文献来源:
Sun, Ying;Haghighat, Fariborz;Fung, Benjamin C.M.A review of the-state-of-the-art in data-driven approaches for building energy prediction.[J].Energy & Buildings,2020,Vol.221: 110022
文章摘要:
Building energy prediction plays a vital role in developing a model predictive controller for consumers and optimizing energy distribution plan for utilities. Common approaches for energy prediction include physical models, data-driven models and hybrid models. Among them, data-driven approaches have become a popular topic in recent years due to their ability to discover statistical patterns without expertise knowledge. To acquire the latest research trends, this study first summarizes the limitations of earlier reviews: seldom present comprehensive review for the entire data-driven process for building energy prediction and rarely summarize the input updating strategies when applying the trained data-driven model to multi-step energy prediction. To overcome these gaps, this paper provides a comprehensive review on building energy prediction, covering the entire data-driven process that includes feature engineering, potential data-driven models and expected outputs. The distribution of 105 papers, which focus on building energy prediction by data-driven approaches, are reviewed over data source, feature types, model utilization and prediction outputs. Then, in order to implement the trained data-driven models into multi-step prediction, input updating strategies are reviewed to deal with the time series property of energy related data. Finally, the review concludes with some potential future research directions based on discussion of existing research gaps.
分享内容:
