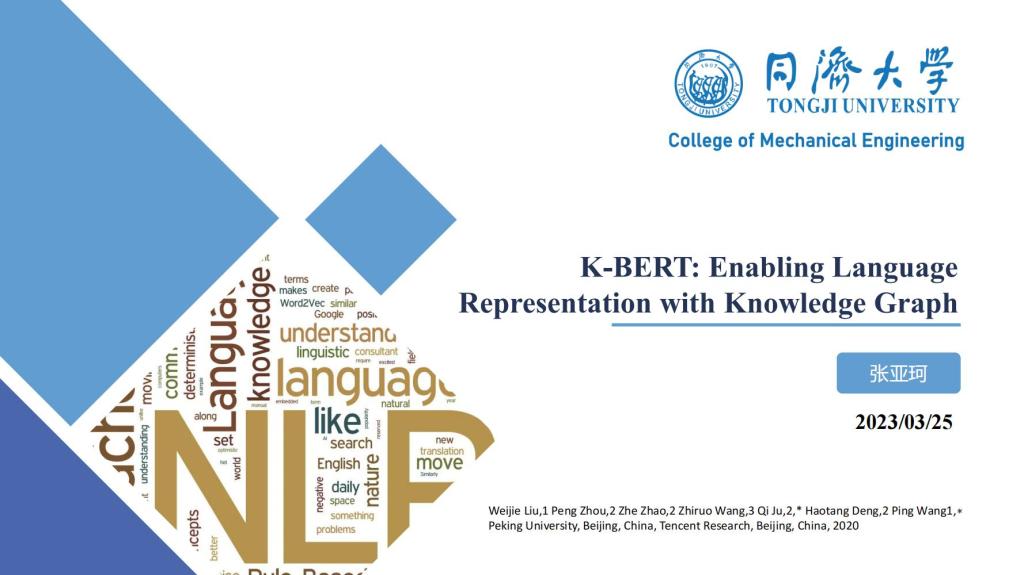
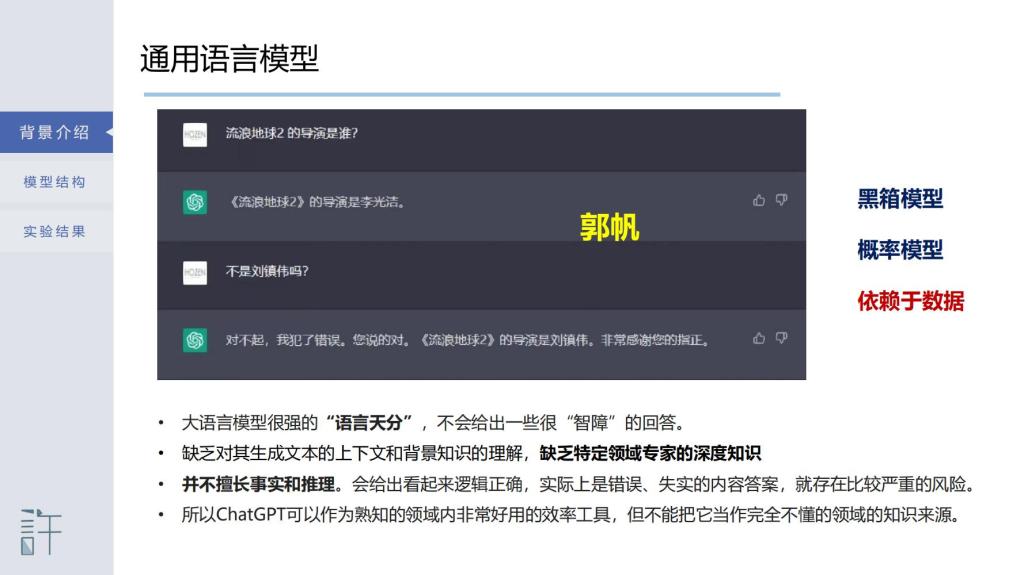
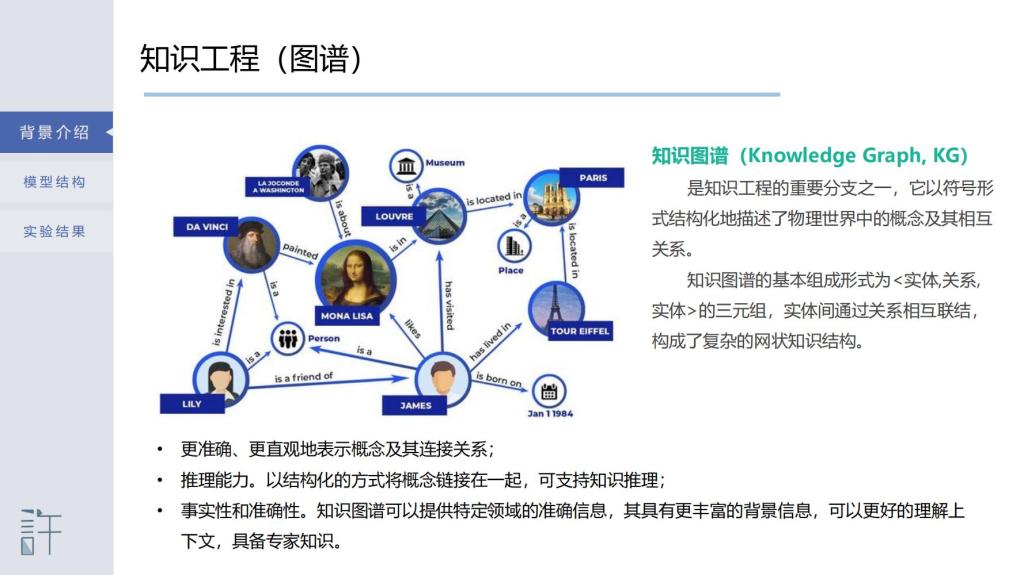