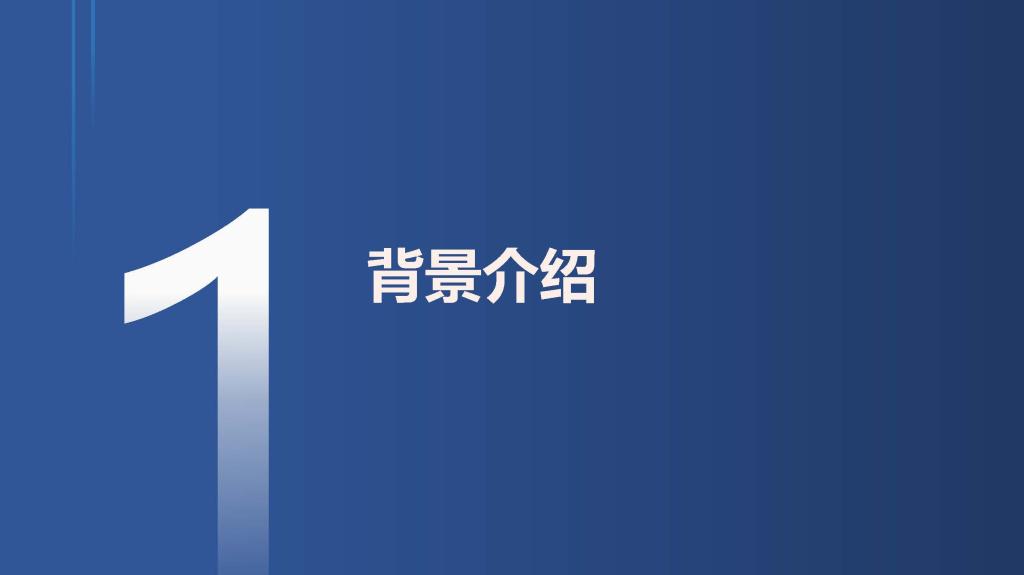
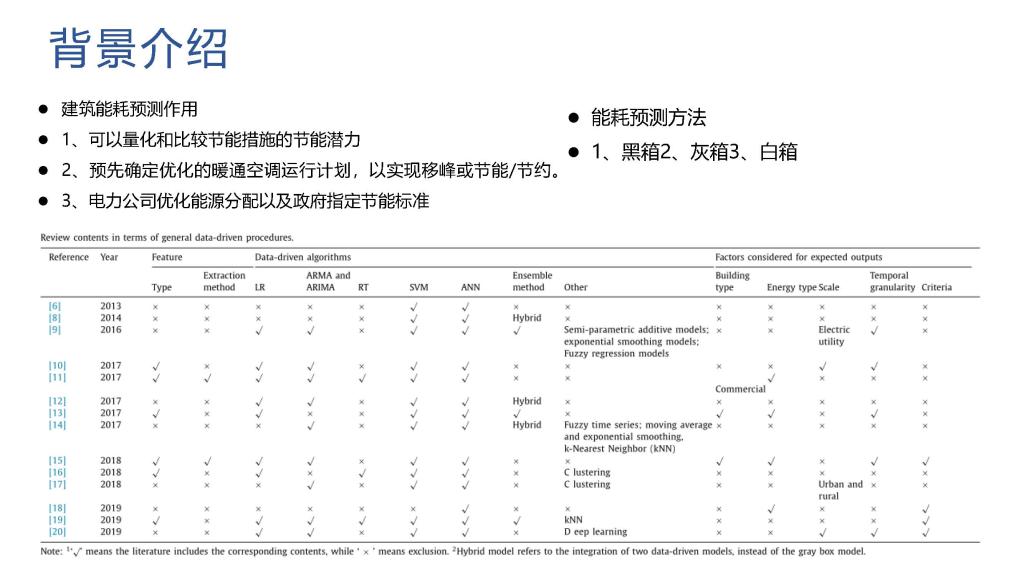
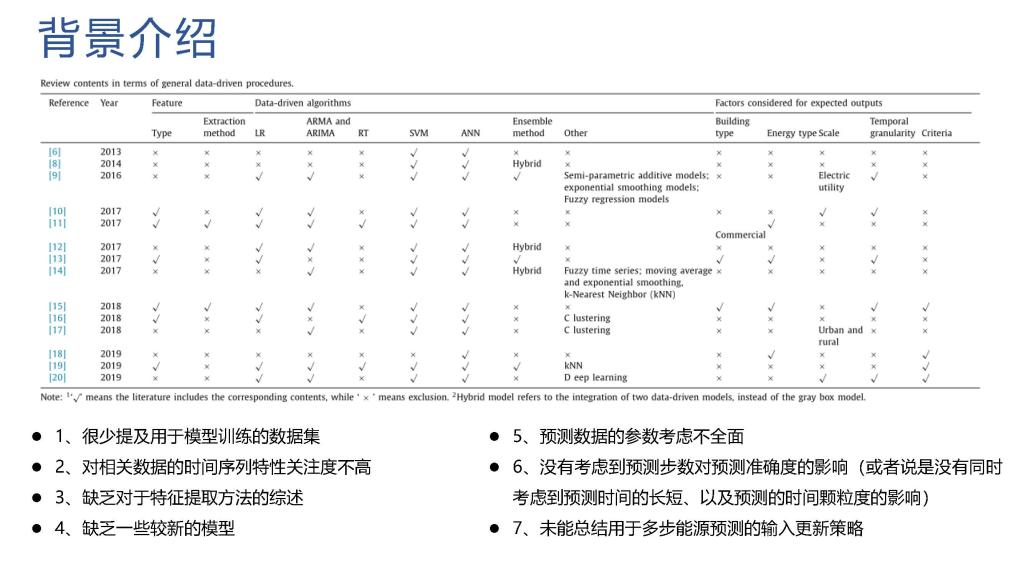
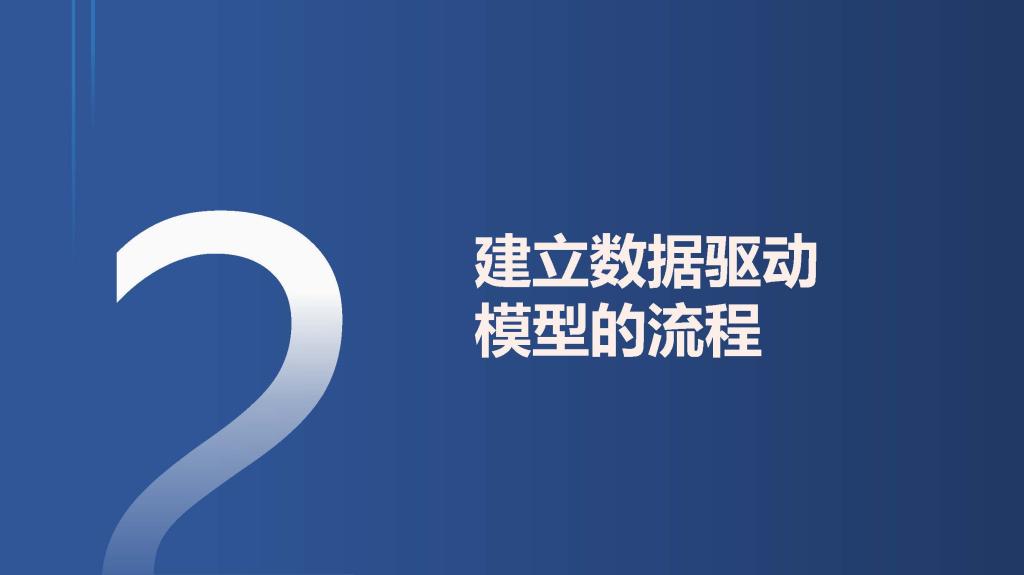
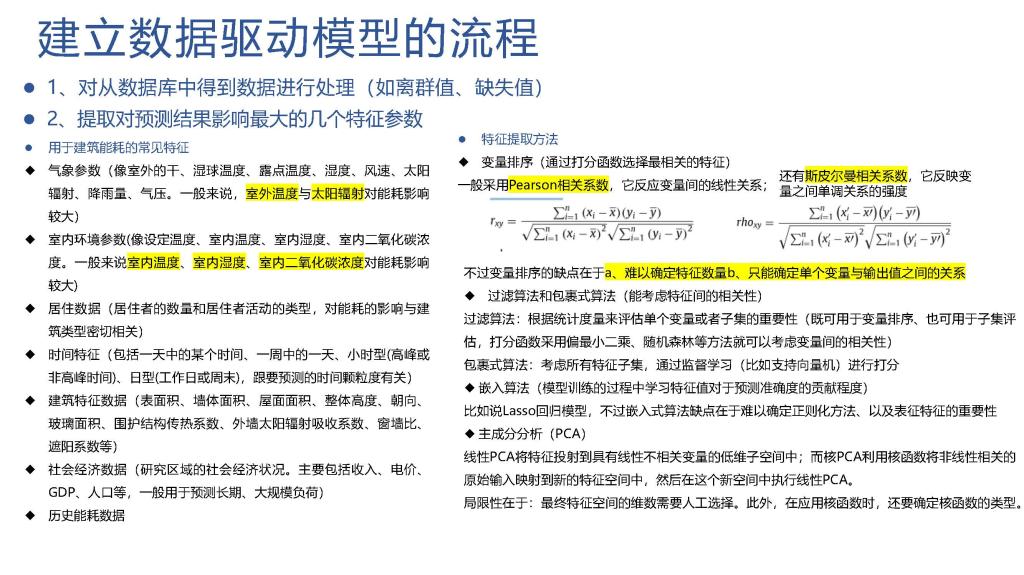
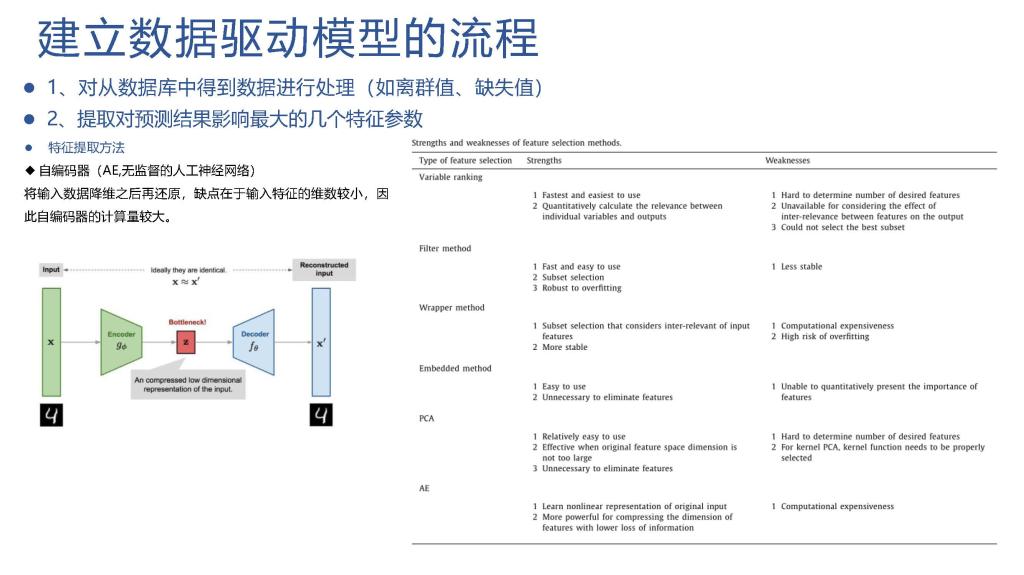
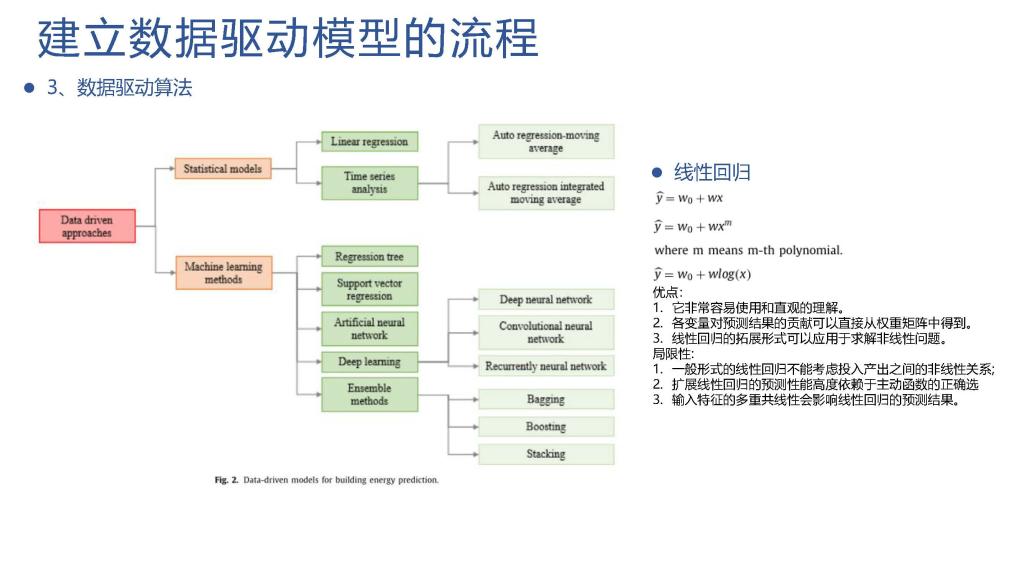
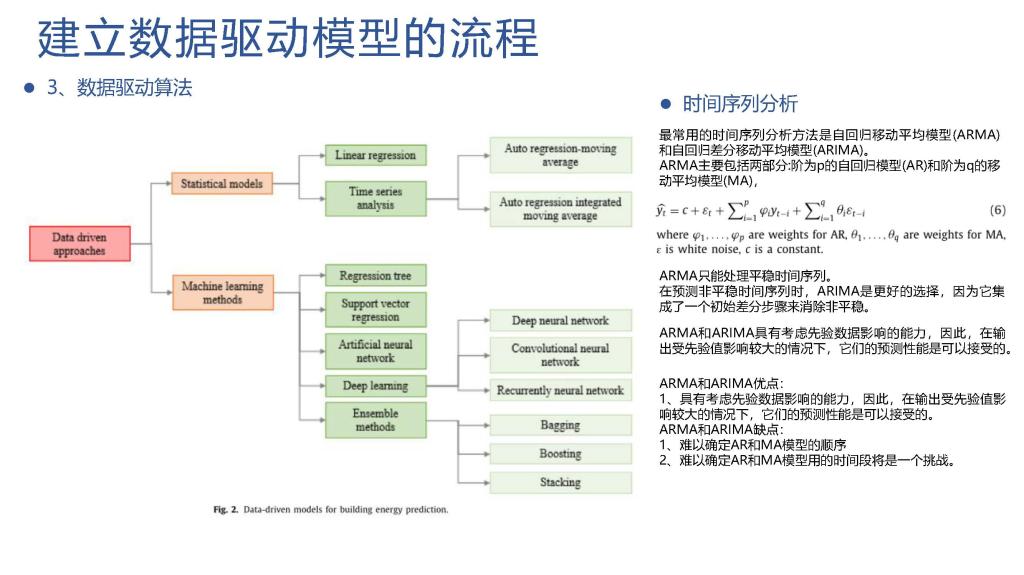