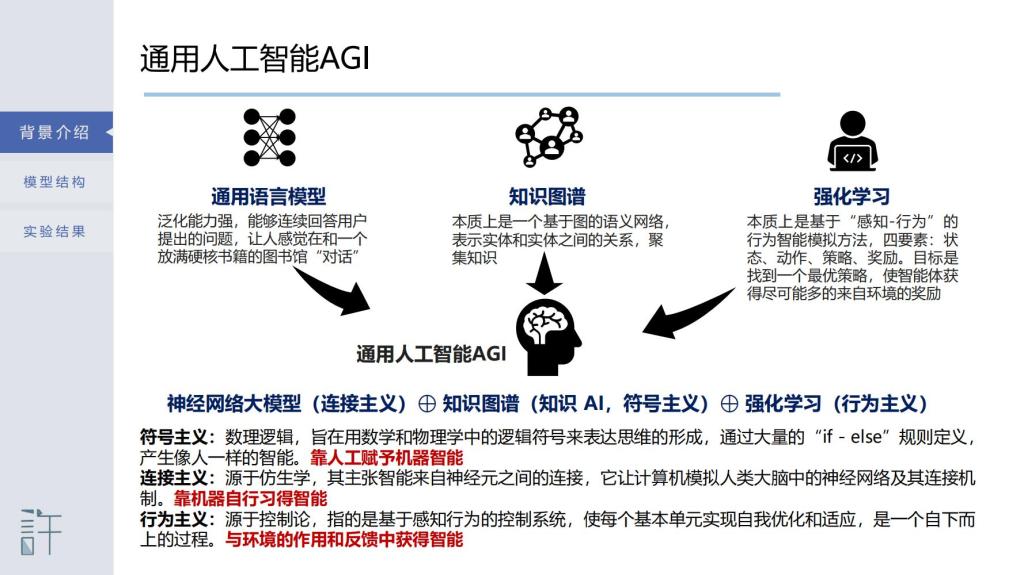
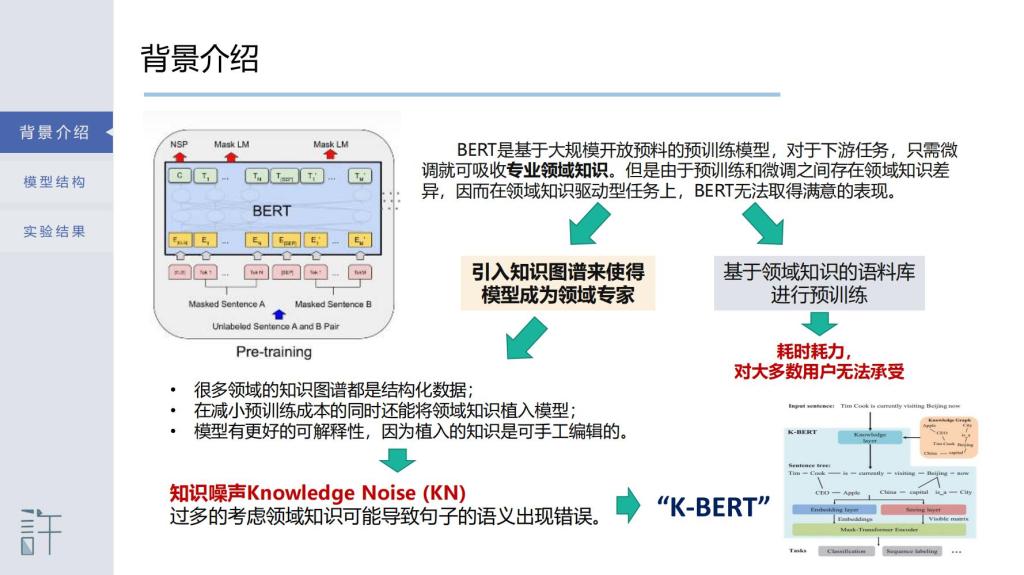
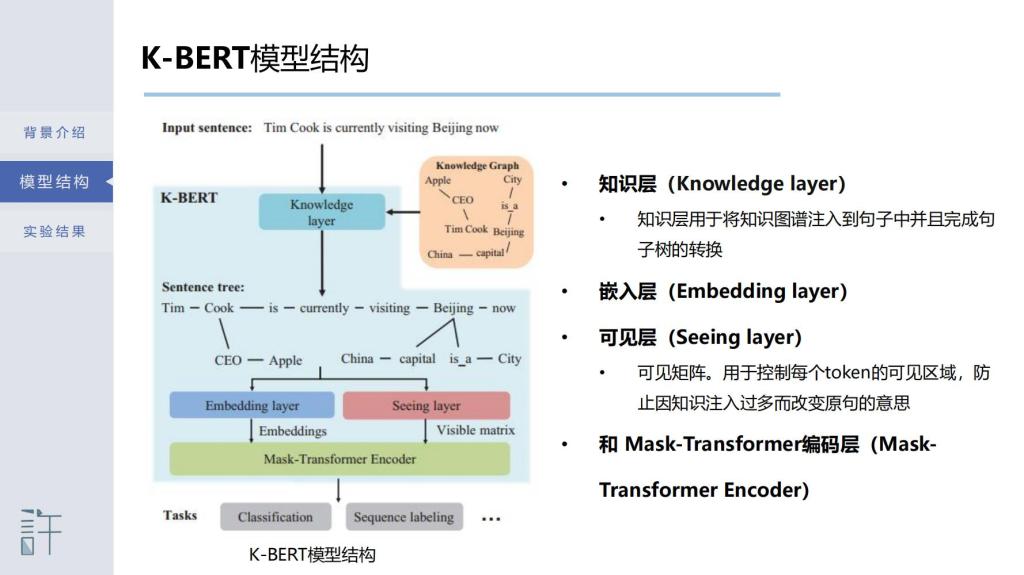
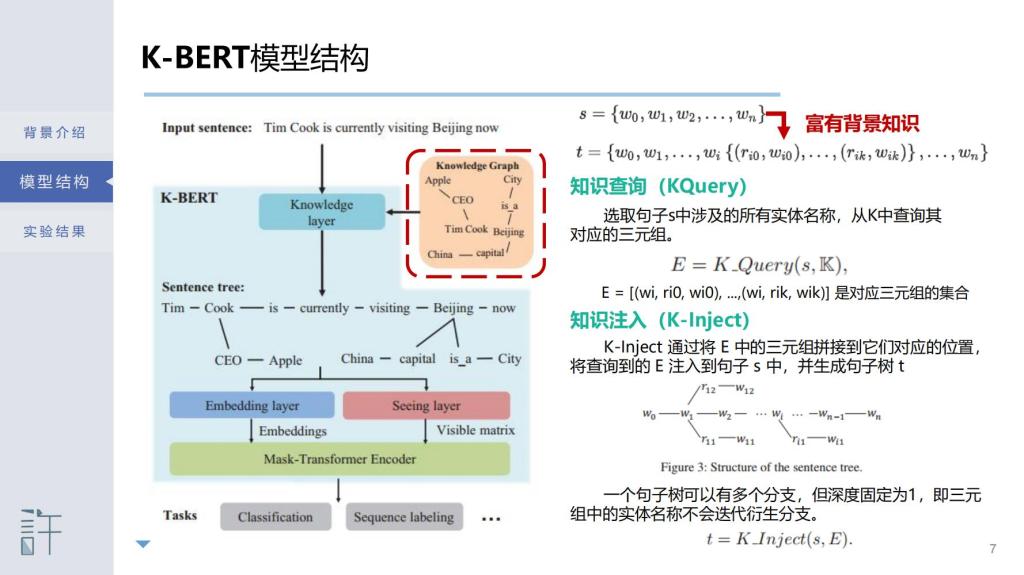
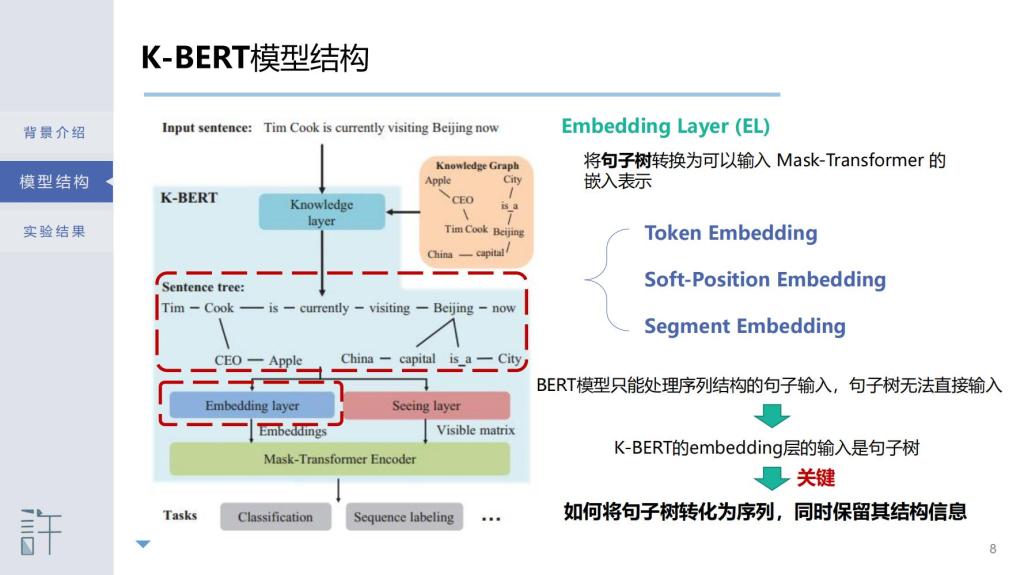
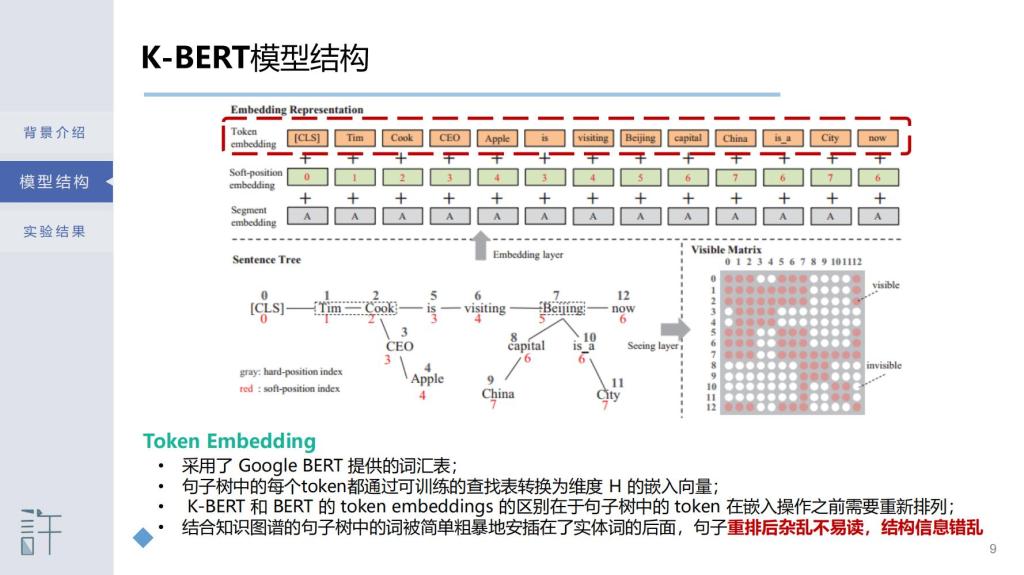