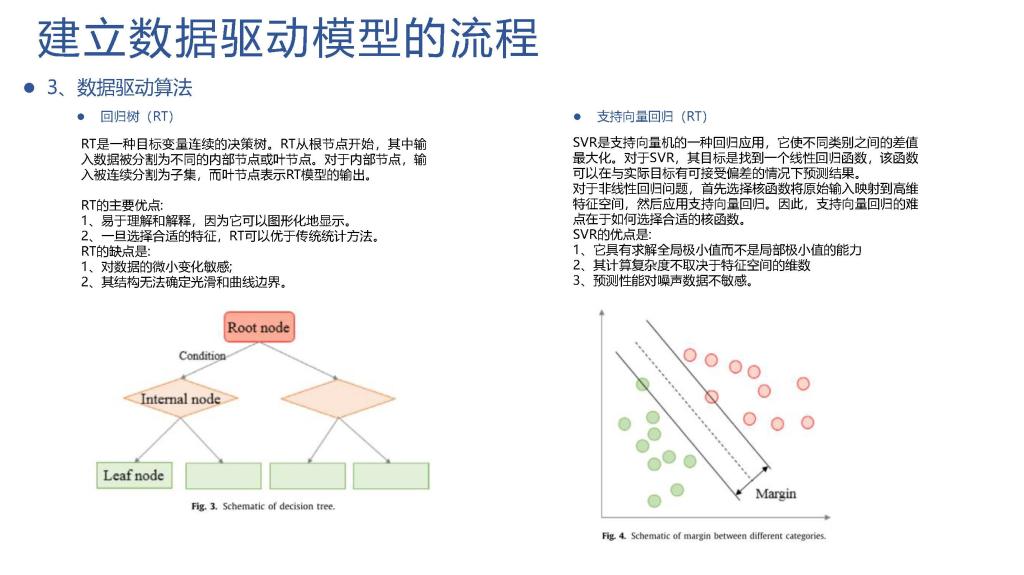
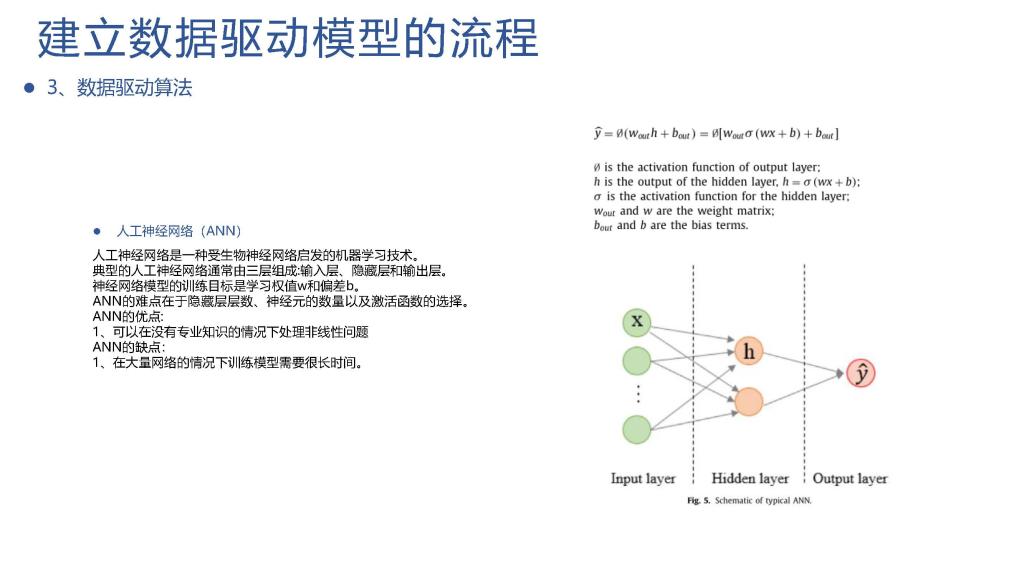
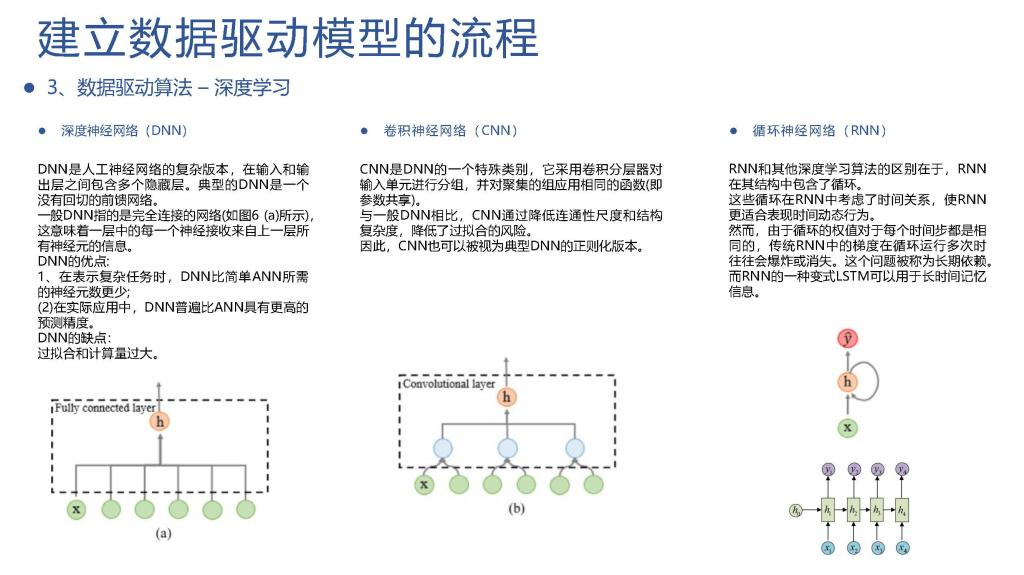
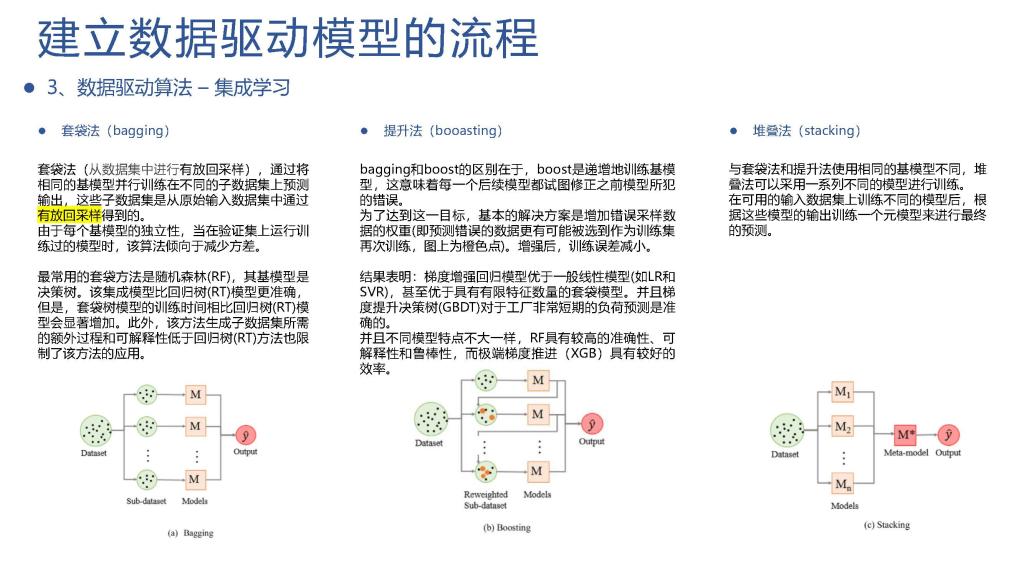
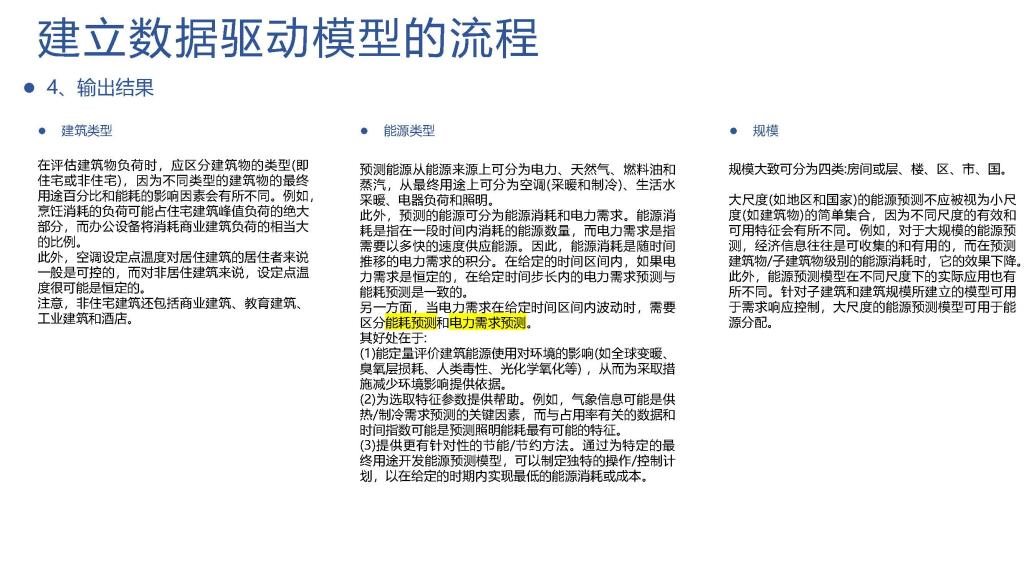
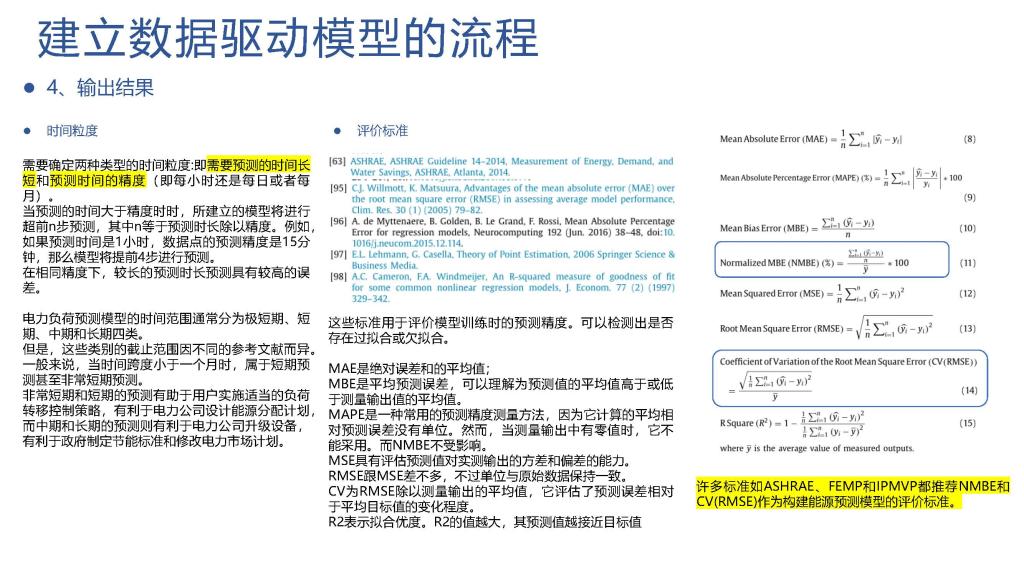
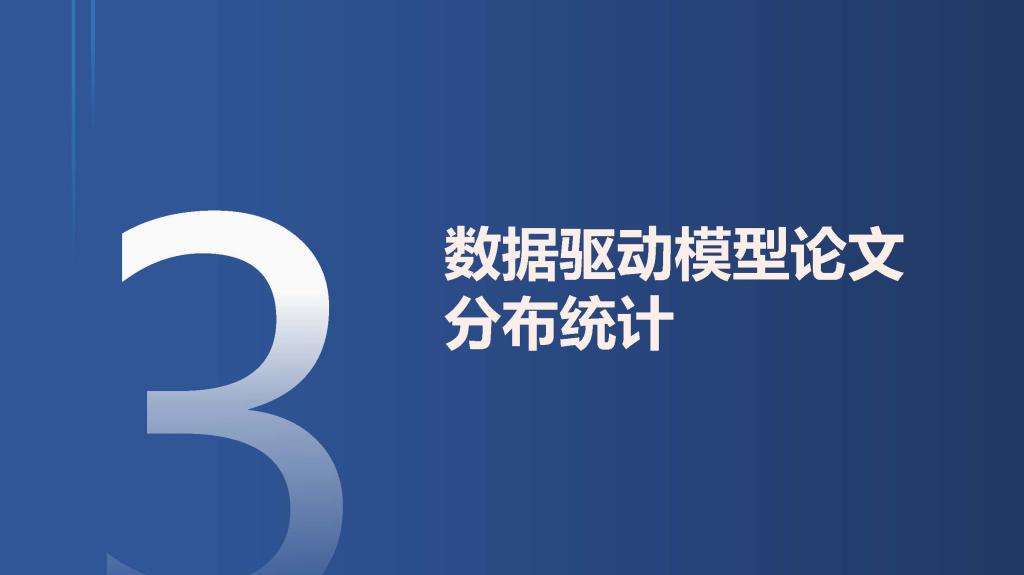
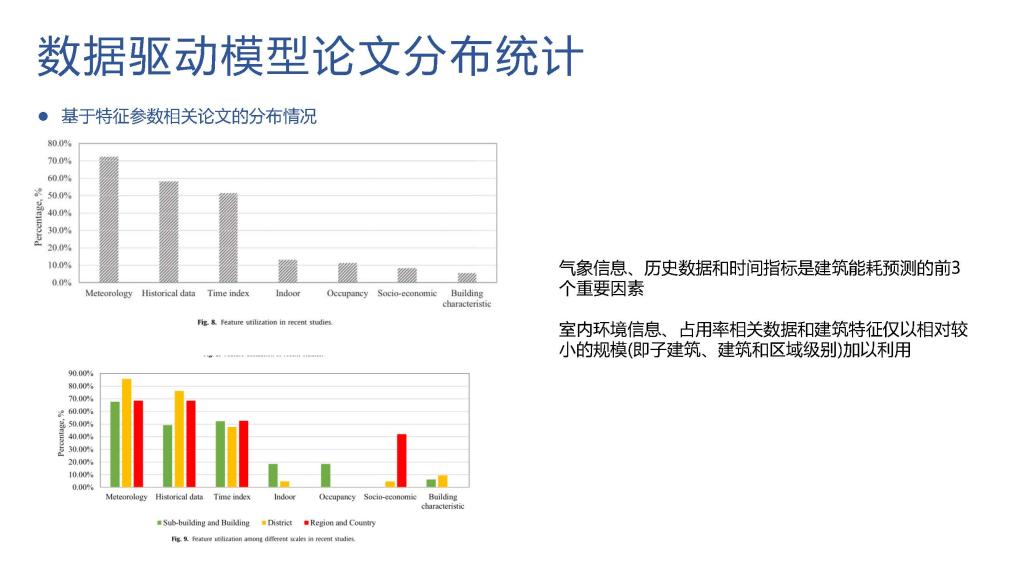
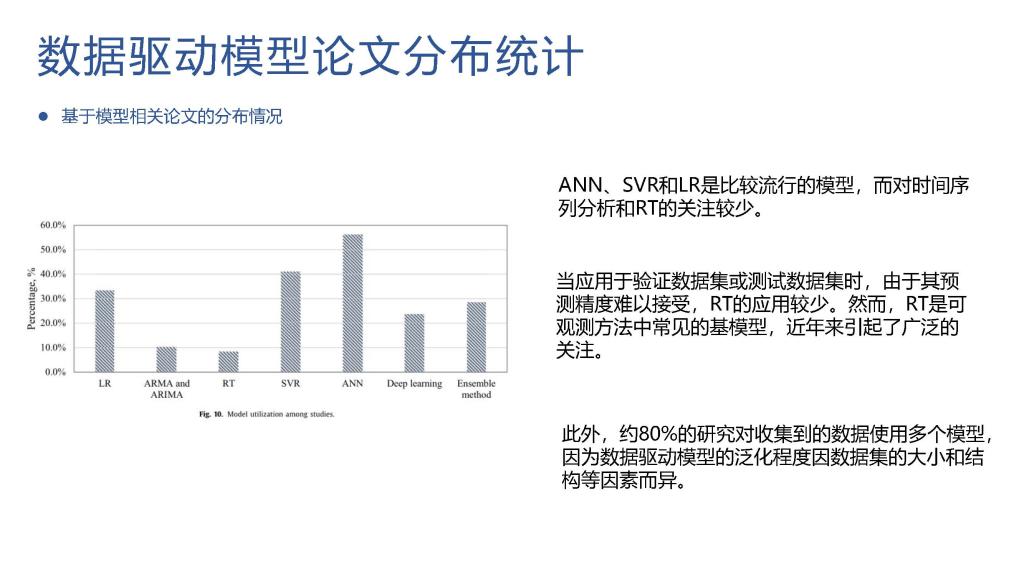