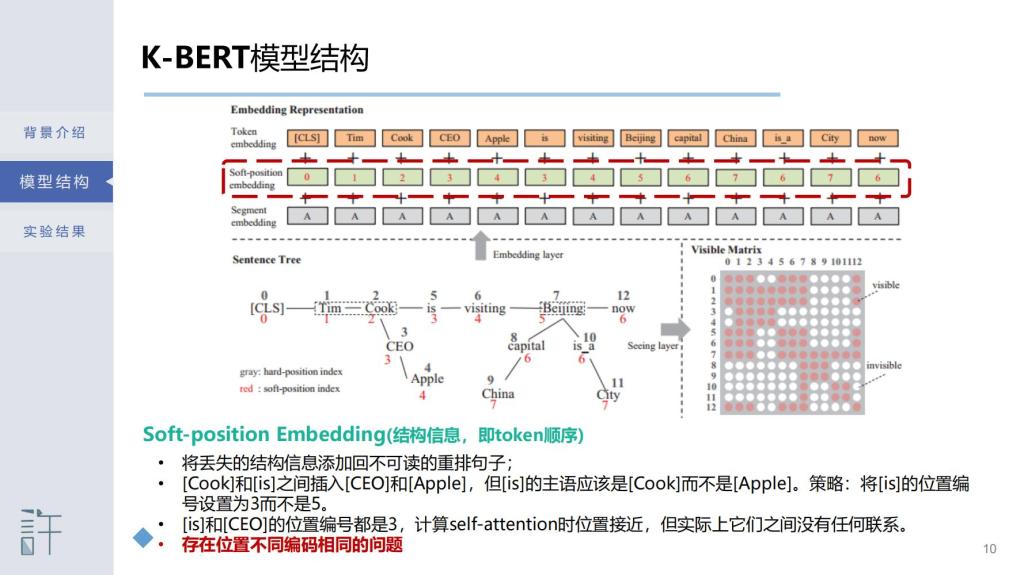
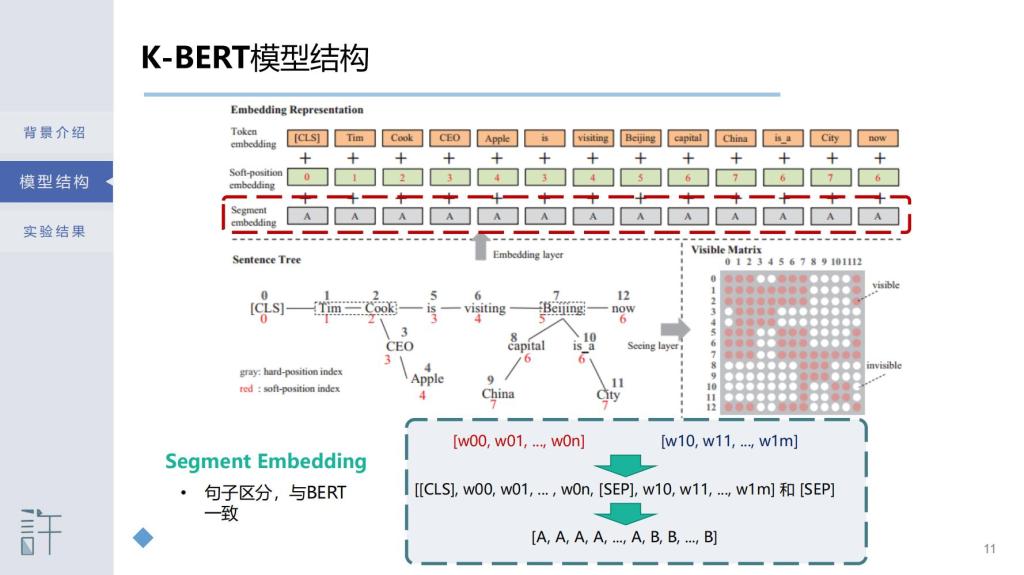
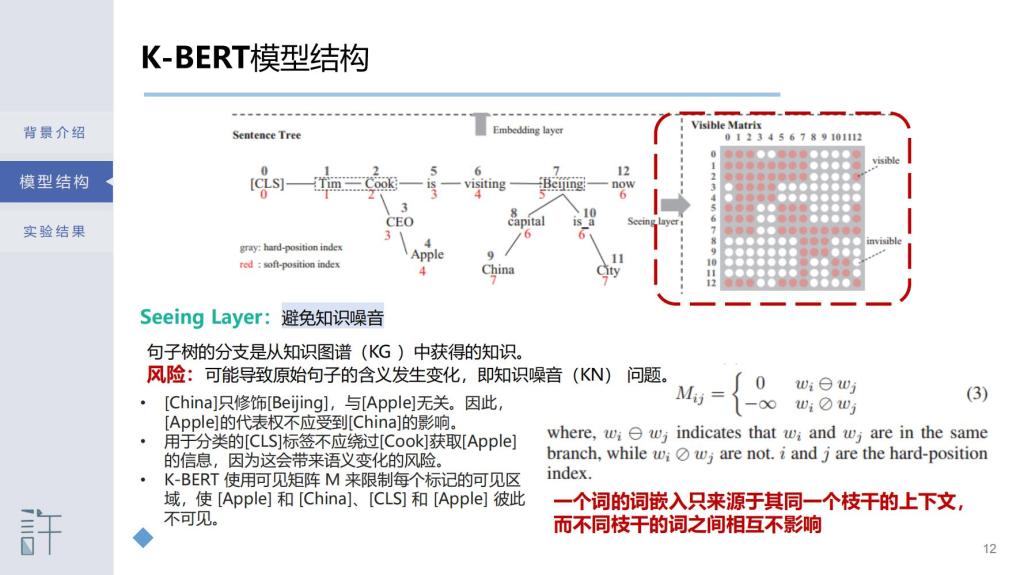
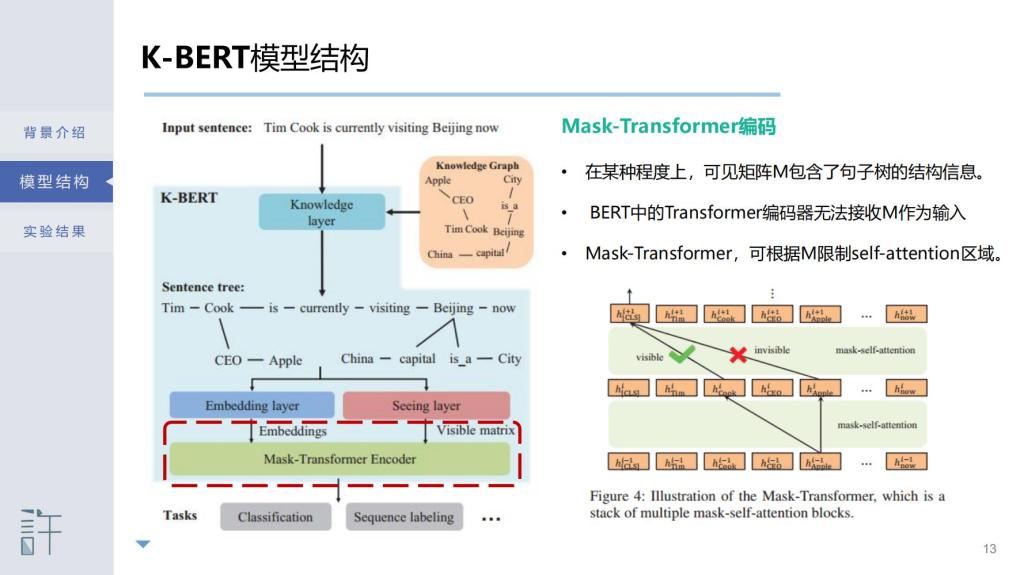
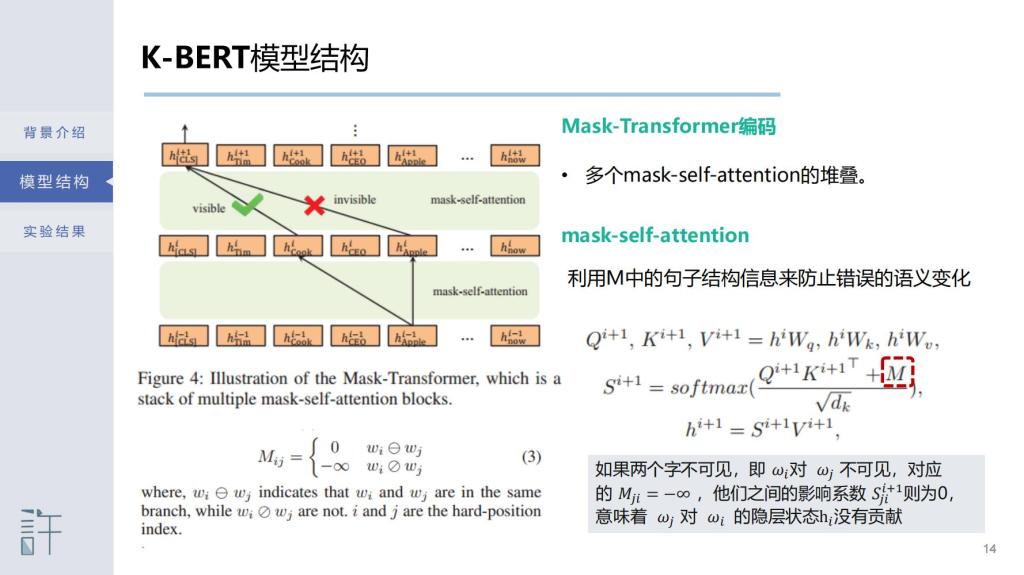
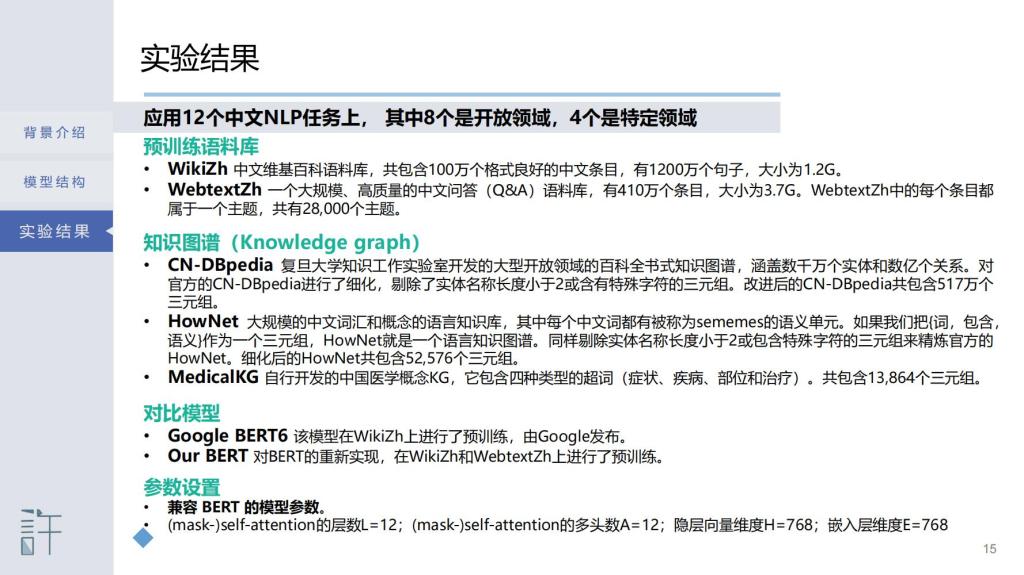