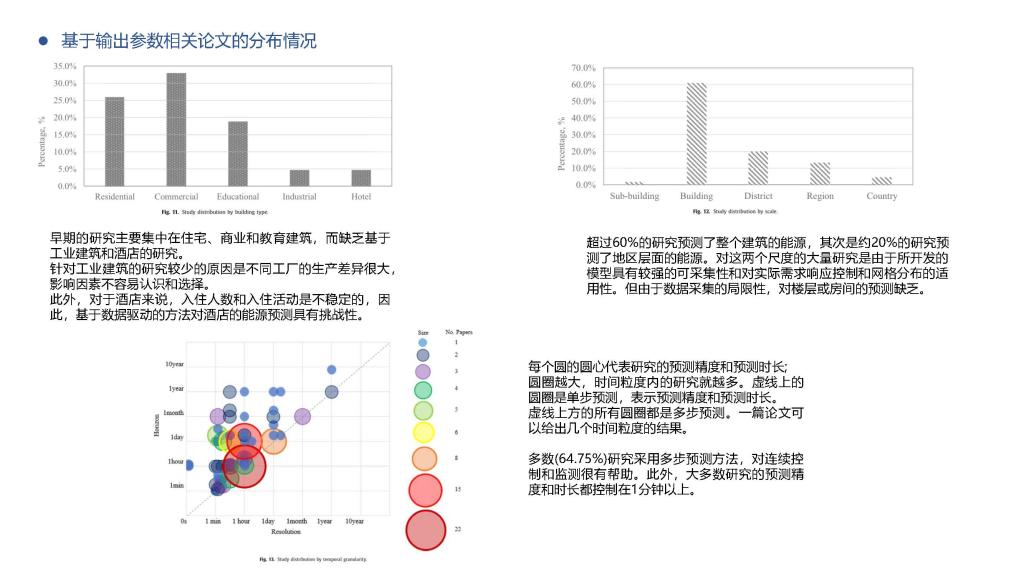
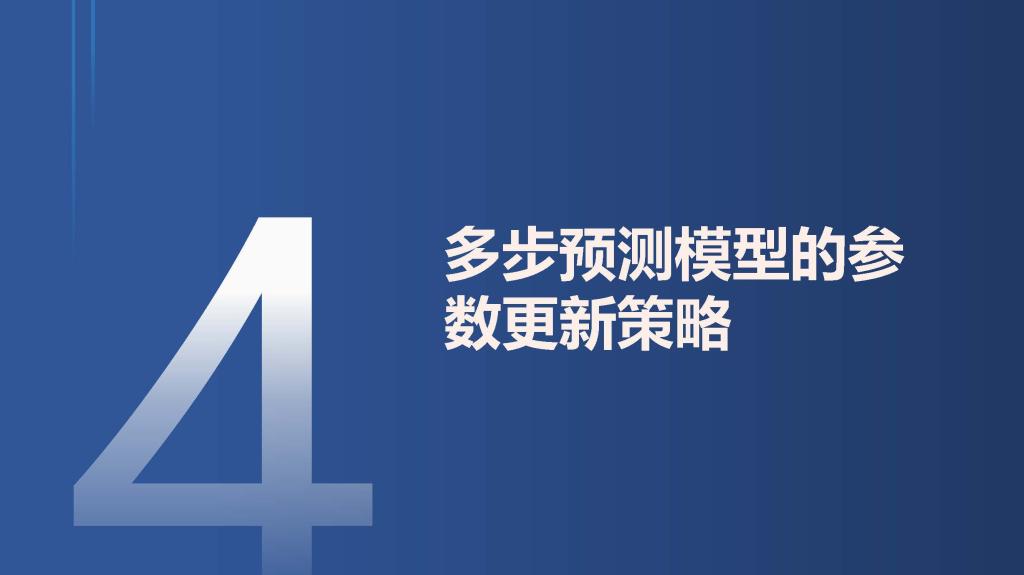
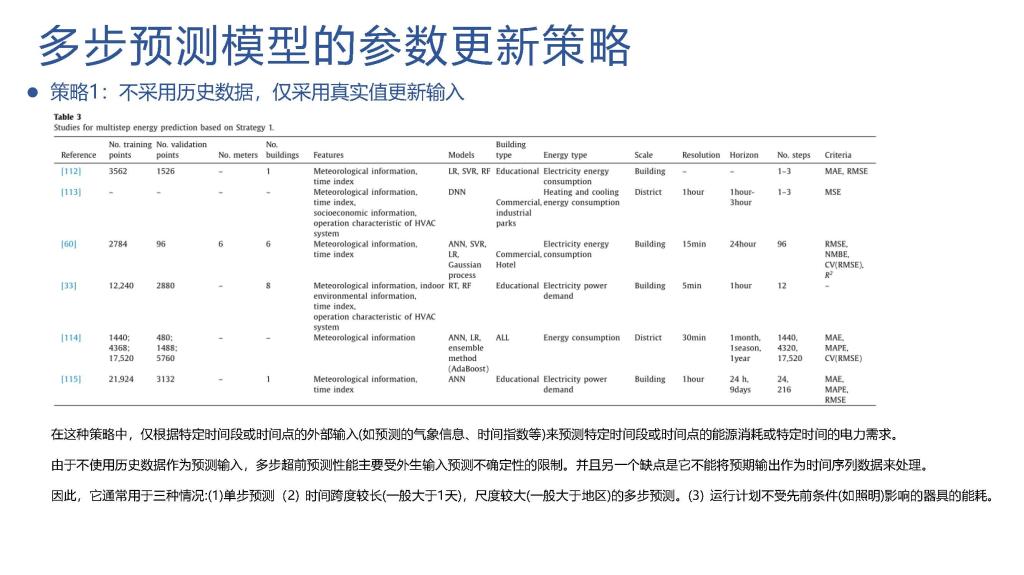
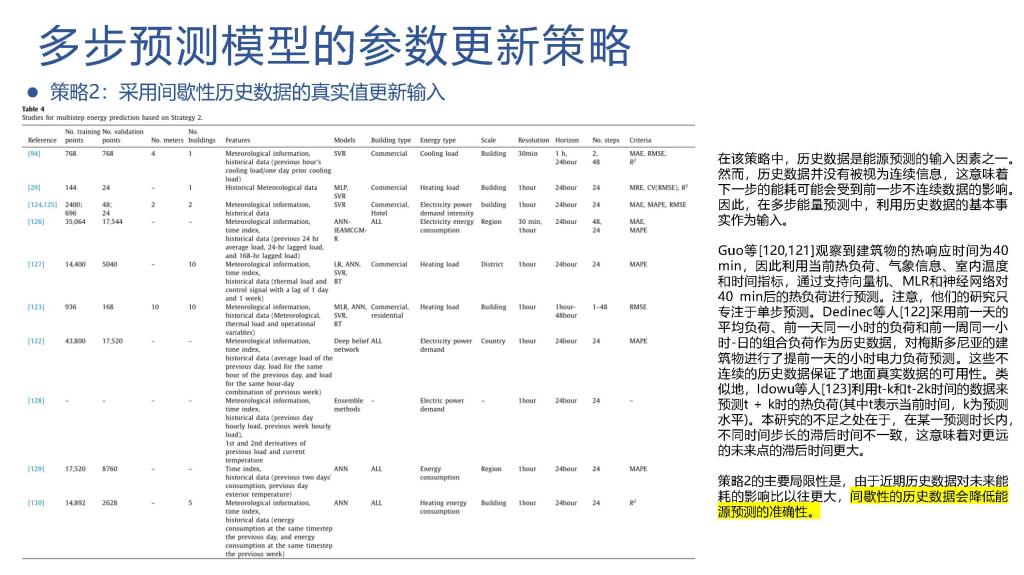
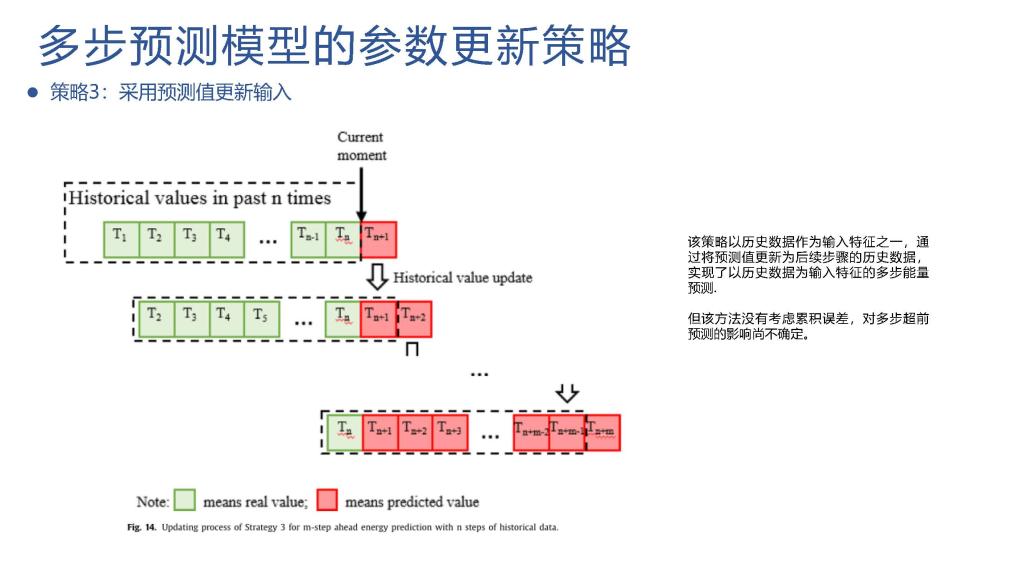
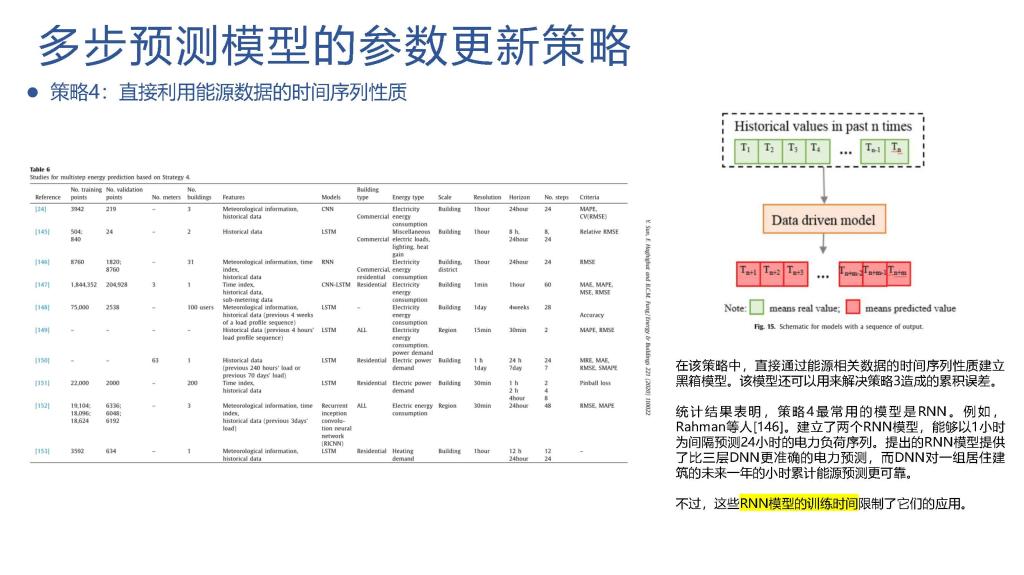
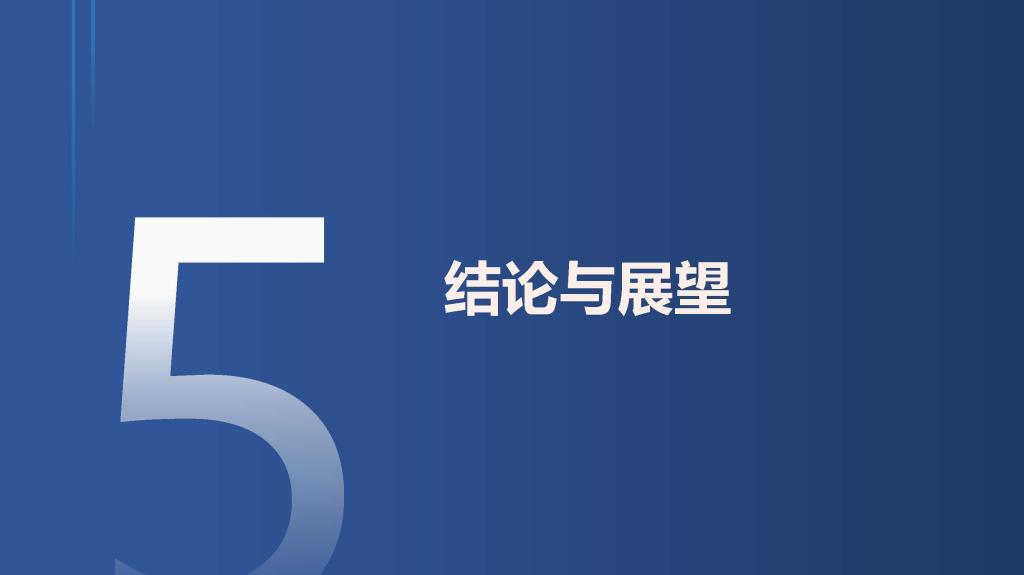
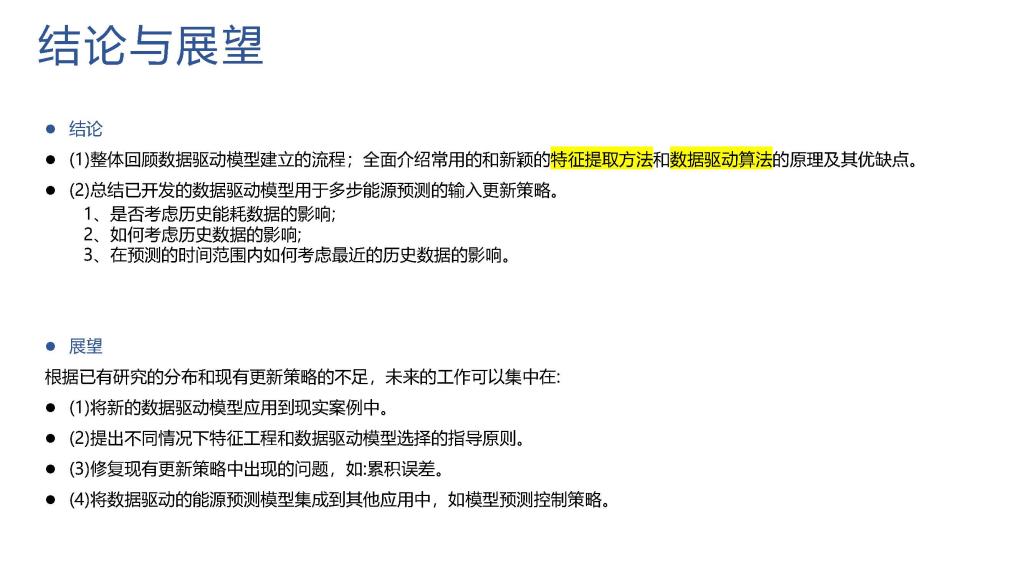