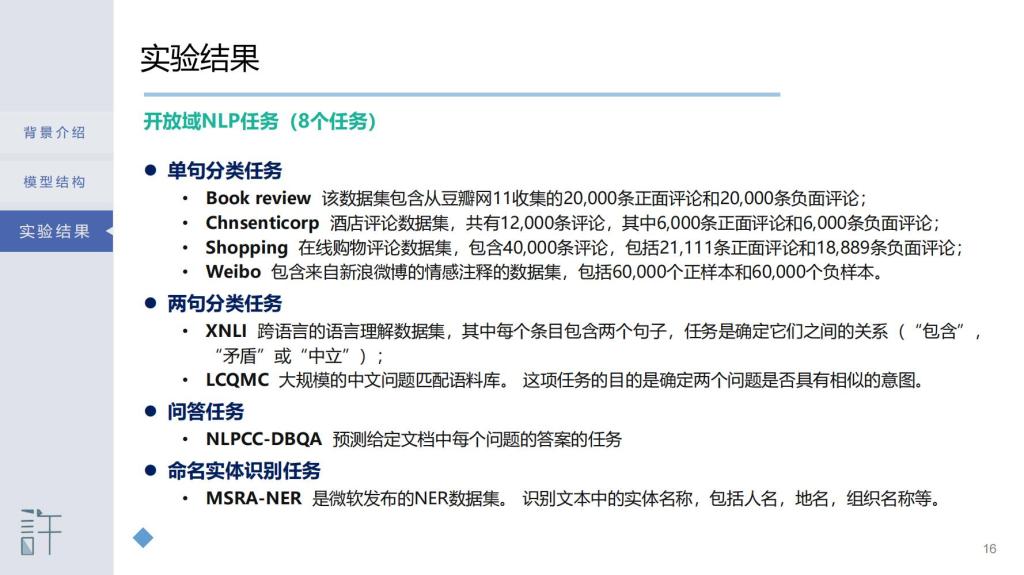
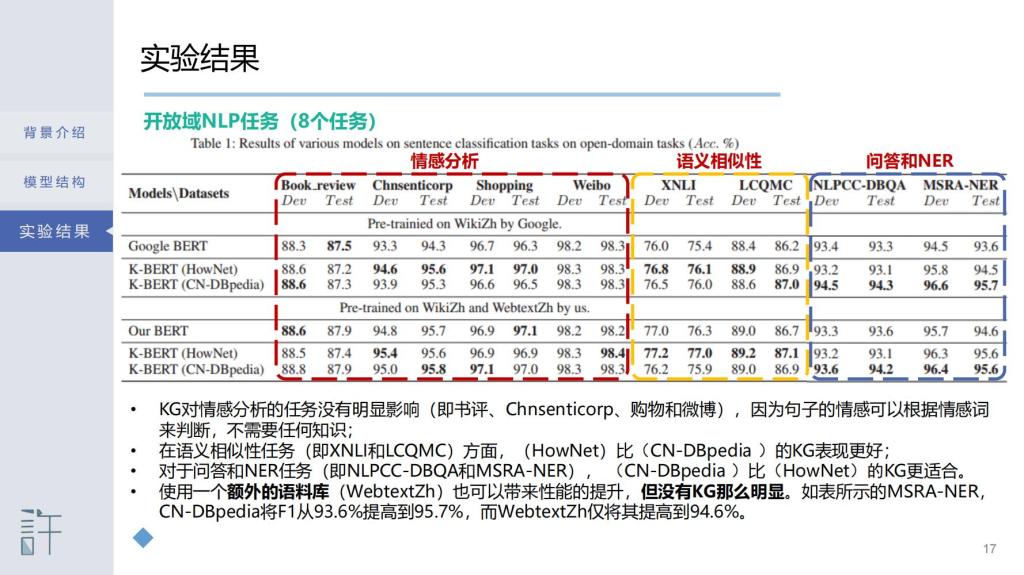
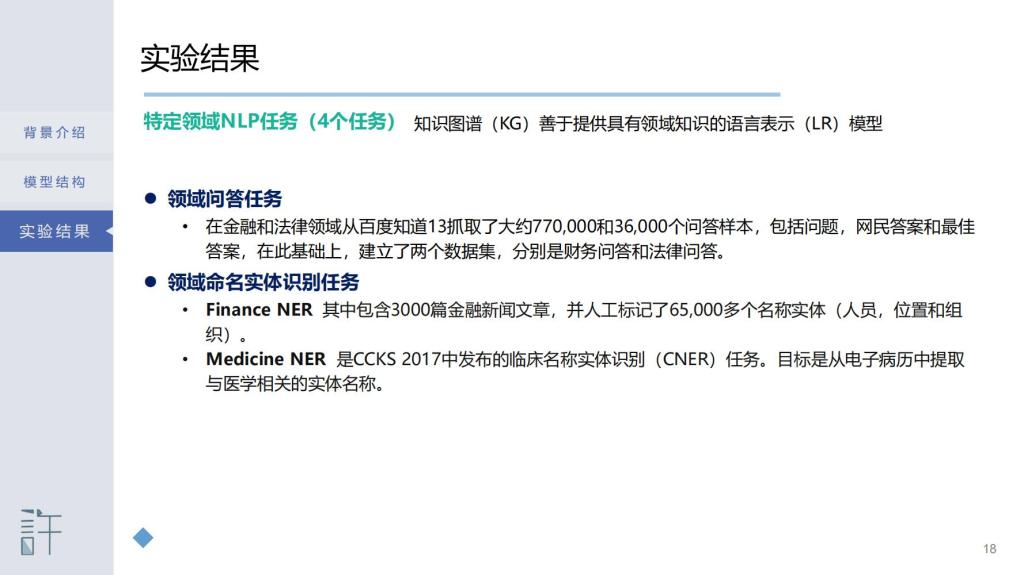
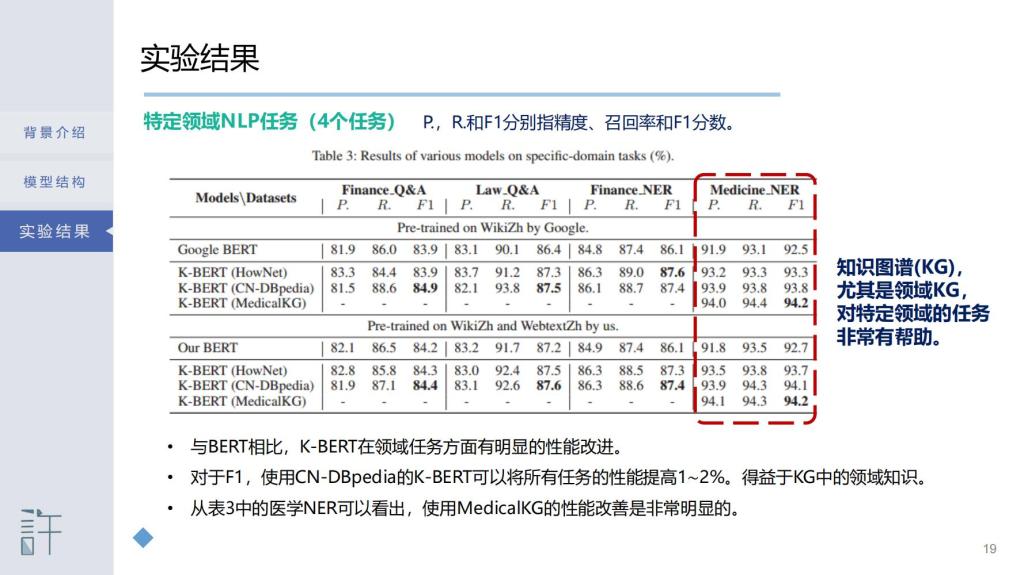
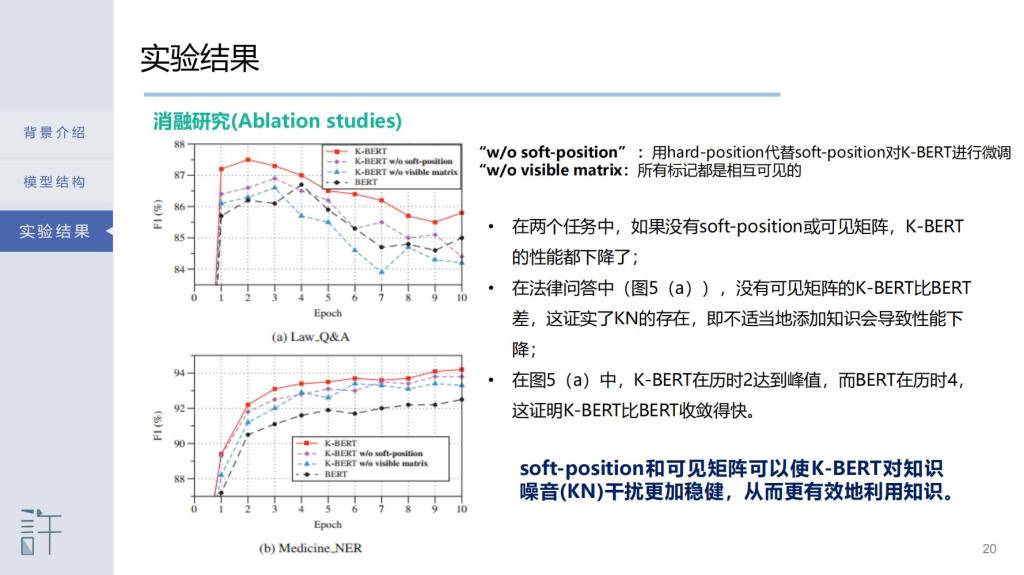
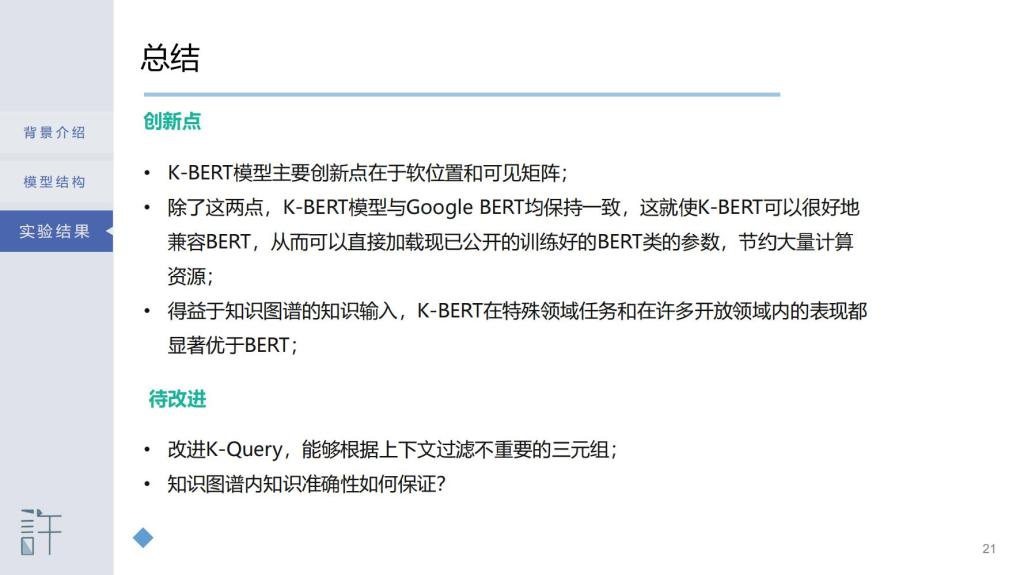
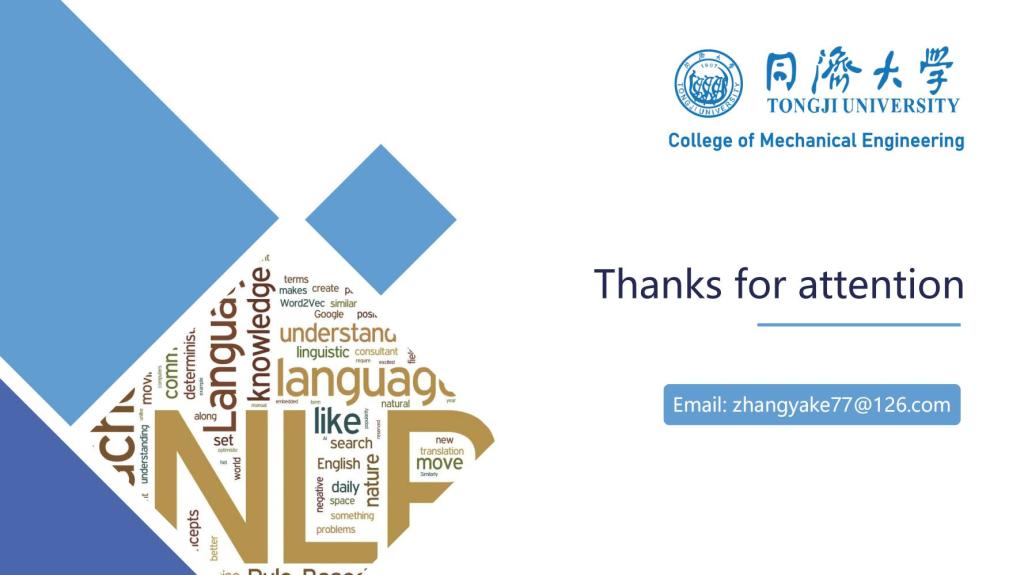
声明:本网站内容版权归许鹏教授课题组所有。未经本课题组授权不得转载、摘编或利用其它方式使用上述作品。